Term
Definition
Agreement studies
Studies assessing how well different methods of measuring the same underlying quantity agree with each other. Typical analyses are Bland-Altman methods (see Bland-Altman) including plotting differences versus the average of each pair, and calculating limits of agreement.
Alpha
Probability of rejecting the null hypothesis when the null is true in the population; probability of making a false-positive conclusion when conducting 1 or more tests; equal to the significance level or type I error
Alternative hypothesis
The hypothesis that one wishes to claim; the opposite of the null hypothesis
Analysis of variance (ANOVA)
Statistical method used to compare 2 or more groups on the mean of the outcome variable; equivalent to a 2-sample t-test if only 2 groups
Association
A relationship between 2 variables; assessed in many ways, depending on study design and type of variables
Beta
Probability of not rejecting the null hypothesis when it is false; probability of making a false-negative conclusion; type II error; 1 minus power
Bell-shaped curve
See normal distribution
Bland-Altman analysis
Bland-Altman method of assessing agreeing between 2 methods of measurement; for example, includes plotting the difference between methods on the outcome versus the average of each pair, and calculating limits of agreement. Limits of agreement are the mean difference +/− SD of the difference.
Bonferroni correction
Method used to maintain an overall type I error (or alpha), say at 0.05, by setting the significance criterion for a particular tests to alpha divided by number of tests.
Case control study
Study assessing the association between an exposure and outcome that is designed by first identifying subjects with and without the outcome and then assessing whether or not they had the exposure.
Categorical variable
A variable that is categorized, either ordinal (eg, Likert scale) or nominal (eg, gender, blood type).
Causal inference
Making conclusions that one variable causes the outcomes in another, as opposed to the variables simply being associated.
Central limit theorem
Key statistical theorem that states that the mean of repeated samples from a population will approximately equal the mean of the population, and the sampling distribution of the mean will have a normal distribution (bell-shaped curve).
Chi-square test
T-test used to compare 2 or more groups on a binary outcome variable.
Cohort study
A prospective study following a group or groups of patients over time.
Confidence interval (95%)
Interval defined as an estimate or estimated effect +/− 1.96 × standard errors; 95% of such intervals contains the true estimate or estimated effect.
Continuous variable
A variable, such as blood pressure or body mass index (BMI), that is measured on a continuum
Confounding
Means “distortion”. A variable that is associated with both the exposure and the outcome, and that should therefore be adjusted for when assessing association between exposure and outcome to prevent distortion of the effect of interest.
Correlation coefficient
A measure of the linear relationship between 2 variables.
Cox proportional hazards regression model
Regression method used to compare groups on time to an event, especially when all patients do not have the event during follow-up. Main assumption is that the hazard functions (function defined by percent having event over) are parallel for the groups being compared. A hazard ratio is estimated to compare groups on survival.
Cross-sectional study
Association between 2 variables at a fixed moment in time.
Data dredging
Practice of conducting many tests in a single dataset with the rather unfocused goal of searching for something that will be significant.
Dependent data
Data that is not independent, and often represents repeated measurements on the same subjects or units.
Dependent variable
The outcome variable in a regression model.
Diagnostic accuracy
Measures that assess how well a variable of interest can discriminate the truth, and measured by sensitivity, specificity, positive and negative predictive value, as well as the area under the receiver operating characteristic curve (for continuous or ordinal predictor).
Discrete variable
Variable that represents counts, such as number of infections for a subject.
Dispersion
Variability; the degree to which units differ from each other on some outcome.
Effect size
The true difference or effect divided by its standard deviation.
Estimation/estimate
A quantity measured in a sample that tries to capture the truth in the given population.
Evidence-based medicine
Medical practice which allows itself to be informed by rigorous research results.
Explanatory variable
The independent variable of interest, and which a researcher desires to associate with an outcome.
Histogram
Display of data that bins patients into equal-sized bars based on an outcome of interest and graphs the bars either horizontally or vertically.
Independent data
Data that are not correlated with each other—typically from different subjects.
Independent variable
Explanatory variable in a model, also sometimes called the predictor variable or exposure variable.
Inference
Making a decision about an association of treatment effect in a population of interest from data on a sample from that population
Interquartile range
Difference between the 3rd and 1st quartiles (75th and 25th percentiles) of a variable
Interval scaled variable
The quantitative variable for which a common distance between any 2 values has the same meaning.
Interaction
An interaction is present if the relationship between 2 variables (say exposure A and outcome B) is different for different levels of a third variable (interacting variables C).
Linear regression
Statistical model with continuous dependent variable
Kaplan-Meier curve
Method use to display survival or failure curves for time-to-event data and compare curves using log-rank or Wilcoxon tests
Kruskal-Wallis test
Generalization of the Wilcoxon Rank Sum test to more than 2 groups
Limits of agreement (95%)
Mean bias (or difference) between 2 methods of measurement ±1.96 × standard deviation of the difference. Shows where 95% of differences are expected to fall.
Logistic regression
Statistical model with binary dependent variable
Log-rank test
Test used to compare 2 or more survival curves (see Kaplan-Meier)
McNemar’s test
Test used to compare 2 paired or correlated proportions
Mean
Average of a set of data points; equal to the sum of the values divided by the sample size.
Median
Middle value among a set of data values sorted from smallest to largest. For an even number of observations, the median is the average of the 2 middle points.
Meta-analysis
Quantitative synthesis of results of more than 1 research study on the same topic
Mode
Most common value in a sample
Multiple comparisons procedures
Method used to control the type I error at a nominal level (usually 5%) when multiple comparisons are performed or multiple outcomes are assessed in the same study.
Multiple testing problem
The phenomenon that repeated testing increases the chance of false-positive findings.
Multivariable model
A statistical model that contains more than 1 independent or explanatory variable.
Multivariate analysis
A statistical model that contains more than 1 dependent or outcome variable, such as in a repeated measures model.
Negative predictive value
The probability of the true disease status being negative given that the test result or predictor is negative.
Nominal variable
A type of variable that is not continuous, discrete or ordinal—but only a name and without any inherent ordering, such as gender or blood type.
Non-normally distributed data
Data that does not follow a bell-shaped or Gaussian curve distribution.
Normally distributed data
Data that does have a bell-shaped or Gaussian distribution.
Normal distribution
A bell-shaped or Gaussian distribution defined by a mean and standard deviation.
Null hypothesis
The research hypothesis that researchers want to reject, and typically represents no association for the research question of interest.
Observational study
A study in which the independent variable or exposure variable is not under the control of the researcher.
Odds ratio
The ratio of the odds of an outcome in one group versus another. An odds ratio expresses the association between an exposure variable and an outcome variable, but does not imply causal inference between the 2 variables.
Ordinal variable
A data variable that consists of a limited number of ordered categories, such as ASA physical status or a Likert scale.
P-value
Probability value. A P-value gives the probability of observing a result as extreme or more extreme than the one observed in a research study if the hypothesis were in fact true.
Paired t-test
A statistical test used to compare to dependent samples on a continuous outcome. Oftentimes the dependent samples represent measurements on the same patients under 2 different scenarios, such as before and after an intervention.
Pearson correlation
A measure of the linear association between 2 continuous or ordinal variables. The square of the Pearson correlation (R squared) represents the proportion of variance in one variable explained by the other.
Population
The group of subjects or units that are the target of a research study; ie, the subjects, or units that one wishes to generalize to.
Positive predictive value
The probability that the true status is positive given that the test or predictor value is positive.
Power of a test
The probability of rejecting the null hypothesis for a given statistical method under a particular alternative hypothesis treatment effect.
Prediction model
A statistical model built particularly for the purpose of predicting individual patient values, and typically assessed for model fit (calibration) and how well the model can discriminate among the outcome values or explain the variance in the outcome.
Propensity score methods
Statistical methods used to control confounding by first modeling the probability of having the exposure as a function of potentially confounding variables, and then either matching exposed versus unexposed on that risk score (the propensity score) or weighting inversely on it when assessing the association between exposure and outcome.
Proportion
A measure of central location defined as the number of events divided by the number of patients who are subjects. Equivalent to the mean of a binary variable with values 0 and 1.
Quartiles
The data values corresponding to the 25th and 75th percentiles of a sample are referred to as the first and third quartiles.
Randomized trial
Research design in which the experimental units are randomly assigned to receive 1 of the 2 or more interventions being assessed, thus removing confounding or selection bias.
Range
The difference between the largest and smallest data value in a sample.
Rejecting the null
The decision to disavow the null hypothesis (typically of no association) based on a statistical test that gives a small P-value, or else based on a confidence interval for the association of interest that does not contain the null hypothesis value.
Relative risk
The ratio of 2 proportions, typically estimated in a randomized study when comparing 2 groups on the outcome of interest.
Research hypothesis
The scientific hypothesis upon which researchers build a research study.
Repeated measures ANOVA
A statistical method that includes repeated measurements on the same subjects, or units, and accounts for the likely correlation within those subjects or units when either comparing times or comparing groups over time.
R-squared
The statistic that estimates the proportion of the variance in the outcome variable,which is explained by 1 or more predictor variables in a linear regression, and is equal to the square of the Pearson correlation for simple linear regression.
Sample
The particular set of subjects, or units, that are measured in a research study; we make inference on the population of interest from the data in the sample.
Sample size calculation/ justification
The calculation giving either the number of required subjects, or the power to detect a difference in a research study. Components needed to calculate a sample size are the treatment effect of interest, estimated variability of the primary outcome, significance level, and power.
Scatterplot
A graph plotting 2 continuous variables—one on the vertical axis and the other on the horizontal axis—to visually assess their association.
Sensitivity
The probability that truly diseased patients will test positive.
Significance criterion
The P value criterion used to indicate whether the null hypothesis will be rejected or not.
Significance level
The type I error or probability of at least 1 false-positive finding in a research hypothesis or set of hypotheses.
Spearman correlation
A method to assess association between 2 quantitative variables using the rankings of the data values as opposed to the actual data values.
Specificity
The probability of truly non-diseased patients testing negative.
Standard deviation
Roughly speaking, the average deviation from the mean in a sample; the square root of the variance.
Standard error of the mean
The estimated variability of the mean of a group or of the difference between groups. For a single group, the standard error is equal to the standard deviation divided by the square root of N
Statistic
A quantitative measurement on a sample whose goal it is to estimate the same quantity in the population of interest.
Statistical errors
Alpha (type I error) and beta (type II error)
t-test
Statistical method used to compare to independent samples on a continuous outcome, or else to compare the single mean to a constant
Test statistic
The signal-to-noise ratio used in every statistical test, such as the difference in means divided by the standard error of the difference
Treatment effect
The difference between groups or the association of interest. Can be defined in many ways.
Variance
In a sample, the measurement of how much the individual units differ from each other on an outcome of interest.
Wilcoxon rank sum test
Mann-Whitney or Wilcoxon-Mann-Whitney test: compares groups on the ranks of the data. Equivalent to a t-test on the ranks. Does not directly compare medians.
Wilcoxon signed ranks test
A nonparametric test used to compare to dependent samples on a continuous or ordinal outcome variable using the ranks of the differences instead of the actual values.
38.2 Types of Data
Appreciation of the various types of data is an important step in understanding which statistical test would be most appropriate for a given situation [1]. The main types of data are interval or continuous, such as creatinine or blood pressure; ordinal or ranked data, such as American Society of Anesthesiologists (ASA) class (I, II, III, IV) or a Likert scale response (eg, satisfaction with care from low to high as 1,2,3,4,5); and categorical or nominal, such as male/female, alive/dead, or red/white/blue/green. Categorical data with two categories (male/female) is also called binary data. It is always a loss of information and therefore a less powerful analysis to make a continuous variable (say, age) into a binary variable (say, < 50, ≥ 50), although it is occasionally the best way to answer a specific research question. Counts such as number of children in a family or number of postoperative infections are called discrete measurements, and can often be analyzed using the same statistical methods as truly continuous data.
38.3 Descriptive Statistics
Summary statistics such as mean (standard deviation) for “normally distributed” data and median (25th%, 75th%) for non-normally distributed data are very useful ways to report study results. But any statistical analysis should also include plots of the data to visualize the relationship(s) that a statistical model is trying to express. It is a good rule of thumb that one should not report statistical results that cannot be visualized to some degree in a graphical display. A boxplot showing median, mean, quartiles, and range of data is an excellent way to display continuous data, and much better than simply plotting the mean and standard deviation (SD) (or standard error of the mean [SEM]) with a so-called “detonator” plot. In addition, if the data set is quite small, it is good to report a listing of the data points for each observation.
38.4 Normal Distribution
The frequency distribution of many variables is naturally a bell-shaped curve; symmetrically distributed with higher concentration of data near the central value, and less data moving away from the center (◘ Fig. 38.1). Examples are age, body mass index (BMI), blood pressure, height, weight, log-transformed length of stay (actual LOS is non-symmetrically shaped—skewed to the right). Gaussian distribution is another name for the “normal” distribution. Data following a true normal distribution has specific properties: it is defined by two parameters, a mean and standard deviation (SD); data are symmetrically distributed around a mean; ~ 68% of data points lie within 1 SD of the mean; mean = median.
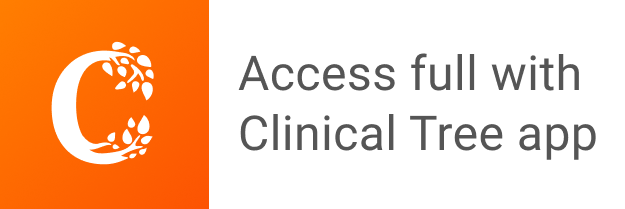