Fig. 3.1
Representative Survival curves (
) and their annual mortality rates (
) of populations for which in the first year: (a), 5 % die; (b), 10 % die; (c), 15 % die. For comparison, survival and mortality rates when a constant number of people die (
and
) and a constant proportion of people die (
and
)






Figure 3.1b shows a cohort with a mortality rate of 0.10 (10 %) in the first year, that is double the rate of the cohort in Fig. 3.1a. Unsurprisingly the median life expectancy, when the population has halved, is shorter in Fig. 3.1b than in Fig. 3.1a. In the first cohort the median life expectancy is 8 years and in the second cohort it is 4.6 years: the number dying in Fig. 3.1b does not remain double that in Fig. 3.1a, if it did, one would expect the median life expectancy to be halved (as shown by the black lines). The dashed black line in Fig. 3.1b illustrates clearly how a constant number of deaths results in an increasing proportion of deaths, also shown in Fig. 3.1c, in which the first year mortality rate is 0.15 (15 %). These two figures also show how the mortality rate in the population, which initially accelerated after the first year, levels off and might even decrease (Fig. 3.1c), although one should be circumspect that mortality rates truly decrease as the data on survivors are limited for this part of the population.
Everybody in a cohort dies, if you wait long enough, which would allow you to draw lines that terminated on the horizontal axis. However, even researchers who are prepared to observe longer than one lunar cycle want to publish their research without having to wait for everyone to die. Cohorts are usually recruited over a period of time, some people might be observed for nine postoperative years and others for nine postoperative days. You could draw a simple survival curve for the whole cohort, but it would only extend for nine postoperative days, so you would waste the 3278 additional days that you have observed the patients recruited first, 9 years ago. Alternatively, you could generate a survival curve up to 9 years, but how should you treat patients you’ve observed for less than 9 years who were still alive when you last checked? You don’t know when patients will die, but if you have data for other patients who’ve died during a longer observation period you can hazard a guess i.e. you can estimate the survival curve that is the composite of patients who survived for the whole of the longest observation period, patients who died, and patients who survived but who have not been observed for the whole observation period (‘censored’ patients). The most commonly-used estimate of survival data is that described by Edward Kaplan and Paul Meier in 1958, i.e. the Kaplan-Meier estimator [3].
3.3 The Kaplan-Meier Estimator
In this chapter we’re looking at survival; time to death. ‘Survival’ or time-to-event curves can be constructed for any binary state – it needn’t be ‘alive or dead’. All such curves are constructed in the same way.
Kaplan-Meier estimated curves are constructed by integrating or ‘sticking together’ mortality rates during brief intervals. The start time, time zero, is the time you start observing each patient in the cohort, irrespective of the particular date from which each patient was observed. An interval is the time to the next event, or for our purposes, the time to the next death, i.e. intervals vary in duration, determined by how long the next death in the cohort is from the preceding death. The mortality rate during the interval is the single death in the interval divided by the number observed for the whole period (including the patient who died). Censored patients whose observation period completed during the interval do not contribute to the calculation for that particular period. For instance, we might start an interval with 100 patients. Before the next death 10 patients are censored. The mortality rate for the interval is 1/90 and the survival rate is 89/90 (0.989). In the next interval 5 patients are censored. The mortality rate for the interval is 1/84 and the survival rate is 83/84 (0.988). The estimated cumulative survival at the end of the two intervals is 0.989 × 0.988, which is 0.977.
A table of the number of patients ‘at risk’ may be displayed below the Kaplan-Meier graph. Figure 3.2a is the Kaplan-Meier estimated survival after scheduled repair of abdominal aortic aneurysms in over 1000 patients [4]. By four postoperative years only 324 patients remain ‘at risk’ (33 % of the original cohort), but the estimated survival is 80 % i.e. estimated mortality is 20 % at 4 years. Shouldn’t the mortality be 67 %? What happened to the ‘unaccounted’ 47 % of the original cohort? You can see that there are ‘ticks’ attached to the survival line. These ticks mark the missing patients, indicating that they were censored i.e. at the marked times they were alive, after which they were unobserved, to live for ‘who knows’ how much longer. The estimated survival line is also bracketed by a shaded 95 % confidence interval. The corresponding mortality hazard estimate is illustrated in Fig. 3.2b, again bracketed by a 95 % confidence interval. You might note that the confidence interval is much wider around the hazard estimate than the survival estimate: this is because the survival estimate is cumulative whilst the smoothed hazard estimate in this particular example has been set to sample from single sequential months.


Fig. 3.2
Kaplan-Meier estimated curves (95 % CI) after scheduled abdominal aortic aneurysm repair in 1088 patients: (a), survival; (b), smoothed mortality hazard [4]
Open abdominal aortic aneurysm repair temporarily increases mortality tenfold and endovascular repair increases mortality fourfold. Approximately half the cohort in Fig. 3.2 had each type of aneurysm repair. The heightened mortality rate caused by surgery is evident in the initial steep survival curve and the steep decreasing mortality hazard curve. Mortality hazards generally increase with time: a decreasing mortality hazard demonstrates that the cohort has been exposed to an unusual hazard. One can envisage two general effects of major surgery on the normal population survival curve. The survivors might be left in a weakened state, so that the mortality hazard remains higher than expected (for an equivalent non-surgical population). Alternatively, surgery may have preferentially killed the weak, leaving the stronger, albeit numerically depleted cohort, which could have a mortality hazard less than expected.
3.4 Analysing Survival
Death within 30 days of surgery is usually analysed as a homogenous outcome: you’re alive or dead at 30 days, and if you’re dead it doesn’t matter whether you died on day 1 or day 30. The ease of such an analysis does not outweigh the disadvantage that the postoperative period is not representative of subsequent survival, in addition to which few deaths occur during such a short interval. It does matter when you die if mortality and survival are analysed over months or years.
Kaplan-Meier survival curves are analysed for two purposes: to determine whether patient characteristics are associated with survival; to determine whether an intervention changes survival. The most common statistical tests for both purposes assume a constant ratio of mortality hazards between two groups, for instance one with a characteristic and one without, or one exposed to an intervention and an unexposed group. This assumption holds perfectly true between two exponential survival curves, as illustrated by the blue lines in Fig. 3.3, for which the mortality hazard ratio remains two (dotted blue line). General population survival curves (red lines) maintain a fairly steady hazard ratio, but constant numbers of deaths in each year (black lines) exhibit a hazard ratio that rapidly increases with time (approaching infinity when survivors near zero in one cohort).


Fig. 3.3
Survival curves and mortality hazard ratios for three pairs of data: general population cohorts (
and
); constant numbers of deaths per year (
and
); and constant proportions of deaths per year (
and
)






The log-rank test calculates the probability that two survival curves are the same i.e. derived from the same population. Cox regression analyses can adjust the comparison for prognostic variables that might have been distributed differently in the two groups. Both types of analysis require fairly stable hazard ratios throughout the observation period. Hazard ratios that cross (with or without crossing of the survival curves) limit the power of these tests. There are alternative methods to analyse hazards ratios that cross, which can also allow one to weight early or late events: for instance, one might use survival analyses for length of postoperative stay and one might want to emphasise lengths of stay longer than the median as indicating a burden of morbidity. Standard log-rank tests do not permit this more nuanced exploration of the data. Researchers should familiarise themselves with Fleming-Harrington tests and Renyi supremum tests, for example, as disproportionate changes in hazards are likely in two groups if , for instance, one has had surgery and the other has not.
3.5 Principles for Picking Prognostic Factors
Firstly, we have to identify factors associated with survival. A logical but expensive method is to measure everything about the patient and their environment and then test for an association with survival. Another disadvantage of such a method is that one would falsely identify associations that are a consequence of data trawling.
Orthodox survival research might be characterised as some experiments testing whether a single new variable, for instance a genetic mutation, is a survival factor, whilst other experiments analyse known factors for associations with survival in a particular patient cohort.
We should make the peri-operative period part of the patient’s life. Most published papers in surgical and anaesthetic journals treat an operation in isolation [5–8]. Fragmentation of a patient’s life into time silos limits the power of each to identify survival factors, the importance of which might only be fully appreciated over the duration of a patient’s life, an example of which is age. Underpowered periods of observation not only miss important survival factors, they also generate spurious highly-significant associations. Spurious associations fragment the evidence base as they are interpreted as being real but context-sensitive i.e. specific to particular cohorts, whether due to patient factors, surgical factors or other factors. An approach to limit spurious associations is to include into all survival models those factors that have been proven over long observation periods i.e. in primary care. It is unnecessary and counterproductive to demonstrate statistical association of these default factors with survival in each cohort: they should be forced in to the model so that the associations of other variables with survival can be assessed in the context of known general survival factors. The association of age with survival, for instance, is not suspended by walking through the hospital doors.
We want to estimate survival as accurately as possible, without underestimation or overestimation. We therefore want to take into account all the prognostic associations that are independent of all the other prognostic associations: we don’t want to miss any prognostic factors, but we don’t want to double-count associations that are shared with other factors. Researchers usually test survival association with individual candidate variables, one at a time. It would be more logical to test candidate variables with the default survival variables, rather than in isolation. The threshold p value for choosing candidate variables for the multivariable analyses is a matter of choice, but is often set at 0.10 rather than 0.05. One could argue that smaller p values would encourage researchers to observe survival in larger cohorts over longer periods of time, which would reduce spurious associations. Interactions between candidate factors should also be tested, with interactions being included or excluded independently of the parent variables.
The association of each selected variable that is independent of other variables is determined in a multivariable model. The final multivariable model is selected from candidate models, each of which contains different combinations of the candidate factors. Stepwise selection is usual, by sequentially including or excluding candidate factors. There are a number of metrics that can be used to determine whether the survival model is improved by the inclusion of a candidate factor (or interaction term), including the Akaike information criterion and the false discovery rate. The problem encountered by all studies is that the selected model cannot be properly applied to the cohort used to generate the model: the performance of the model can only be properly assessed on an independent cohort. The performance of a model within the cohort from which it was derived overestimates its performance: it performs less well when applied to other cohorts – it is ‘over-fitted’.
3.6 Year, Age and Sex
These three variables by default should contribute to any survival model because they strongly associate with general survival [1].
Mortality rates have fallen by about 2 % each year for at least five decades, with a corresponding extension to survival (Fig. 3.4). Age is the most important survival variable (Fig. 3.5). The difference in survival between men and women has reduced over the past five decades. The current lifetime difference in median survival is around 4.5 years now (2015), with the difference in median life expectancies at 90 years and 80 years of age being 1 year and 2 years, respectively (Fig. 3.6).




Fig. 3.4
Survival for an average 75 year old man in 1983, 1993, 2003 and 2013 (left to right). The median life expectancy increases from 6.7 years, to 7.5 years, 9.7 years and 11.5 years

Fig. 3.5
Survival for average men aged 90, 80, 70 or 60 years old (left to right)

Fig. 3.6
Survival for men (
) and women (
) aged 90 years and 80 years (left to right)


3.7 Organ Ischaemia and Failure
Acute coronary syndrome, stroke and peripheral arterial disease independently decrease long-term survival. Heart failure and cognitive impairment also decrease survival. A broad-brush estimate is that each of these diagnoses independently increases mortality hazard 1.5–2.0 times [9–22]. Functional tests, including physical fitness, increase the precision of this estimate (see below). The cumulative relative mortality hazard associated with multiple diagnoses is their product: for instance, two of these diagnoses would increase the mortality hazard by 2.25 times compared with someone without either diagnosis, if one assumes each increases mortality by 1.5 times. If a patient has not had an acute coronary syndrome or stroke their mortality hazard is increased by about 1.2 times for angina and TIAs.
Renal dysfunction increases the mortality hazard [23]. The estimated glomerular filtration rate permits one to use a ‘sliding scale’ of risk associated with the severity of the dysfunction. The expected glomerular filtration rate (ml.min−1.1.73 m−2) is (0.003 × age × age) – (1.017 × age) + 139.4. The Cockcroft-Gault estimated filtration rate is ((140 – age) × mass × number) ÷ creatinine (μmol.l−1), where ‘number is 1.04 for women and 1.23 for men. The mortality hazard increases by 1.0085 times per ml.min−1.1.73 m−2 that the estimated filtration rate is below the expected rate.
3.8 Whole Body Fitness
Physical fitness is an independent survival factor [24, 25]. Its measurement also serves to gauge the functional impact of other survival factors, for instance a stroke, heart failure or angina. It is unclear whether a single functional test can capture the prognostic information associated with physical function. There is certainly substantial overlap in the prognostic value of functional tests. The multiplication of the mortality hazards associated with two measures of function is likely to severely overestimate the hazard of physical dysfunction.
A pertinent example for the peri-operative physician of the problems of measuring different but dependent variables is the cardiopulmonary exercise test (CPET). The CPET measures five main variables during incrementally harder exercise, usually by increasing the braking on a cycle ergometer or the speed and slope of a treadmill: power; oxygen consumption; carbon dioxide excretion; minute ventilation; and heart rate. Particular features of these measurements are extracted for prognostic testing, which include oxygen consumption, both peak and at the anaerobic threshold, and the ratios of minute ventilation to oxygen consumption and particularly to carbon dioxide excretion (often at the anaerobic threshold).
These measurements are different. However, their deviation from normal values results from abnormal function. The dysfunction is of the whole body, so the prognostic overlap of these variables is substantial. Table 3.1 is taken from reference [4], with permission. Table 3.1a lists the univariate associations of different variables with survival after scheduled abdominal aortic aneurysm repair. The column titled ‘z’ indicates how much the variables were associated with survival, the effect increasing with the difference from zero. The column titled ‘p value’ indicates the precision of that effect. In isolation the peak power was the factor most associated with postoperative survival, followed by age. The other CPET variables were also strongly associated with survival. You might like to note that serum creatinine concentration was only weakly associated with survival, whereas the estimated glomerular filtration rate was strongly associated with survival. When the prognostic overlap between variables was accounted for in multivariable analysis (Table 3.1b), only four variables contributed statistically independent prognostic information (sex and date were retained for the reasons explained previously): age; renal function; peak oxygen consumption; and the ventilatory equivalent for carbon dioxide excretion. Although in this particular dataset peak oxygen consumption and ventilatory equivalents for carbon dioxide carried the available CPET prognostic information, another dataset might be characterised by a different combination of CPET variables. In any dataset a single CPET variable will be dominant, perhaps largely by chance, and will be incorporated in the multivariable model. In all cases the prognostic value of the other CPET variables will be largely exhausted, due to the prognostic overlap with the dominant CPET variable.
Table 3.1
Univariate (a) and multivariate (b) analyses of preoperative variables associated with postoperative survival in 1096 patients who had scheduled repair of abdominal aortic aneurysms

Full access? Get Clinical Tree
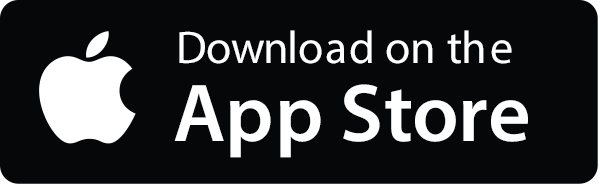

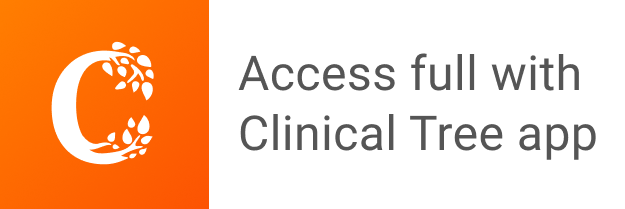