Study objective
We determine how peer review affects the quality of published data graphs and how the appointment of a graphics editor affects the quality of graphs in an academic medical journal.
Methods
We conducted an observational time-series analysis to quantify the qualities of data graphs in original manuscripts and published research articles in Annals of Emergency Medicine from 2006 to 2012. We retrospectively analyzed 3 distinct periods: before the use of a graphics editor, graph review after a manuscript’s acceptance, and graph review just before the first request for revision. Raters blinded to study year scored the quality of original and published graphs using an 85-item instrument. Editorial comments about graphs were classified into 4 major and 16 minor categories.
Results
We studied 60 published articles and their corresponding original submissions during each period (2006, 2009, and 2012). The number of graphs increased 31%, their median data density increased 50%, and quality (completeness [+42%], visual clarity [+64%], and special features [+66%]) increased from submission to publication in all 3 periods. Although geometric mean (0.69, 0.86, and 1.2 pieces of information/cm 2 ) and median data density (0.44, 0.70, and 1.2 pieces of information/cm 2 ) were higher in the graphics editor phases, mean data density, completeness, visual clarity, and other markers of quality did not improve or decreased with dedicated graphics editing. The majority of published graphs were bar or pie graphs (49%, 53%, and 60% in 2006, 2009, and 2012, respectively) with low data density in all 3 years.
Conclusion
Peer review unquestionably improved graph quality. However, data densities of most graphs barely exceeded that of printed text, and many graphs failed to present the majority of available data and did not convey those data clearly; there remains much room for improvement. The timing of graphics editor involvement appears to affect the effect of the graph review process.
Introduction
Background and Importance
Most research articles undergo a peer review process that can involve adding information, clarifying key points, checking for completeness, acknowledging limitations, or ensuring the article is presented in the preferred style of the journal. Although peer review has been in place for centuries, its efficacy is unclear. A 2002 meta-analysis failed to demonstrate any overarching benefit from peer review, but studies that examine specific components of the peer review process provide support for its utility. Wagner and Middleton demonstrated that technical editing, defined as any process designed to “improve accuracy or clarity or impose a predefined style, “increase[d] the readability and quality of articles”; however, the investigation failed to specify the exact editorial processes involved. A 2002 study in this journal concluded that the creation of methodology reviewers resulted in more focused editorial comments, and a second study showed that these comments resulted in modest improvements in article quality.
What is already known on this topic
Data graphs in medical science papers are common but vary greatly in their design. How they might be improved to convey more information has been little studied.
What question this study addressed
Was the quality of data graphs affected by increased resources at one journal compared with no special efforts when an editor reviewed graphics after acceptance of the paper and when the editor review occurred before the first request for revision?
What this study adds to our knowledge
The number of graphs, the amount of information conveyed, and measures of quality increased during implementation of the added measures. However, the majority remained simple pie or bar graphs with low data density despite the extra effort.
How this is relevant to clinical practice
Although the graphics editing yielded some improvement, there remained a great deal of room for more.
Despite graphs and figures being commonplace in the biomedical literature, studies demonstrate that published graphs are often suboptimal and fail to use the potential of the format. The peer review process has been shown to do little to correct these shortcomings.
Annals of Emergency Medicine has a comparatively sophisticated and well-studied peer review process relative to other journals. Papers are initially assessed by one of approximately 40 decision editors, who send approximately 50% of submissions for further review. Since 1997, each article sent for peer review has also been reviewed by a dedicated methodology or statistical editor. In addition, beginning in 2008, a senior “graphics” editor was assigned to review each paper. In 2008, this review took place at final acceptance and was the last step in the peer review process before publication. In 2011, the process had changed so that the graphics editor saw the paper before the first request for revision, and his comments were included along with those of the other peer reviewers. During both periods, the graphics editor had access to all comments made by other reviewers.
Goals of This Investigation
In this paper, we examine how the characteristics of graphs changed from submission to publication and from year to year, with a particular focus on whether the introduction of a graphics editor resulted in increased critique of graphs and improved graphic data presentation.
Materials and Methods
Study Design
We conducted an observational time-series analysis of the quality of data graphs in Annals from 2006 to 2012. We created a sample of 180 randomly selected published research articles composed of 60 manuscripts initially submitted for publication in 2006, 60 submitted in 2009, and 60 submitted in 2012. This represents approximately 60% of original science articles published in these years. These 3 years represent 3 distinct periods in the development of the journal’s editorial process: the period before formalized graph review (2006), graph review after the paper was accepted for publication (2009), and graph review at first “revise and resubmit” request (2012).
We separated each figure, including its caption, from the paper and selected a maximum of 5 graphs per paper. If a paper included more than 5 graphs, we randomly selected 5. In this report, we use “figure” to designate an object that is listed as a figure in the paper and “graph” to indicate a depiction of study data in a nontext, nontabular format. As such, figures such as flow diagrams of patient enrollment, theoretical models, or photographs are not graphs. Regardless of whether graphs were labeled as a single figure (eg, Figure 5 A , B , and C ) or were treated separately (eg, Figures 5, 6, and 7), we scored them as a single graph if they shared at least 1 identical axis and were thematically connected and as separate graphs if they were not.
Data Collection and Processing
We conducted the 3 parts of our data collection process independently: measuring the size of each graph, scoring the quality of each graph, and cataloguing the peer review comments about each graph. For the first part, an assistant, blinded to the purpose of the study and the year of each graph, calculated the area occupied by graph and caption by measuring the height and width in centimeters, using the measurement tool in Adobe Acrobat X Pro 10.1.16 (Adobe Systems, San Jose, CA).
For quality scoring, we trained 5 raters on a standardized set of graphs, and, after achieving adequate interrater reliability on a 20-graph sample (percentage agreement >90% on all 85 items), they scored the content and quality of study graphs presented in isolation, separate from their paper. Each rater scored a balanced number of submitted and published graphs from each of the years. Raters, who were never asked to score both the submitted and published versions of the same graph, were blinded to graph year, but not to whether each graph was from an unpublished or a published article because of differences in formatting that could not be blinded.
Raters scored each figure with a standardized form that captured both the graph’s type (eg, bar graph, scatter plot, parallel line plot) and 84 attributes, which included items used to calculate the numerator of the data density index (how many pieces of information are presented per square centimeter of graph), the dimensionality, and items about special features (eg, the depiction of clustering), graph completeness (eg, the presence of appropriate titles and labels), visual clarity (eg, the absence of excessive overlapping), and a gestalt assessment of whether the graph was self-explanatory ( Appendix E1 , available online at http://www.annemergmed.com ). After completing the data form, raters were allowed to refer to the whole paper to confirm that they had correctly understood any abbreviations and had interpreted the purpose of the graph correctly. The data collection form (Excel 2010; Microsoft Corp, Redmond, WA) contained explanations on how to score each item and pull-down menus showing scoring options ( Appendix E1 , available online at http://www.annemergmed.com ). Raters were encouraged to discuss with the authors any scoring decisions for which they were uncertain. During the comparison of published and unpublished versions of a graph, one author (C.D.) had the opportunity to review the scoring of both graphs side by side and correct (in consultation with D.L.S. or R.C. when necessary) any errors.
We created the portmanteau variables “completeness” and “clarity” to indicate whether all elements of these constructs were present and “special features” to indicate whether any such feature was present ( Appendices E1 and E2 , available online at http://www.annemergmed.com ). To be deemed “complete,” a graph had to include 10 essential features, listed and described in full in the scoring form ( Appendix E1 , available online at http://www.annemergmed.com ). A graph with visual “clarity” did not display any of 13 common “visual problems,” which are also listed and defined in the scoring form.
We calculated the data density index, the number of pieces of information per square centimeter, using a modified version of the formula proposed by Tufte. In brief, the data density index denominator is the area of the graph and its caption in the journal. Unpublished graphs were assigned the area of the published ones. The numerator was the number of pieces of discrete, unique information contained in a graph. A properly identified bar in a bar graph received 2 points, 1 for the height of the bar and 1 for the bar’s identifier. Each point in a scatter plot received 3 points, 1 for each axis value and 1 for the linkage of those 2 values. In all graphs, points were awarded for additional unique information such as the quantity depicted by an axis, labels, annotations, regression lines, and statistics (see Schriger et al and Tufte for details). For example, the data density index numerator for Figure 1 , a bar graph, was calculated as 18 bars plus 18 bar identifiers plus 1 for the labeled, distance-dependent x axis, or 37 points in total. The data density index for Figure 1 is 0.42 (37/86 cm 2 ).
Determining the numerator of data density index for Figure 2 , a series of univariate plots, is slightly more complicated. The calculation can be understood as follows: the approximately 540 visible points in the 6 rightmost sections (the “All years” plots are redundant and are not counted) are multiplied by 3 (1 for the axis value, 1 for the color, and 1 for the shape); to this we add 8 column identifiers, 32 median lines, 24 medians depicted by words or letters, 6 shape and 3 color identifiers, and an axis title, for a total of 1,694 pieces of information and a data density index of 7.5. Figures 3 and 4 have data density indexes of 1.07 (102/97 cm 2 ) and 0.76 (100/132 cm 2 ), respectively, and Figure 5 has a data density index of 0.95 (263/277).
We calculated dimensionality by determining the number of characteristics of each data point depicted in the graph. For example, a simple scatter plot would have a dimensionality of 2 because both the x and y axes provide a unique characteristic. However, if the points were color coded to define a particular attribute, the dimensionality would be 3, and, if a symbol or shape were used to convey another attribute, dimensionality would be 4 ( Appendix E3 , available online at http://www.annemergmed.com ). We considered bar, line, and pie charts to be “simple” graphs; box plots, histograms, and receiver operating characteristic curves to be “intermediate” graphs; and scatter plots, survival curves, parallel line plots, parallel coordinate plots, maps, and hybrid graphs to be “complex” graphs.
We analyzed the effect of editorial comments on the quality of graphic presentation by collecting all editorial feedback to authors during the peer review process from the journal’s database (Editorial Manager; Aries Systems, North Andover, MA). This correspondence included comments from the decision editor, the regular content reviewers, the methodology and statistics reviewer, and, depending on the study phase, the graphics editor. One author (C.D.) used the “find” function of Microsoft Word (version 2011; Microsoft Corp) to highlight graphic and statistical terms, such as “graph,” “figure,” “plot,” “bar,” “scatter,” “box,” “axes,” and “labels.” Using the highlighted terms as a guide, she carefully scanned all text for comments about the manuscript’s graphs. She then attributed each comment to 1 or more of the paper’s graphs, assigned the topic of the comment to one of 16 categories in 4 major classes ( Appendix E4 , available online at http://www.annemergmed.com ), and noted the role of the person who made the comment (regular reviewer, methodology/statistical reviewer, paper editor, or graphics editor). When a sentence in a review contained concepts that could be attributed to several categories, she parsed that sentence into multiple distinct comments. The fidelity of this process was confirmed by independent coding of a sample of reviews by a senior author (D.L.S.).
Primary Data Analysis
We sought to describe differences in a variety of measures of graph quality between original and published versions of articles and among study years. We programmed Stata (version 14; StataCorp, College Station, TX) to compare each element in the original and published versions of each graph in the context of this inventory of figure-related comments. The difference in medians and its CI were calculated with the cid command in Stata (version 14). For graphs that appeared in only the manuscript or published article, we examined whether comments were responsible for the elimination or addition of the graph. Through this process, we determined how often changes in graphic presentation occurred in response to editorial feedback and how often editorial feedback resulted in a change.
Materials and Methods
Study Design
We conducted an observational time-series analysis of the quality of data graphs in Annals from 2006 to 2012. We created a sample of 180 randomly selected published research articles composed of 60 manuscripts initially submitted for publication in 2006, 60 submitted in 2009, and 60 submitted in 2012. This represents approximately 60% of original science articles published in these years. These 3 years represent 3 distinct periods in the development of the journal’s editorial process: the period before formalized graph review (2006), graph review after the paper was accepted for publication (2009), and graph review at first “revise and resubmit” request (2012).
We separated each figure, including its caption, from the paper and selected a maximum of 5 graphs per paper. If a paper included more than 5 graphs, we randomly selected 5. In this report, we use “figure” to designate an object that is listed as a figure in the paper and “graph” to indicate a depiction of study data in a nontext, nontabular format. As such, figures such as flow diagrams of patient enrollment, theoretical models, or photographs are not graphs. Regardless of whether graphs were labeled as a single figure (eg, Figure 5 A , B , and C ) or were treated separately (eg, Figures 5, 6, and 7), we scored them as a single graph if they shared at least 1 identical axis and were thematically connected and as separate graphs if they were not.
Data Collection and Processing
We conducted the 3 parts of our data collection process independently: measuring the size of each graph, scoring the quality of each graph, and cataloguing the peer review comments about each graph. For the first part, an assistant, blinded to the purpose of the study and the year of each graph, calculated the area occupied by graph and caption by measuring the height and width in centimeters, using the measurement tool in Adobe Acrobat X Pro 10.1.16 (Adobe Systems, San Jose, CA).
For quality scoring, we trained 5 raters on a standardized set of graphs, and, after achieving adequate interrater reliability on a 20-graph sample (percentage agreement >90% on all 85 items), they scored the content and quality of study graphs presented in isolation, separate from their paper. Each rater scored a balanced number of submitted and published graphs from each of the years. Raters, who were never asked to score both the submitted and published versions of the same graph, were blinded to graph year, but not to whether each graph was from an unpublished or a published article because of differences in formatting that could not be blinded.
Raters scored each figure with a standardized form that captured both the graph’s type (eg, bar graph, scatter plot, parallel line plot) and 84 attributes, which included items used to calculate the numerator of the data density index (how many pieces of information are presented per square centimeter of graph), the dimensionality, and items about special features (eg, the depiction of clustering), graph completeness (eg, the presence of appropriate titles and labels), visual clarity (eg, the absence of excessive overlapping), and a gestalt assessment of whether the graph was self-explanatory ( Appendix E1 , available online at http://www.annemergmed.com ). After completing the data form, raters were allowed to refer to the whole paper to confirm that they had correctly understood any abbreviations and had interpreted the purpose of the graph correctly. The data collection form (Excel 2010; Microsoft Corp, Redmond, WA) contained explanations on how to score each item and pull-down menus showing scoring options ( Appendix E1 , available online at http://www.annemergmed.com ). Raters were encouraged to discuss with the authors any scoring decisions for which they were uncertain. During the comparison of published and unpublished versions of a graph, one author (C.D.) had the opportunity to review the scoring of both graphs side by side and correct (in consultation with D.L.S. or R.C. when necessary) any errors.
We created the portmanteau variables “completeness” and “clarity” to indicate whether all elements of these constructs were present and “special features” to indicate whether any such feature was present ( Appendices E1 and E2 , available online at http://www.annemergmed.com ). To be deemed “complete,” a graph had to include 10 essential features, listed and described in full in the scoring form ( Appendix E1 , available online at http://www.annemergmed.com ). A graph with visual “clarity” did not display any of 13 common “visual problems,” which are also listed and defined in the scoring form.
We calculated the data density index, the number of pieces of information per square centimeter, using a modified version of the formula proposed by Tufte. In brief, the data density index denominator is the area of the graph and its caption in the journal. Unpublished graphs were assigned the area of the published ones. The numerator was the number of pieces of discrete, unique information contained in a graph. A properly identified bar in a bar graph received 2 points, 1 for the height of the bar and 1 for the bar’s identifier. Each point in a scatter plot received 3 points, 1 for each axis value and 1 for the linkage of those 2 values. In all graphs, points were awarded for additional unique information such as the quantity depicted by an axis, labels, annotations, regression lines, and statistics (see Schriger et al and Tufte for details). For example, the data density index numerator for Figure 1 , a bar graph, was calculated as 18 bars plus 18 bar identifiers plus 1 for the labeled, distance-dependent x axis, or 37 points in total. The data density index for Figure 1 is 0.42 (37/86 cm 2 ).
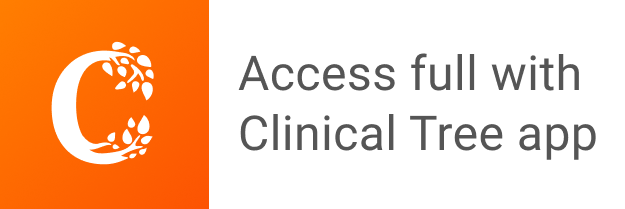