Chapter 11 Joshua R. Lakin and Eric W. Widera Prognostication involves two separate tasks. The first requires clinicians to estimate prognosis, which is the probability of an individual developing a particular outcome over a specific period of time. The second is to communicate this information to the patient and/or family. These two tasks are fundamental skills for hospitalists to master as nearly every diagnostic or therapeutic medical decision requires some knowledge of a patients’ prognosis. Furthermore, prognosis adds a crucial timeframe required for determining realistic and achievable goals of care for patients, families, and health-care providers. Prognosis impacts many decisions in the normal process of admission to discharge for patients who are hospitalized. Upon admission, prognosis drives triage to higher or lower levels of care based on the likelihood of decompensation. During the hospital stay, knowledge about prognosis influences most diagnostic and treatment decisions. For instance, when older adults were asked about their end-of-life preferences, 44% desired CPR [1]. After learning the probability of survival, the number desiring CPR dropped by half to 22%. Prognostic information can also aid in targeting interventions to those likely to live long enough to benefit, as seen with decisions around preventative cancer screening and tight glucose control in diabetes or artificial nutrition for a patient with advanced cancer. Upon discharge, prognostication affects location of discharge and which interventions are continued. For example, decisions on whether someone needs a short-term skilled nursing facility stay or long-term care are based on the likelihood that an individual will improve with physical and occupational therapy. Estimating prognosis is also is explicitly required for eligibility. Another reason to develop skill in prognostication is that patients and their families desire this information, even if the prognostic information is uncertain [2]. The reasons surrogates cite for this desire include preparing for loss, allowing families to hope for the best and prepare for the worst, fostering trust in their doctors, and aiding in decision-making [2]. However, despite this need, physicians remain distressed by prognosticating and studies have demonstrated that both patients and physicians tend to overestimate prognosis. Part of this is due to lack of training. In a large survey in 1998, 7% and 6% of internists reported inadequate training in diagnosis and therapy, respectively, but 57% reported inadequate training in prognostication despite needing this information frequently [3]. The estimation of prognosis can be conceptually broken down into three main methods: (1) clinician prediction of survival (CPS), (2) population-based averages and prognostic indices, and (3) a combined approach using all of these methods. Clinicians use a variety of inputs into individual predictions of survival, including pathological and clinical findings, diagnosis, comorbidities, past and ongoing therapy, and psychosocial factors. The combined weight and analysis of each of these factors is what eventually drives a clinician’s estimate but, unfortunately, this process remains poorly understood. Nonetheless, while the process is not clear, the accuracy of clinician judgment has been studied. A systematic review of physicians’ estimates of survival in cancer patients demonstrated that while clinicians can separate those at high risk of death from those at low risk of death, their prognostic estimates are poorly calibrated as there is a systematic bias toward optimism [4]. Further studies have shown that CPS is confounded by the doctor–patient relationship and by experience level. For instance, clinicians provide more optimistic prognoses to patients they have known for a long time, although this may be less of an issue for hospitalists who typically are meeting patients for the first time. The factors involved in population-based averages and prognostic indices vary extensively, from patient- or population-based characteristics such as age, gender, and race displayed in a life table to specific biomarkers analyzed in regression models and then incorporated into an online tool. As displayed in Table 11.1, there are several categories of tools, and we have provided some specific examples within each category. Table 11.1 Categories of Population Averages and Prognostic Tools The use of life tables require knowledge of only a few key characteristics but, as a result, give a broad population-based estimate of median survival. This presents problems in populations such as the elderly where there is significant variation in life expectancy among patients of similar ages. Another method to determine population-based averages is to use published studies whose participants closely mirror the patient’s clinical details and then apply the results from these studies to generate a prognosis. This method provides a more individualized estimate than does a life table but requires an accurate match between a specific patient and a study population. Because studies often exclude individuals who are hospitalized or who have multiple comorbidities in order to strengthen association statistics, using these studies for ill hospitalized patients is often difficult. The last method to refine population-based estimates is to use well-validated prognostic indices. Prognostic indices are tools that utilize systematically selected characteristics from a particular population, such as functional status or lab results, to calculate a prognostic estimate. The use of prognostic indices requires knowledge of the accuracy, validity, and generalizability of a specific index. For instance, if a prognostic index was developed in a community-based setting, it will likely overestimate prognosis in hospitalized adults. Each of the aforementioned methods has its own benefits and drawbacks. Clinician estimates of survival have consistently shown to overestimate survival. Population-based averages and prognostic indices may avoid this bias, but the prognostic estimates derived from them may not be generalizable or individualized to the individual patient under the care of the hospitalist. Given this, we recommend using either population-based data from recent research studies or a prognostic index that closely approximates the patient’s condition to “recalibrate” an educated, self-aware clinical judgment. We will now detail a few of these tools that will be most useful to hospitalists. Many patients for whom hospitalists care have multiple chronic conditions, functional impairment, advanced illness, and/or cognitive impairment. Focusing on one specific disease when estimating prognosis fails to take in the interactions of all these factors. In addition, older adults are typically underrepresented in the development of most disease-specific prognostic indices and in clinical trials. Non-disease-specific prognostic indices, which have been created in multiple different settings including hospitals, nursing homes, home, and hospice, are typically most useful for these patients. A repository of published non-disease-specific prognostic indices for older adults can be found at www.ePrognosis.org. One prognostic index that is of particular importance for hospitalists and is included in ePrognosis is the Walter index. This index was developed in tertiary care hospitals and validated in community hospitals, making it useful to estimate all the causes for 1-year mortality [6]. For the elderly and those with advanced illness, one of the strongest markers for mortality is the performance or functional status of a patient. A number of tools focusing on functional status have been developed. Two of the most commonly used tools in palliative care and hospice include the Karnofsky Performance Status Scale (KPS) and the Palliative Performance Scale (PPS). The KPS (Table 11.2) was devised as part of one of the first studies to show an association between performance status and survival, and it has since been validated, albeit only in cancer populations. The obvious challenge in using the KPS, as well as the other performance status scales, is that patients are often admitted to the hospital with an acute decline that may or may not be reflective of their baseline functional status. Table 11.2 Karnofsky Performance Status Scale Building upon this tool is the PPS (Table 11.3), and it has been validated in a broader population but is also subject to similar biases as the KPS. Importantly, the PPS has been studied more recently in both cancer and noncancer hospitalized patients receiving palliative care and used to build survival estimates by PPS level [7]. The PPS and survival periods resulting from this data are also displayed in Table 11.3. The validation in hospitalized patients makes the PPS a useful performance status tool in informing prognosis in hospitalized patients. However, it is important to remember that the PPS is biased by the fact that prognostic data was collected as part of care by palliative care teams, so selection of patients is not a random set of those admitted to the hospital. Table 11.3 Palliative Performance Scale (PPS)a Tool [7] and Survival Statistics [8] a Instruction for use of PPS (see also definition of terms) b Survival times were taken from the initial PPS done during the first assessment at home or in the hospital by a regional palliative care team. 1. PPS scores are determined by reading horizontally at each level to find a “best fit” for the patient which is then assigned as the PPS% score. 2. Begin at the left column and read downward until the appropriate ambulation level is reached, and then read across to the next column and downward again until the activity/evidence of disease is located. These steps are repeated until all five columns are covered before assigning the actual PPS for that patient. In this way, “leftward” columns (columns to the left of any specific column) are “stronger” determinants and generally take precedence over others. The categories below represent categories of commonly seen diseases and syndromes in hospital medicine. Each subject examines the prognostic considerations and highlights available tools if any. There is significant heterogeneity between disease courses in different types of cancer, even with narrowed definitions such as “within metastatic solid tumors” [9]. For instance, metastatic breast and prostate cancer are associated with much longer survival times as compared to metastatic lung cancer. With tissue diagnosis and staging established with the aid of consultants such as oncology, surgery, gastroenterology, and interventional radiology, there are several means of estimating an initial disease-based prognosis. One method is through the use of published studies related to a particular diagnosis, specifically from treatment trials. While this can often be challenging for physicians, there are a large number of possibly relevant treatment trials that can provide guidance on prognosis for treatment versus supportive care at any particular point in an oncologic disease course. Of note though, most of these trials do not include patients who are hospitalized or who have poor functional status, multimorbidity, or organ dysfunction. Using one of the performance status-based tools as described in the previous section is a foundation for hospitalists in cancer prognostication as there is often a dearth of other evidence from clinical trials in hospitalized, ill advanced cancer patients. Furthermore, in this population, performance status is consistently demonstrated to show an association with survival, although length of survival may depend on the underlying illness. For example, for patients with metastatic cancers with relatively good prognosis and treatment options, such as prostate or breast cancer, a KPS less than 60 correlates with a median survival of less than 6 months [10]. This is in contrast to cancers with poor prognosis, such as pancreatic or biliary cancers, which result in a median survival of less than 6 months for those with a much higher KPS score (less than 90) [10]. In addition to functional status, a range of symptoms and laboratory values have been demonstrated to correlate with survival. For instance, out of a myriad of symptoms studied, anorexia, weight loss, xerostomia, dysphagia, dyspnea, confusion, and cognitive decline have shown clear association with prognosis [4, 9]. The Palliative Prognostic Score combines a group of patient level characteristics (CPS, KPS score, symptoms, white blood cell count, lymphocyte count) into one prognostic score, recently updated and validated to include delirium as well [11]. Like other diseases that involve chronic, progressive organ dysfunction, short-term survival is challenging to prognosticate for people with heart failure. It is clear though that the need for hospitalization in this population is associated with high mortality rates despite recent advances in heart failure management. In a study of 2.5 million Medicare beneficiaries hospitalized with heart failure, there was a reduction in in-hospital mortality from 5% to 4% between 2001 and 2005, although mortality at 30 days, 180 days, and 1 year remained unchanged at 11%, 26%, and 37%, respectively [12]. The prognosis is worse after each subsequent hospitalization for heart failure. The median survival in one study of older patients admitted for heart failure declined from 2.4 years in those with one hospitalization to 0.6 years for those with four hospitalizations [13]. The prognosis only worsens for the oldest old who have a median survival of 1 year after just a single hospitalization and a median survival of 6 months after two hospitalizations [13]. In addition to hospitalizations, there are several other markers of poor prognosis. These include patient demographic factors, heart failure severity, comorbid diseases, physical examination findings, and laboratory values including cachexia, hyponatremia, anemia, and NYHA Class. Two important prognostic tools to help clinicians estimate prognosis in heart failure include the EFFECT model and the Seattle Heart Failure Model. The EFFECT model was developed from a cohort of 2624 patients who presented with heart failure between 1999 and 2001. The factors used to stratify risk of death include age, comorbidities, and physiologic variables (respiratory rate, systolic pressure) and laboratory findings (blood urea nitrogen, serum sodium concentration, and hemoglobin) at the time of hospital presentation. Thirty-day-mortality risks range from 0.4% in the lowest risk group to 59% in the highest risk group. An online calculator for the EFFECT model can be found at www.ccort.ca/CHFriskmodel.aspx. The Seattle Heart Failure Model is a freely available although lengthy online calculator (http://depts.washington.edu/shfm/) that was developed and validated among outpatients participating in clinical trials, observational studies, and clinical registries. Caution is warranted though when generalizing the results to hospitalized patients or those with other major comorbidities such as renal failure, dementia, or cancer. Individuals with advanced dementia typically have a prolonged period of severe functional disability, and during this time they are at risk of acute events such as pneumonias and urinary tract infections that are markers of very poor short-term survival. For instance, the 6-month mortality rates exceeded 50% in one study of individuals with advanced dementia who are admitted to the hospital with either pneumonia or a hip fracture. Hospice eligibility criteria for dementia require that individuals need to meet or exceed stage 7a on the Functional Assessment Stage (FAST) scale (Table 11.4) and must have at least one complication from their dementia (aspiration, upper urinary tract infection, sepsis, multiple stage 3–4 ulcers, persistent fever, weight loss >10% within 6 months). However, studies have shown that these criteria fail to accurately predict 6-month survival in those with advanced disease. The Advanced Dementia Prognostic Tool (ADEPT), which can be found online at ePrognosis.org, can help identify nursing home residents with advanced dementia at high risk of death within 6 months. Compared to the hospice eligibility criteria, the ADEPT had greater predictive value of 6-month prognosis. Table 11.4 Summary of Functional Assessment Staging (FAST) Two of the most commonly used prognostic models used in chronic liver disease are the Child–Turcotte–Pugh (CTP) score and the Model for End-Stage Liver Disease (MELD). The CTP uses five variables (serum bilirubin, serum albumin, prothrombin time, ascites, and encephalopathy) to categorize patients into one of three classes (A, B, or C). The largest drawback to the CTP is the subjectivity in grading ascites and encephalopathy, and its limited ability to stage the severity along a broad continuum seen in liver disease as individuals are only placed into three categories. MELD is a prospectively developed and validated continuous scoring system that calculates the severity of chronic liver disease across a broader spectrum than the CTP. It uses a patient’s laboratory values for serum bilirubin, serum creatinine, and the international normalized ratio (INR) to predict survival. Mortality for patients awaiting liver transplantation is noted in Table 11.5. Of note, there are some conditions, such as hepatocellular carcinoma (HCC) and hepatopulmonary syndrome, in which the calculated MELD score overestimates survival. An online MELD calculator can be found at http://www.mayoclinic.org/meld/mayomodel6.html. Table 11.5 3-Month Mortality for Patients Awaiting Transplantation Based on MELD Score [14]
Prognostication: Estimating and Communicating Prognosis for Hospitalized Patients
11.1 WHAT IS PROGNOSTICATION?
11.2 WHY IS PROGNOSTICATION IMPORTANT?
11.3 OVERVIEW OF METHODS TO ESTIMATE PROGNOSIS
11.3.1 Clinician Judgment and Experience
11.3.2 Population-Based Averages and Prognostic Indices
Tool
Examples
Life tables
Centers for Disease Control—National Vital Statistics Reports
Generalizing data from studies and trials
Overall survival in previously treated stage IIIB or IV non-small cell lung cancer [5]
Prognostic indices
ePrognosis.org, Palliative Performance Scale, Acute Physiology and Chronic Health Evaluation (APACHE)
11.3.3 Combined Approach
11.4 TOOLS FOR ESTIMATING NON-DISEASE-SPECIFIC PROGNOSIS
Able to carry on normal activity and to work; no special care needed
100
Normal, no complaints; no evidence of disease
90
Able to carry on normal activity; minor signs or symptoms of disease
80
Normal activity with effort; some signs or symptoms of disease
Unable to work; able to live at home and care for most personal needs; varying amount of assistance needed
70
Cares for self; unable to carry on normal activity or to do active work
60
Requires occasional assistance but is able to care for most of his personal needs
50
Requires considerable assistance and frequent medical care
Unable to care for self; requires equivalent of institutional or hospital care; disease may be progressing rapidly
40
Disabled; requires special care and assistance
30
Severely disabled; hospital admission is indicated although death not imminent
20
Very sick; hospital admission necessary; active supportive treatment necessary
10
Moribund; fatal processes progressing rapidly
0
Dead
PPS Level
Ambulation
Activity and Evidence of Disease
Self-Care
Total Care
Conscious Level
Mean Survival Time in Days (95% CI)b
Median Survival Time in Days (95% CI)b
Range of Survival in Daysb
100%
Full
Normal activity and work; no evidence of disease
Full
Normal
Full
n/a
n/a
n/a
90%
Full
Normal activity and work; some evidence of disease
Full
Normal
Full
n/a
n/a
n/a
80%
Full
Normal activity with effort; some evidence of disease
Full
Normal
Full
151 (92, 210)
71 (0, 196)
33–424
70%
Reduced
Unable to do normal job/work; significant disease
Full
Normal or reduced
Full
168 (133, 203)
110 (77, 143)
3–607
60%
Reduced
Unable to do hobby/house work; significant disease
Occasional assistance necessary
Normal or reduced
Full or confusion
104 (61, 147)
50 (33, 67)
7–624
50%
Mainly sit/lie
Unable to do any activity; extensive disease
Considerable assistance required
Normal or reduced
Full or confusion
58 (43, 72)
35 (29, 41)
2–320
40%
Mainly in bed
Unable to do any activity; extensive disease
Mainly assistance
Normal or reduced
Full or drowsy ± confusion
65 (36, 95)
31 (15, 47)
1–493
30%
Totally bed bound
Unable to do any activity; extensive disease
Total care
Normal or reduced
Full or drowsy ± confusion
32 (23, 41)
12 (9, 15)
1–249
20%
Totally bed bound
Unable to do any activity; extensive disease
Total care
Minimal to sips
Full or drowsy ± confusion
14 (8, 21)
6 (4, 8)
<1–123
10%
Totally bed bound
Unable to do any activity; extensive disease
Total care
Mouth care only
Full or drowsy ± confusion
5 (1, 9)
2 (1, 3)
<1–22
0%
Death
—
—
—
—
—
—
—
11.5 TOOLS FOR ESTIMATING DISEASE-SPECIFIC PROGNOSIS
11.5.1 Cancer
11.5.2 Heart Failure
11.5.3 Advanced Dementia and Cognitive Decline
Stage 1
No subjective or objective impairments in cognition
Stage 2
Mainly subject complaints of forgetting names and misplacing objects
Stage 3
Objective evidence of memory impairment; impairment beginning to affect work performance
Stage 4
Moderate cognitive decline with impairments in instrumental activities of daily living
Stage 5
Difficulty in naming current aspects of their lives with some disorientation
Stage 6 (a–e)
Difficulty dressing, bathing, toileting without assistance. Experiences urinary and fecal incontinence in stage 6d and 6e
Stage 7 (a–f)
Speech declines from less than six intelligible words per day (7a) to one or less (7b). Progressive loss of ability to ambulate (7c), sit up (7d), smile (7e), and hold head up (7f)
11.5.4 Chronic Liver Disease

Full access? Get Clinical Tree
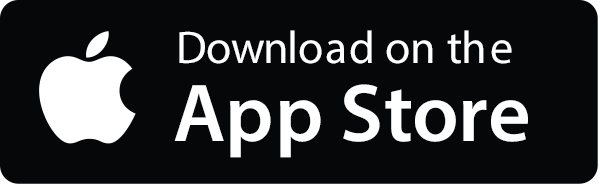
