Alan Cook, MD, MS University of Texas at Tyler, Tyler, TX, USA Which of the following statements is the most correct? The area under the receiver operating characteristic curve originated as a measure of radio signal detection or discrimination of signal from noise. It also gained a great deal of traction in psychology then radiology and medical decision‐making. The AUROC can be interpreted as a measure of a model’s ability to discriminate patients with an outcome from those without. Here, the outcome was death from traumatic injury. The construct of the graph is the sensitivity (y‐axis) over 1‐the specificity (x‐axis) for each point computed by the model or at predetermined cut points. This can also be described as the true‐positive rate (y‐axis) over the false‐positive rate (x‐axis). The AUROC for the ISS indicates the ISS can discriminate survivors from fatalities 83.1% of the time. Whereas the TMPM can make such discrimination 87.5% of the time. As such, the TMPM compares favorably over the ISS. In this analysis, the closer the curve is to the point [0, 1] (left upper corner) the greater the area under the curve indicating better discrimination capability. Thus, the diagonal line represents an AUROC of 0.5 where the model predicts no better than a coin toss. Answer: A Hanley J, McNeil B. The meaning and use of the area under a receiver operating characteristic (ROC) curve. Radiology. 1982; 143:29–36. Select the best answer from the following: Figure 48.1 AUROC comparison of ISS and TMPM. The AUROCs for ISS and TMPM are 0.831 and 0.875, respectively. This study should be considered cohort study as the patients are selected for the study, and the outcome is compared according to smoking status (the exposure). If the study sought to describe the proportion of smokers among elderly trauma patients, it could be considered a prevalence study or a cross‐sectional study. Prevalence is a measure of the number of subjects in a population who have a condition at the time of the study and can be thought of as a “snapshot‐in‐time,” much like a survey or poll. While a randomized study is considered the epitome of study designs, it is not feasible in all studies. Here, we cannot ethically randomize elderly patients to smoke or not, and the physiological phenomenon of interest is deterioration of pulmonary function, which takes a significant amount of time to accumulate before an effect would be manifest clinically (choice C). The long follow‐up time would be prohibitively resource intensive. Although no intervention is being studied, the study would require consent from the participant as the protocol will entail medical testing and include the analysis of other clinical data. Since no new intervention is involved, the study may qualify for expedited review by the Institutional Review Board (choice D). If the study began with a group of teenagers and followed their pulmonary function over their lifetime at 5‐year intervals, the study would be a longitudinal study (choice E). The quintessential cohort study is the Framingham Heart Study. Answer: C Dawber TR, Meadors GF, Moore Jr FE. Epidemiological approaches to heart disease: the Framingham study. American Journal of Public Health and the Nations Health. 1951; 41(3):279–86. Confounding results when a third variable is responsible for the effect of the exposure (X) on the outcome (Y). The third variable, the confounder, is related to the exposure and the outcome. A classic example is the effect of alcohol consumption on lung cancer. The effect may be accounted for by the confounder of smoking. Oftentimes, people smoke while drinking or drink in places where smoking is present, like bars. Here, smoking is not equally distributed among the alcohol drinkers. Confounding is shown schematically in the following diagram: The effects of confounding can be mitigated or adjusted for by including the confounding variable in a multivariable model, for example. The result of including smoking status in the analysis would likely mitigate or completely negate any observed association between alcohol consumption and lung cancer. Answer: E Williamson EJ, Aitken Z, Lawrie J, Dharmage SC, Burgess JA, Forbes AB. Introduction to causal diagrams for confounder selection. Respirology. 2014; 19(3):303–11. Randomization is the process in a randomized control trial where the participants are allocated to intervention or control groups through a formal randomization process. A trial that includes a randomization step as part of the protocol is, by definition, a prospective study that requires an intervention, follow‐up time, and staff to collect data. These characteristics of randomized trials tend to make them expensive compared to other study designs. A key strength of randomization is the removal of selection bias in the allocation of subjects to intervention and control groups. The removal of selection bias and the prospective nature of the study provide strong justification to infer causality between the intervention and outcome of a study. The randomization process tends to produce relatively balanced groups of participants in terms of characteristics important to the study. However, since p‐values are influenced by the effect size of the intervention and the number of observations in the analysis, the mere act of randomization does not assure statistical significance. Finally, not all studies lend themselves to a random allocation of subjects to the treatment or control groups. This is the case when treatments have become established as the standard of care despite the lack of prospective randomized trials. Answer: D Greenland S. Randomization, statistics, and causal inference. Epidemiology. 1990; 1(6):421‐9. The proper test for this analysis is: In a previous question, we discussed the phenomenon of confounding. One method of adjusting for one or more confounders in the analysis phase of a study is to control for them in a multivariable model. Here, the outcome of interest is binary, VAP (yes/no). Therefore, the paired Student’s t‐test, which compares the means of a variable for a group of individuals measured before and after an intervention, like subjects’ weight before and after a diet change is not appropriate (choice A). The Mann‐Whitney U test is another name for the Wilcoxon Rank Sum test where one can compare the means of a variable between two independent groups of subjects when the distribution of the variable is not normally distributed. Additionally, the Mann‐Whitney U test is a bivariate test and cannot accommodate the nine variables necessary to the study (choice E). The Cox proportional hazard ratio is a multivariable model that incorporates a time‐to‐event component, e.g. the number of ICU days until discharge or death. The study in question is simply interested in whether or not VAP develops, not how long it takes to develop (choice D). The multivariable linear regression would be an appropriate multivariable model if the outcome of interest is continuous and linear, like hospital length of stay. The multivariable logistic regression is the model of choice for the analysis at hand (choice C). The logistic regression model is used to describe the relationship between a binary outcome variable, VAP, and a set of independent predictor variables whether they are continuous, categorical, or binary. The results are reported as odds ratios with 95% CIs and p‐values for the predictors. Answer: B Peng C‐YJ, So T‐SH. Logistic regression analysis and reporting: a primer. Understanding Statistics: Statistical Issues in Psychology, Education, and theSocial Sciences. 2002; 1(1):31–70. Describe the variable activations in terms of data type. Numerical data can take several forms. The type of numerical data in a variable can determine the appropriate tests of significance and regression model to choose. The simplest type is binary or dichotomous. Binary data contain two mutually exclusive values like 1 or 0 for alive or dead (choice A). Nominal data represents categories of a phenomenon like blood type, for example 1 = A+, 2 = A−, …, 6 = O−. There is no quantitative difference between the categories. A + ≠ 2 × A−. Moreover, the blood types aren’t ordered. The numeric values are contiguous as a matter of convenience (choice B). Ordinal data can be placed in meaningful order, e.g. the order of finishers in a race (1st place, 2nd place, and so on). However, there is no information about how far apart the runners finished (0.01 seconds between 1st and 2nd place, 0.07 seconds between 2nd and 3rd place) (choice C). If the variable was named “Total Time” and contained each racer’s course time in milliseconds, the variable would be considered continuous just as the variable “Activations.” Note that continuous data are presented as discrete values rounded to a convenient decimal place (choice D). Most biometric data belong on the ratio scale. The ratio scale is like the continuous numeric scale with the limitation that it includes zero but does not include negative numbers (choice E). Answer: C Barkan H. Statistics in clinical research: important considerations. Annals of Cardiac Anaesthesia. 2015; 18(1):74. The 2 × 2 contingency table is a fundamental construct in biostatistics. It can represent a test result (positive or negative) and the disease state (present or absent), a risk factor and the disease, etc. All of the following can be calculated from the 2 × 2 table as follows:
48
Statistics
Mean (SD)
Minimum, maximum
Median (IQR)
Activations
199.7 (110.5)
60, 408
160 (200)
Resident cases
22.1 (6.8)
12, 32
23.2 (11.6)
Disease
No disease
Exposure
a
B
No exposure
c
D
or the ratio of risk of an outcome in the exposed to that in the unexposed. Odds ratio (OR) is the ratio of odds of an outcome in the exposed to the odds in the unexposed.
Sensitivity and specificity are common terms in scientific literature. Sensitivity is the proportion of true positive cases (a) among all who develop the disease (a + c). While specificity is the proportion of true negative cases (d) among all who do not have the disease (d + b).

Full access? Get Clinical Tree
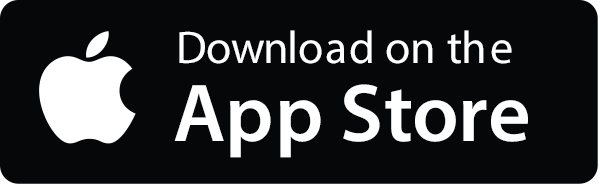
