KEY POINTS
Quality is defined as “the degree to which health services for individuals and populations increase the likelihood of desired health outcomes and are consistent with current professional knowledge.”
The ability to measure quality is an essential component to improve quality of care.
Quality of care has multiple domains and no single metric can appropriately define quality.
Quality indicators should represent metrics that have face validity and are actionable by patients, clinicians, and managers.
Methodological rigor is necessary to avoid spurious interpretations and provide proper interpretation of quality metrics.
Public reporting quality metrics can have unintended consequences to the health care system.
Quality metrics can be divided into outcome metrics, process metrics, and structural metrics.
Quality metrics that are based on outcomes are widely used to compare health care systems, but are not necessarily sensitive or specific to identify outliers and may lead to biased conclusions.
When rigorously and objectively defined, quality metrics that are based on processes of care can be more informative on specific aspects of quality.
Many structural aspects of ICUs are associated with quality, but it is possible for ICUs that do not have these attributes to still perform with high quality.
DEFINING QUALITY
The definition of quality depends on the field being evaluated. For example, although they each provide food and housing, the definitions for high-quality hotels, prisons, and hospitals will be considerably different. The International Organization for Standardization defines quality broadly as “the totality of features and characteristics of a product or service that bears on its ability to satisfy stated or implied needs” (ISO 8402–1986 standard). In health care, quality has been abstractly defined as “the degree to which health services for individuals and populations increase the likelihood of desired health outcomes and are consistent with current professional knowledge.”1 Although a bit vague, this definition emphasizes two challenging aspects of measuring the quality of health care: (1) the need to improve outcomes and (2) the importance of evidence. Throughout this chapter, we will focus on these two concepts to discuss measuring quality through evidence-based processes of care that should ultimately lead to improved outcomes.
WHY DO WE MEASURE QUALITY?
“Count what is countable, measure what is measurable, and what is not measurable, make measurable” is frequently attributed to Galileo.2 The ability to manage outcomes or processes of care is fundamentally tied to being able to measure them. Finding clinically relevant, measurable, and actionable outcomes and processes in health care is necessary to provide clinicians with the ability to improve their systems. This is not to say that all important determinants of quality can be measured or that those that cannot be measured should be ignored. Deming, the grandfather of quality metrics, once stated that “running a company on visible figures alone” is one of the seven deadly sins of management.3 However, to demonstrate improvement or to detect deviations from the expectations, metrics are needed.
Governments, regulators, clinicians, insurance companies, and patients may need different quality measures or the same measures presented in different ways. Unfortunately, quality indicators are often selected based on convenience, feasibility, or politics rather than validity. In this chapter, we will try to define what the ideal characteristics of quality metrics should be and apply these principles to the current metrics proposed for intensive care medicine.
INDICATORS
An ideal indicator would have the following key characteristics: (1) specific and sensitive to the process or outcome being measured; (2) measurable based on detailed definitions so that indicators are comparable; (3) actionable so that the they can lead to specific interventions to improve quality; (4) relevant to clinical practice and based on available scientific evidence; and (5) timely so that the information is reported to the interested parties in a way that can motivate change (see Table 2-1).4
The Ideal Quality Indicator (SMART)
Characteristic | Definition |
---|---|
Sensitive and specific | The ability of the indicator to detect true positives and true negatives. |
Measurable | Validity and reliability. An indicator should measure what it is intended to measure (validity) and should be reproducible (reliability). Clear instructions for inclusion and exclusion criteria, as well as objective parameters are essential. |
Actionable | The indicator can be modified by actions taken from the stakeholders. |
Relevant | The indicator is based on scientific evidence. |
Timely | The indicator is available in a timely manner to allow for interpretation and corrective actions. |
Specific and Sensitive: Indicators share the same properties as diagnostic tests: sensitivity and specificity. Sensitivity is the ability of a test to identify true positives. For example, a sensitive indicator for ventilator-associated pneumonia (VAP) should identify patients who actually have VAP. An indicator that measures a process of care, such as compliance with daily interruption of sedation, should identify patients who have received that treatment. On the other hand, specificity should also be high; therefore, patients who do not have VAP should not be identified by the measure and patients who did not receive an interruption of sedation should be properly coded. Perfectly accurate quality measures do not exist; however, as long as a test is measurable, then comparisons of different units and of the same unit over time become feasible.
Measurable: The parameter must be measurable in a reliable and valid way by different observers and over time. Subjective definitions such as “if patient is in shock” are too broad to allow for adequate measurements; a better way is to have clear definitions, based on observable parameters, such as “if systolic blood pressure is below 90 mmHg for at least 1 hour.” For example, the proportion of ventilated patients receiving a spontaneous breathing trial (SBT) is not adequate to control the process, as many patients may not undergo an SBT because they have contraindications. Therefore, metrics should clearly specify the population that is eligible for measurement.
Reliability implies that repeated measurements will provide the same results. An indicator that gives different results for the same population should not be used to address quality. An example of a measurement that is reliable is the measurement of time to reaching target cooling temperature after cardiac arrest. The time zero (hospital arrival) and the time of goal temperature can be clearly defined and abstracted from charts. On the other hand, VAP rates are less reliable. A study comparing the identification of VAPs by two experienced providers, using the CDC VAP definition, observed a twofold variation in the total numbers of VAP. Based on these data, twofold increases or decreases in VAP rates could be simply due to variation in interpretations of the CDC VAP definition.5
Actionable: An indicator is only helpful if the users and managers of the outcomes and processes are able to take actions based on the information gained. For example, although long-term health-related quality of life in ICU survivors is an important outcome, it is a poorly actionable quality measure as the determinants of this outcome are poorly understood and may not be primarily determined by practices in the ICU. On the other hand, an indicator that provides ICU managers with compliance rates for SBTs may be immediately actionable if unacceptable. In some circumstances, indicators may be selected due to a misinterpretation of research and may not be achievable. For example, a single-center randomized controlled trial observed a reduction in mortality for patients with severe sepsis or septic shock when treatment was guided by central venous saturation.6 Some groups have decided to use the proportion of patients who have central venous saturation higher than 70% in the first 6 hours as a quality marker. This is flawed. The clinical trial did not study achieving a central venous saturation of 70%, but trying to achieve it. Some patients will never achieve it, due to individual characteristics, while others will get there regardless of the treatment provided. A hospital might look like a poor quality center with low rates of “achieving 70% central venous saturation” simply because their patient population is particularly old or sick. The correct quality metrics would be compliance with processes of care used to achieve the goal, for example, the proportion of patients with low central venous saturations that received protocol guided treatment in the first 6 hours.
Relevant: Indicators need to be based on evidence that they lead to improved outcomes and that the outcomes themselves are relevant. An indicator must be accepted by the main stakeholders, including patients, families, clinicians, hospital managers, policy makers, and service buyers. For health care providers, indicators that are based on available scientific evidence are preferred in relation to indicators selected according to nonscientific criteria or availability. Using indicators that do not have sound resonance from stakeholders is bound to be received with resistance and either disregarded or subjected to data manipulation in conscious or unconscious ways. A good example is the use of nighttime discharges as a quality metric. Although one study demonstrated an association of nighttime discharges with mortality in the ICU,7 its external validity is threatened by differences in health care systems, and different ICUs may not demonstrate the same association. In this situation, it would be difficult to convince stakeholders that nighttime discharge is a good quality indicator when local data demonstrate its safety.
Timely: To be helpful in influencing decisions, indicators must be available in time to allow for actions. Learning that an ICU’s rate of compliance with a daily interruption of sedation protocol was low 6 months ago is less helpful than observing monthly compliance to allow for more immediate actions to be taken. Outcome-based quality indicators, such as mortality and infection rates, frequently fail this item as ICUs require a long-time frame to have enough numbers of events to allow for an accurate description of the population.
Indicators can be measured and reported in various ways. A rate-based indicator uses data about events that are expected to occur with some frequency. These can be expressed as proportions or rates (proportions within a given time period) for a sample population. To permit comparisons among providers or trends over time, proportion- or rate-based indicators need both a numerator and a denominator specifying the population at risk for an event and the period of time over which the event may take place. Examples of common indicators that are proportion or rate based include infection rates (number of central line infections [CLIs] per 1000 central line days) and compliance with preestablished protocols (number of patients receiving an SBT per number of patients eligible for an SBT). An important challenge in proportion- or rate-based indicators is defining the denominator population eligible for the quality measure. Indicators can be reported as a single continuous value. The most common continuous quality indicator is time. Examples would be time to hypothermia after cardiac arrest and time to antibiotics in severe sepsis. Of course, continuous measures can be dichotomized into a proportion particularly when there is evidence that there is an optimal threshold value. Finally, indicators can be reported as a count of sentinel events. These identify individual events or phenomena that are intrinsically undesirable, and always trigger further analysis and investigation. Each incident would trigger an analysis of the event and lead to recommendations to improve the system. Examples of indicators that can be used as sentinels are medication errors, cardiac arrest during procedure, and arterial cannulation of major vessels during central line insertions.
RESEARCH CONCEPTS RELEVANT TO QUALITY MEASUREMENT
Clinicians, managers, and clients will need to decide, based on a panel of indicators, whether the quality of care is adequate or not. In essence, users of these data are trying to draw a causal inference between the observed data, specifically the quality indicators, and quality of care.8 Therefore, readers of quality reports should approach these data with the same criteria for validity as we apply to causal associations in research data, namely chance, bias, regression to the mean, confounding, and secular trends (see Table 2-2). Incorrect conclusions about quality are possible if these are ignored.
Research Concepts Relevant to Quality Measurement
Statistical Concept | Definition | Solution |
---|---|---|
Chance | The association is not real; it occurs by a random error. | Calculate p values, increase sample size, increase precision of the measurement, and choose more common events. |
Bias | The association is not real; it occurs by a systematic deviation from reality. Biases can be nondifferential (when the measurement is biased in all samples) or differential (when the measurement is biased in only one sample). | Ensure that indicators are measured with the same definition in the different units or over time. Increase precision of the measurement (eg, using a standard definition). |
Regression to the mean | The association is not real. It occurs between two weakly correlated measures when one of the values is in the extremes; the next measurement will move in the opposite direction. | Repeat measure over time. Do not take actions on isolated extreme values as they are likely to return toward the baseline. |
Confounding | The association is real, but the cause of the differences observed is not due to quality of care, but to a third variable that is associated with both the quality indicator and the different units (or over time). | Identify possible confounders before collecting data. Restrict analysis to a subset of patients without the confounder or use an adjusted analysis. Avoid inferring differences in quality of care across units if the case mix is considerably different. |
Secular trends | The association is real, but the quality indicator would be improving in spite of efforts for improvement. There is no real cause-effect. | Analyze interrupted time series. Not an important issue for demonstrating that quality is improving over time, but causality should not be inferred. |
Imagine that two ICUs in the same hospital are measuring their VAP rates. Assume that in reality, there is no difference in the VAP rates between units. At any given time period, it is conceivable that one unit will have a VAP rate of 10/1000 mechanical ventilation days, while the other will have a VAP rate of 4/1000 mechanical ventilation days. This type of association could occur spuriously just by chance. To avoid this type of random error, quality indicators should be formally compared with statistical tests, to quantify the magnitude of the association that could be due to chance alone. This is usually demonstrated with p values or confidence intervals, which gives us a sense of the probability that chance explains the results. In the example above, one unit could have five VAPs over 500 mechanical ventilation days and the other unit one over 250 days. Although the rates seem to be 2.5 times higher in the poorly performing ICU, the p value in this case would be 0.12 and the 95% confidence interval of the relative risk would be from 0.39 to 16. These results would, therefore, be expected to occur by chance alone one out of every eight measurements and the 2.5 times increase in VAP rates would also be compatible with an actual decrease in VAP of 60%. Analyses of rates are particularly unstable when studying rare events over short periods where a single event can lead to apparently large differences in rates.
Strategies to decrease chance include sampling a larger number of patients, choosing processes and outcomes that are more frequent, and increasing the precision of measurements. For example, a continuous variable that measures the time to delivery of antibiotics is a more precise measure of quality than the proportion of patients who receive antibiotics in less than 1 hour and would require fewer patients to demonstrate differences in quality at the expense of a less interpretable quality measure.
Bias can be defined as a systematic deviation from reality. Efforts should be made to avoid introducing biases in data collection for quality indicators. While there are many sources of bias, there are fundamentally two types: nondifferential and differential. Nondifferential bias introduces noise but not a deviation into the measurement. For example, using physician documentation as the measure of VAP presumably would both over- and underdiagnose VAP depending on a variety of physician factors. The major problem with nondifferential bias is that the noise introduced will obscure actual quality differences. To solve this problem, a protocol with objective parameters for detecting VAPs should be used.9
More troublesome is when quality indicators are measured in different ways across units or in the same unit over time. When ICUs or hospitals are compared for outcome measures or an ICU is monitoring its quality over time, it is assumed that there is no differential bias in the way the indicators were collected. Differential biases are more challenging than nondifferential because instead of introducing noise, they introduce a signal, but it is a flawed signal. Differential biases can be subtle. If a standardized definition requires detection of bacteria in sputum, an ICU that has a policy of ordering sputum cultures for every febrile patient will have a higher VAP rate due to colonization than an ICU that has a protocol for selective ordering of sputum cultures. Similar problems could exist even in more objective indicators, such as time to cooling after cardiac arrest. If time zero is defined in one ICU as the time of hospital arrival and in another ICU as the time of arrest, differences in the quality marker simply indicate a biased measurement.
Regression to the mean is a recurring statistical phenomenon that has serious implications for the interpretation of changes in quality indicators.10 The classic medical example is screening a population for elevated blood pressure and offering treatment to those with hypertension. Regardless of the efficacy of this treatment, the next set of blood pressures will be lower. The same phenomenon occurs in quality. This can clearly be a problem when selecting outcomes to improve or even selecting hospitals with a quality problem. Since the labeled outliers may not be real outliers, their ratings will improve in the next measurement regardless of the presence of a quality issue or the efficacy of the quality improvement project. Many before-after quality improvement projects suffer from this potential error. One of the solutions to this problem is the use of serial measurements of quality indicators. Therefore, trends over time demonstrating consistently poor quality prior to an intervention and sustained improvement after are the best insurance against regression to the mean.
Just as epidemiologists are aware of confounding variables when drawing conclusions about causality, quality scientists must be aware of these variables. Confounding measures are those that are associated with the ICU and the quality measure but do not necessarily cause the problem. For example, if it is known that patients post-cardiovascular surgery are less prone to develop a VAP compared to patients intubated for shock, the comparison between units could be confounded if the patient demographics in the ICUs are very different. Obviously, this is less of a problem when following a single unit over time; however, major variations in the case mix of an ICU over time could cause this phenomenon. There are standard approaches to address confounding. Restriction excludes certain subsets of patients where the quality measure is known to be more or less common. Adjustment mathematically balances confounding factors across sites. The most common approach would be to use a severity of illness measure to adjust the risk of death in analyzing mortality differences between ICUs.
Quality indicators may improve over time for reasons apart from specific efforts to change practice. These changes, usually called secular trends, are not necessarily problematic when the aim is to demonstrate that quality is improving over time, but may be misleading when the data are used to attribute the changes to a specific intervention. An excellent example of this problem can be seen from the original description of the central line bundle to decrease CLIs.11 The published report demonstrated a significant decrease in CLI rates, from 2.7 to 0 per 1000 catheter-days. The reported rates are likely correct, but at the same time CLIs were decreasing without the implementation of the bundle.12 Therefore, what can be concluded is that there is a real decrease in CLI rates over time, but the use of the bundle may or may not be the cause, as rates may have been declining due to secular trends. To solve this problem when trying to infer causality, different models of analysis, beyond the scope of this chapter, should be used, such as an interrupted time series or controlled interrupted time series.13
Some of the statistical problems discussed can be addressed with a simple monitoring tool, the statistical control chart (SCC). Chance, regression to the mean, and secular trends are addressed by SCCs. This approach has its origin in industry and was initially developed in 1924 by Walter Shewhart at Bell Laboratories, but is widely applicable in health care, under multiple formats, depending on the type of data available.14 Briefly, SCCs use statistical methods to distinguish random variability from special-cause variation from real changes introduced into the system. For example, although the rates of self-extubations in ICUs are relatively constant, there may be variations in the exact number during any given month. SCCs are designed to distinguish random variation, which is not interesting to clinicians from special-cause variation due to changes in, for example, a sedation protocol.
An SCC relies on serial measurements of the process or outcome of interest in the population or a random subset of patients. In ICUs, these measurements may take any of the indicator forms: proportions, rates, continuous measures, or indicators. The type of data is important as it defines what type of distribution will be used to construct the SCC. Different types of data require different types of control charts, which use specific formulas for the graphs. The reader is referred elsewhere for a more in-depth discussion.14,15
After understanding what types of data are in use, each data point is plotted in a graph, organized by time on the x-axis and the results on the y-axis. Three lines are then constructed: a center line (CL), which usually uses the arithmetic mean of the process, but can also use the median or an expected value. Then two lines are traced, the upper control line (UC) and lower control line (LC), using three standard deviations (SD) above and below the CL.14
When a measurement is observed outside the UC or LC lines, the process has undergone a special-cause, or nonrandom, variation. Other, more complex, rules exist, such as drawing control lines at two SD and identifying two out of three points outside the lines as special variation. Trends are also important, and a sequence of seven points moving in the same direction (either increasing or decreasing) also points toward special-cause variation. To conclude that a process is under control, stability of at least 25 data points is required.
MEASURING TO IMPROVE
There is a growing interest in using quality measurements to identify high- and low-quality performers at a systems level, which would prompt actions to help low performers improve. Examples of such initiatives include the UK star system,16 Canada’s HSMR system,17 and the New York State Department of Health reporting of adjusted mortality after coronary artery bypass graft surgery.18 In fact, public reporting of hospital performance has been proposed as a means of improving quality of care while ensuring both transparency and accountability.19 A recently published systematic review of 45 articles examined the evidence that public reporting actually improves quality. Eleven studies suggested that public reporting increased quality improvement activities in hospitals, with 20% to 50% of hospitals implementing changes in response to the reports. The relationship between public reporting and improved outcomes is less clear. New York State has implemented a public reporting system on cardiac surgery since 1991.20 Although several reports point toward decreased mortality after the introduction of the system,21 concurrent data from other states that did not introduce public reporting demonstrated that the decrease in mortality occurred at similar rate, which questions the real effect of the statewide reporting system.22
Public reporting clearly creates the incentive to improve performance, but does not necessarily direct providers on how to improve. Expectations would be that improved metrics would be preceded by efforts to implement evidence-based practices. However, metrics can also be improved by avoiding high-risk patients or by manipulating the way the indicator is measured.23 In fact, many of the perceived improvements in cardiac surgery outcomes from public reporting in New York State were due to these changes.24 Higher-risk patients in New York were also less likely to receive percutaneous coronary intervention (PCI) than were those in Michigan, which did not have PCI public reporting.25 This migration of patients to other states not only biases the reports, but has the negative consequences of overwhelming neighboring health systems and ignoring patient preferences for care.
Other unintended consequences include the widespread adoption of default therapies to patients who may not need them to enhance quality measures. For example, observational studies suggest an absolute reduction of 1% in mortality when antibiotics are administered early (within 4 hours of hospital arrival) for patients with community-acquired pneumonia (CAP).26 Notwithstanding the small benefit of the proposed process of care, this association was the basis for the recommendation that antibiotics should be administered in less than 4 hours for patients with CAP, which was endorsed by the Infectious Diseases Society of America (IDSA),27 and later by the National Quality Forum, the Joint Commission, and the Centers for Medicare & Medicaid Services. This measure has since been publicly reported for all US hospitals, which drove some hospitals to adopt policies mandating antibiotic administration even before chest radiographs were obtained.28 The imposition was followed by several studies challenging the quality indicator: One study observed that 22% of patients with CAP had uncertain presentations (often lacking infiltrates on chest radiography), where delayed antibiotics would be appropriate29; other studies demonstrated that the 4-hour policy led to increased misdiagnosis of CAP, with concurrent increased antibiotic use for patients who did not have CAP30,31; more recently, prospective cohorts have failed to demonstrate any association between early antibiotics and treatment failure for CAP.32 These unintended consequences led the IDSA to revise their guidelines and exclude a fixed time frame for antibiotic use, recommending that antibiotics be administered as soon as a definitive diagnosis of CAP is made.33
Risk-adjusted mortality is a common tool used to measure and benchmark the quality of intensive care. This measurement can be thought of as a “test” to diagnose whether an ICU has high quality or not. We can apply the same criteria of validity, reliability, chance, confounding, and bias to see if the application of risk-adjusted mortality can be used to identify quality. Unfortunately, using simulations Hofer demonstrated that both sensitivities and positive predictive values are inadequate. Depending on the case mix, sensitivities would range from 8% to 10% (ie, approximately 90% of low performers would not be detected) and positive predictive values would range from 16% to 24% (which means that 76% to 84% of units classified as low performers would actually be average or high performers).34 Risk-adjusted mortality and its more commonly reported version, the standardized mortality ratio, certainly have uses; however, the limitations of these measures are well documented.35
It is still unclear whether there is value in public reporting of quality measures in either driving the market to use high-quality centers or motivating quality improvement. It is clear that payers, governments, and consumers are likely to demand these reports in the future. The challenge then becomes how to apply a rigorous methodology to the data collection, implementation of changes, and analysis of effectiveness both at the local and system levels.
While there are many newer formulations, the classic model proposed by Donabedian36 separated quality into three domains: structure, process, or outcome of health care, the rationale being that adequate structure and process should lead to adequate outcomes37; however, this has not always been the case and in fact process and outcomes frequently do not move in the same direction.38
Structure measures the attributes of the settings in which care occurs. This includes facilities, equipment, human resources, and organizational structure. Process measures what is actually done in providing care, including treatments, diagnostic tests, and all interactions with the patient. Outcome measures attempt to describe the effects of care on the health status of patients and populations such as mortality and health-related quality of life. Broader definitions of outcome include improvements in the patient’s knowledge, behavior, and satisfaction with care.
If we combine the above domains of structure, process, and outcomes with the methodological concepts described in the previous section, we can summarize a model of quality of care that is influenced by the variability of its different components (adapted from Lilford39):
From this equation, the rationale for using risk-adjusted outcome rates is clear. By controlling the variation due to case mix and expressing the effects of chance, these models attempt to expose the residual unexplained variation, which is attributable to quality of care. This leads naturally to the ranking of hospitals according to risk-adjusted mortality rates with an implied correlation with quality of care. From the above model, it is clear that these assumptions are overly simplistic. Differences in the definitions and quality of data can lead to differential bias and upcoding of severity of illness. Despite using protocolized data collection, measures of case mix, even in critical care where they are highly evolved, are imperfect. Using data from Project IMPACT, a multicenter cohort of ICUs that carefully collects data on quality of care, Glance customized SAPS II and MPM II scoring systems and used it to rank 54 hospitals based on their risk-adjusted mortality. The two different scores led to differences in classification of 17 ICUs, including some that would be classified as low performers under one model, but as high performers under the other model.40 The possibility of outlier misclassification suggests that risk-adjustment models are poorly suited to claim differences in quality of care. However, when using process-based measurements, the sources of variability decrease considerably.

Full access? Get Clinical Tree
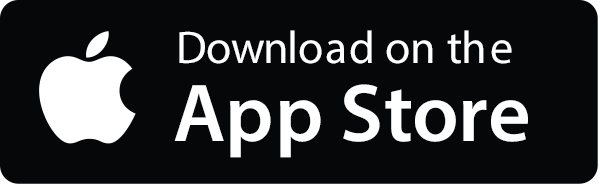
