KEY POINTS
Effective critical care practice requires a rational approach to understanding, interpreting, and integrating clinical research studies, outcome measures, measures of association, and statistical testing relevant to research in intensive care units.
Clinical research studies generally fall into one of two categories: observational studies or experimental studies, and each study type has different strengths and weaknesses.
The goal of the observation is to evaluate associations between exposures and one or more outcomes of interest to investigators. The randomized controlled trial (RCT) is an important experimental design used to assess the efficacy of a medical intervention.
Critical care research frequently relies on surrogate end points that allow demonstration of treatment effect with fewer patients over less time. Trials using surrogate end points should be interpreted with great caution.
Appropriate interpretation of the results of treatment trials requires clear understanding of measures of association, including both relative risk and absolute and relative risk reduction (RRR). Making an educated decision about the application of a study’s findings to one’s patients also necessitates assessing the number needed to treat (NNT) to see a benefit to the population.
Evaluating clinical research evidence also requires addressing the meaning of p values and confidence intervals. These statistical measures aid the assessment of whether observed differences in outcomes between groups reflect true differences or simply chance variation.
To correctly interpret a variety of diagnostic tests, one must understand how well that test reflects the actual presence or absence of disease in any given patient. The sensitivity and specificity of a given test reflect how closely the result of that test reflects the “truth” about a patient’s disease process.
Qualitative methods can serve a variety of purposes in critical care research and should be reviewed no less critically than quantitative methods.
INTRODUCTION
Without a rational approach to interpreting and applying research findings at the bedside, clinicians can be frustrated in their efforts to integrate the results of empirical studies into the care of their patients. Here we review important elements of clinical research study design, outcome measures, measures of association, and statistical testing relevant to research in intensive care units (ICUs). We also discuss the nature and role of qualitative research in intensive care medicine and summarize strategies to assess the rigor of a qualitative research study.
STUDY DESIGN AND RELATED ISSUES
Clinical research studies generally fall into one of two categories: observational studies or experimental studies. Observational studies may include case series, case-control studies, prospective cohort studies, and cross-sectional studies. Each type of observational study has different strengths and weaknesses, but all involve observing the results of a subject’s exposure to a factor of interest that was introduced independent of a research protocol. The goal of the observation is to evaluate associations between exposures and one or more outcomes of interest to investigators. Although observational studies can help identify associations between exposures and outcomes, they generally cannot be used to establish a causal link between the predictor and outcome of interest.1
There are numerous well-known examples in which the results of an observational study suggested a causal link that did not withstand the scrutiny of further scientific testing. One example is the effect of hormone replacement therapy on coronary heart disease.2 Early observational studies suggested that hormone replacement therapy was significantly protective against coronary heart disease, but randomized trials later showed that hormone replacement therapy either had no impact on coronary heart disease or increased the risk of disease.3,4 A variety of reasons for these differences have been suggested, all relating to potentially unidentified confounders in the observational study.
When assessing an observational study, one must be aware that such studies are subject to a variety of types of confounding and bias. Confounding, in which a factor is associated with both a predictor or risk factor and the outcome being studied, can have the effect of appearing either to strengthen or weaken the association between the predictor and the outcome. One very common type of confounding in observational studies is confounding by indication. This type of confounding occurs because those who receive treatment in an observational study are more likely to have worse disease than those who do not receive treatment. In this case, a poor outcome may be erroneously associated with the treatment rather than the disease that actually caused it.5
Bias in observational studies, which results from systematic errors in the design or conduct of a study,6 falls into two major categories: selection bias and information bias. Selection bias results when individuals have differing probabilities of being included in the study sample based on a factor that is relevant to the study design. Information bias results in systematic misclassification of participants in a study based on a variety of sources of misinformation including recall bias, interviewer bias, observer bias, and respondent bias.6 Both confounding and the influence of information bias introduced by loss to follow-up are discussed below in our examination of randomized controlled trials.
The randomized controlled trial (RCT) is an important experimental design used to assess the efficacy of a medical intervention. In RCTs, subjects are randomly assigned to either the treatment or control group. The process of randomization minimizes the risk of confounding because it increases the likelihood that both known and unknown confounders will be equally distributed between the two groups.
Assessing Study Validity: Several factors should be carefully considered by the reader of any RCT before deciding whether the results of the trial are valid, including randomization, blinding, loss to follow-up, and post-randomization confounding.
Randomization Critical evaluation of an RCT should include a comparison of the control and treatment groups at baseline to ensure that potential confounders have been adequately balanced between the two groups by the randomization process. This evaluation is especially important for small studies in which randomization does not always result in equivalency between groups at baseline.
Blinding Blinding (or masking) refers to the process by which study participants or investigators are prevented from knowing to which study group subjects have been assigned. Blinding of both the investigator and the research subject (double-blinding) protects against bias that may arise from either one being aware of the group to which the research participant was randomized. Blinding of the investigator assessing outcomes is especially important if the outcome being measured is subjective, as with a self-reported measure of post-ICU quality of life.
Loss to Follow-Up It is also necessary to carefully assess the adequacy of follow-up when evaluating the validity of study findings. Loss to follow-up can occur in either differential or nondifferential fashion. Non-differential loss to follow-up involves loss of subjects who are not different in important respects from those for whom follow-up data are obtained. Non-differential losses usually result in a loss of power since there will be fewer participants than planned at the final analysis. Such underpowered RCTs are problematic because they often produce falsely negative findings, resulting in missed opportunities to identify beneficial therapies. Differential loss to follow-up presents a more challenging problem. In this case, those who are not followed through to the end of the study are in some way systematically different from those who are observed throughout entire the study period. Differential losses result in both loss of power and potential bias in the findings due to uncontrolled confounders. It has been argued that readers can do a rudimentary assessment of the potential impact of loss to follow-up by assuming that all losses from the treatment group had poor outcomes and all losses from the control group had positive outcomes. Recalculating the overall outcome using this assumption provides an estimate of the impact of those losses.7
Post-Randomization Confounding Confounding may enter in after the randomization process. A recent study of extracorporeal membrane oxygenation (ECMO) for management of acute respiratory failure by Peek et al randomized subjects with acute respiratory failure to either routine critical care management or referral to an ECMO center.8 That study documented better outcomes in the patients randomized to referral for management at the ECMO center. By definition, the potential for confounding exists when factor A, in this case, care at a tertiary referral center, may be associated with improved outcomes, and is also related to Factor B, in this case, management using ECMO, but is not a result of Factor A. Critics have argued, in fact, that the improved in outcomes may have been related to overall improved care at the single referral center rather than the ECMO intervention itself.9
THE PROBLEM OF SURROGATE OUTCOMES MEASURES IN CRITICAL CARE RESEARCH
Before implementing a new treatment, clinicians would ideally like to know what that treatment’s impact will be on important patient-centered outcomes such as mortality and quality of life. Critical care research, however, is often both complex and costly. The time and resources needed to carry out studies that are adequately powered to detect a mortality difference sometimes make them infeasible. Therefore, critical care research not infrequently relies on surrogate end points that allow demonstration of treatment effect with fewer patients over less time.1
Trials using surrogate end points should be interpreted with great caution. Acceptable surrogate end points are those that have been validated as a marker for the disease outcome of interest. Few surrogate markers meet this criterion. There have been important examples in critical care research in which a surrogate end point has suggested that a therapy was beneficial when it was in fact harmful.10
Investigations of partial liquid ventilation (PLV) for acute respiratory distress syndrome (ARDS) in adults are an example of this problem. Early studies of PLV for ARDS demonstrated significant improvements in oxygenation,11 and some interpreted these findings to mean that the treatment was beneficial for patients. However, subsequent studies failed to show any impact on mortality.12,13
Combined end points have been used in some critical care research as a means to identify clinically meaningful outcomes with fewer patients.14 A commonly used combined end point in critical care research is ventilator-free days (VFDs), which measures the amount of time a patient is alive and not on a mechanical ventilator, usually over 28 days.10 There are a number of problems with an outcome measure like VFD.15,16 Although a thorough examination of combined end points is beyond the scope of this chapter, it is important to remember that studies have demonstrated improvements in mortality even without differences in VFD17 and, further, VFD as an end point assumes that the end points of mortality and prolonged mechanical ventilation are of equal weight.15
MEASURES OF ASSOCIATION AND QUANTIFYING EFFECT SIZE
Appropriate interpretation of the results of treatment trials requires clear understanding of measures of association, including both relative risk and absolute and relative risk reduction (RRR). Making an educated decision about the application of a study’s findings to one’s patients also necessitates assessing the number needed to treat to see a benefit to the population.
The relative risk (RR), also called the risk ratio, for a given outcome in a study is calculated by dividing the risk in the treatment group by the risk in the placebo group. The RRR is calculated by subtracting the RR from 1, and the absolute risk reduction is simply calculated by subtracting the risk in the control group from the risk in the intervention group. Consider the following hypothetical example:
An RCT enrolls 400 patients to receive antibiotics or placebo in an effort to decrease the incidence of ventilator-associated pneumonia (VAP). A total of 200 patients are assigned to receive antibiotics and 200 are assigned to receive placebo. Ten patients in the antibiotic group and 15 in the placebo group get VAP. Therefore
And the RR is
The RRR is 0.33 or 33% and the absolute risk reduction is 0.025 or 2.5%. The statistical significance of RR is measured by the 95% confidence interval which, as we will discuss further below, tells us the range of values that is most consistent with the true RR.
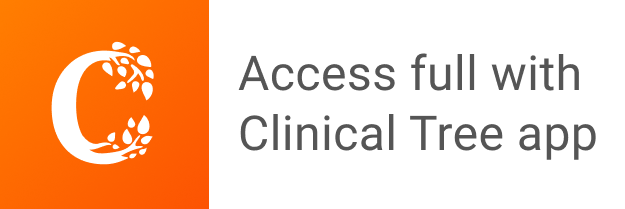