FIGURE 7.1 The “measurement value of X” represents numerical data, such as PaO2/FiO2 ratio. A histogram of sample data is graphed with bars indicating the relative frequency of observations in a given interval. The population of measurements is distributed according to a probability density curve (dashed line).
MOTIVATIONS FOR READING MEDICAL LITERATURE
There are many reasons for reading medical literature. Patients and family members often desire to educate themselves in order to become better advocates. Medical students and residents read articles for problem-based learning sessions and journal clubs. Physicians read articles in order to “keep up” with the medical literature and also to learn more about treating an individual patient. Physician researchers stay abreast of their research fields by regularly reading journals in their area of expertise. Physician researchers may also read articles in a peer-review process. Finally, policy-makers at various levels (e.g., government, payers, hospital, ICU directors) read and synthesize medical research in order to write guidelines and make informed policy decisions. Various reasons for reading medical literature will determine the extent of statistical expertise and rigor required for critical assessment. Herein, we will touch on basic statistical concepts, discuss common study designs, and provide guidelines for critical reading of medical literature. Interpreting the Medical Literature (22) is a useful resource for many consumers of medical journal articles. We also recommend The Handbook of Research Synthesis by Cooper and Hedges (23) for more detail on methodology for in-depth medical literature reviews including meta-analysis.
Basic Statistical Concepts
Statistics is the science of collecting, describing, and analyzing data, that provides the analytical framework for transforming information into knowledge. Of course, this knowledge is imperfect unless, perhaps, a biologic mechanism is identified that completely explains a phenomenon. Statistical methods quantify the imperfection of knowledge by providing results with an associated measure of error (e.g., level of significance, p-value, margin of error).
There are statistical concepts underpinning nearly every aspect of research, a process that includes the following six steps:
- Pose a research question and formulate into statistical terms
- Design a study
- Collect data
- Describe data
- Analyze data using statistical inference
- Answer the research question
Basic statistical concepts in the research process will be outlined below, but first, we give some vocabulary:
- Population—entire group of individuals of interest
- Sample—a subset of the population
- Data element—a measurement or observation on an individual
- Population parameter—a summarizing characteristic of all possible data values such as a population mean or proportion (the value of a population parameter is usually the object of a research question)1
- Statistic—any quantity calculated from data
- Inference—process of extending or generalizing information known about a sample to the entire population
- Probability distribution—assignment of probability to the possible values of data that could be observed
- Sampling distribution—assignment of probability to the possible values of a statistic that could be observed
Table 7.1 gives the notation for common population parameters and the corresponding statistics that estimate them. Figure 7.1 shows the relationship between a probability distribution (represented by the dotted line) and a histogram. The probability distribution of a set of all possible values of a measure (e.g., all possible ages, all possible values of the PaO2/FiO2 ratio, all possible plateau airway pressures, etc.) is conceptual and not observable, whereas a histogram can be graphed from sample data. We have insight into the actual shape of the probability distribution from observing the outline of the sample histogram; the more data we have, the more refined our histogram is, and the true shape of the probability distribution of the data emerges more clearly.
In the “big picture” view, the science of statistics turns information into knowledge by connecting the object of a research question (an unobservable population parameter) into evidence provided by a research study (observable data) through statistical theory. Here we will assume the frequentist paradigm. For example, an investigator asks a research question such as the following: “What is the incidence of ventilator-associated pneumonia?” The research question is then “translated” statistically into a question about a population parameter. The “incidence of VAP” is a population proportion (i.e., π). A research study will be designed, and data from a sample will be collected. To analyze the data, an inference will be made. In other words, information from the sample will be generalized to the entire population. Figure 7.2 illustrates a hypothetic population and sample. Each circle represents an individual in a critical care setting. Dark circles are patients who have VAP. As Figure 7.2 indicates, a valid inference depends on obtaining data from a sample that fairly represents the population of interest—hence, the concept of random sample, a sample free from systematic bias in the way it is chosen or retained in a study.
TABLE 7.1 Nomenclature and Symbols of Standard Parameters and Associated Statistics | ||
![]() |

FIGURE 7.2 Each circle represents an individual in a critical care setting. Dark circles are patients who have ventilator-associated pneumonia (VAP). When statistical inference is conducted, information from the sample is extended (or generalized) to the population of interest. Thus, the proportion of patients with VAP would be estimated to be 40% ± a margin of error based on the sample data.
Valid inference also depends on the selection of an appropriate statistical method to analyze data. Statistical methods are based on statistical and mathematical theory, assuming certain conditions (e.g., random sample, large sample size, normally distributed data, etc.) in order to provide valid results. Researchers (typically a team of physicians and biostatisticians) are responsible for choosing appropriate statistical methods and checking that no violations of assumptions have occurred. Statistical methods work like this: My research question is about a population parameter (e.g., incidence of VAP, a population proportion, π). Using my data, I can compute a statistic that estimates the unknown population parameter (e.g., incidence of VAP in my sample, a sample proportion, p). I will then apply the correct theory that will connect the statistic I have observed to the population parameter of interest. The theoretical connection occurs through the mathematical knowledge of the sampling distribution of a statistic. To better understand the idea of sampling distribution, refer to Table 7.2.
Suppose that 20 research teams are interested in estimating the incidence of VAP, and each team can observe 30 patients. Table 7.2 contains the raw data and the sample proportion (i.e., observed incidence of VAP) obtained in each study. In this conceptual framework, we can think of the statistic (e.g., sample proportion, p) as having a probability distribution (i.e., sampling distribution), which can be visualized by graphing a histogram (Fig. 7.3).
TABLE 7.2 Illustration of Central Limit Theorem: Simulated Data from 20 Hypothetical Research Studies and Calculated Incidence of Ventilator-Associated Pneumonia (VAP) | ||
![]() |

FIGURE 7.3 The histogram of ventilator-associated pneumonia (VAP) proportions taken from 20 hypothetical research studies is graphed. The shape of the histogram resembles a bell-shaped curve due to the Central Limit Theorem (CLT). According to the CLT, the curve will be centered at the true proportion of patients who develop VAP.
In the case of a sample proportion, the Central Limit Theorem (CLT) tells us that under certain conditions (i.e., random sample and large sample size), the shape of the sampling distribution will be normal, centered at the value “π.” Note that the histogram in Figure 7.3 has an approximately normal shape. The CLT also tells us that the center of the normal curve is “π,” the true incidence rate of VAP. Here is the power of the statistical method; even if we do not know the probability shape of the original data, under conditions that are not too difficult to achieve, we (approximately) know the probability shape of the statistic and its connection to the population parameter of interest. We can then use this connecting theory to conduct inference. This “central” idea (hence the namesake of the CLT) is depicted in Figure 7.4 for the case of numerical data—say, the ages of patients who develop VAP in a critical care unit. In this case, the CLT tells us that the shape of the sampling distribution of the sample mean () will be normal, centered at the value “μ”—the population mean—even though the probability distribution of the original data is not normal.
The theory underlying statistical methods generally requires the understanding of probability and calculus, and thus is something of a “black box” for many. For a more in-depth discussion about statistical inference, see Cox (24).
There are three basic goals of statistical inference:
- Estimation
- Test of hypothesis
- Prediction
In the estimation type of inference, the research question is concerned with estimating some characteristic or feature of a population of measurements (e.g., what is the incidence of VAP?). In the test of hypothesis type of inference, the research question is concerned with testing a relationship (e.g., does protocol-driven weaning reduce the incidence of VAP?). Finally, the prediction inference type of research question estimates a characteristic or feature of a population of measurements that will be observed in the future (e.g., what will be the incidence of VAP in 10 years’ time?). The form of statistical results will depend on the inference goal. In the case of estimation, the result will be reported in terms of a confidence interval. A confidence interval (CI) gives a plausible range of values for a population parameter such as a mean, a proportion, or an odds ratio, along with a measure of method success (i.e., the confidence). Tejerina et al. (25) found that VAP was present in 439 out of 2,897 patients, 13.2%; a 95% exact CI for VAP is given by (12.0%, 14.4%). This means that in 95 out of 100 studies, the true value of VAP incidence would be captured in the constructed confidence interval.2 The margin of error for the estimate is half the length of the CI (or 1.2%).
In the case of a test of hypothesis, the result will be reported in terms of a p-value.3 A test of hypothesis for testing a relationship works by setting up two competing hypotheses—the null (relationship does not exist) and the alternative (relationship exists)—and using the observed value of the data to provide evidence for rejecting or failing to reject the null. There are two potential errors that can occur: Type I (rejecting the null hypothesis when it is true) and type II (failing to reject the null hypothesis when it is false). The power of a test is the probability of rejecting the null hypothesis when it is false (the reverse of the probability of type I error). The observed value of the data (i.e., the value of the test statistic) provides a p-value that is compared to a predetermined level of significance, also known as alpha (α) or the probability of type I error. When the p-value is smaller than the set level of significance (typically set at 0.05), there is evidence for rejecting the null hypothesis and concluding that a relationship exists between two factors. When the p-value is larger than the set level of significance, then the test conclusion is to fail to reject null hypothesis. Evidence for concluding that there is no relationship between two factors depends on designing a study with adequate power to detect a “meaningful” relationship.
Tejerina et al. conducted a series of tests of hypotheses to test the relationship of factors thought to be associated with the development of VAP (e.g., gender, neuromuscular disease, sepsis, type of ventilation), finding a number of factors related to VAP at the 0.05 level of significance. For example, sepsis was significantly associated with development of VAP (p-value less than 0.001). The odds ratio of sepsis and VAP (estimate of the strength of association) was given as 19.9 with 95% CI as (15.7, 25.4). This means that the odds of developing VAP in patients with sepsis were 19.9 times higher than the odds of developing VAP in patients who did not have sepsis. The 95% CI gives a plausible range of values indicating that a feasible range for the odds ratio is as low as 15.7 and as high as 25.4. In 95 out of 100 studies, the true value of the odds ratio will be captured in the 95% CI.

FIGURE 7.4 Data is sampled from a probability distribution of any shape. In this illustration the original data comes from a distribution that is skewed right. Suppose multiple studies are conducted and for each study the statistic X– is calculated from the sample data. The Central Limit Theorem posits that the distribution of the statistic is normal with mean μ, the population mean of the original data.
In the case of inference where prediction is the goal, the result will be reported in terms of a prediction interval. A prediction interval is analogous to a CI but will be calculated in such a way to reflect the additional source of variation due to estimating future values. Estimating the incidence of VAP in 10 years’ time would be an example of inference with prediction as its goal.
After collecting the data, we use two main tools to describe it: graphs and summary statistics. A list of summary statistics is given in Table 7.1. In the analysis step, we will use a statistical method that can generally be classified as a univariate, bivariate, or multivariate4 method, according to the number of data elements involved in the research question. For example, estimating VAP involves one data element and would be considered a univariate analysis. Investigating the relationship of type of ventilation and VAP would be a bivariate analysis. Analyses involving more than two data elements are commonly referred to as “multivariate.” The choice of statistical method will depend on the inference goal, the number of data elements in the analysis (i.e., univariate, bivariate, and multivariate), and the data type(s).
There are five data types:
- Categorical nominal
- Categorical ordinal
- Binary
- Numerical discrete
- Numerical continuous
Data types are determined by the possible values a data element can have. Categorical data have values that are names or categories and not meaningful numbers. The categorical ordinal data type has values that can be ordered. The categorical nominal data type has values that have no natural ordering. Binary data have two values. Numerical discrete data when graphed are isolated points on a number line, while numerical continuous data have values that can be conceptually viewed as an interval on the number line. Table 7.3 gives examples of data types from the critical care literature. Table 7.4 lists the most common statistical methods with a brief description of each. For more details about statistical methods, see Motulsky (26), Armitage et al. (21), and D’Agostino et al. (27). Understanding how statistical methods are applied is crucial to understanding the “evidence” that EBM generates. As Gauch wrote, “Method precedes results; method affects results. Method matters” (28).
The sixth and final step of the research process—answering the research question—is of paramount importance. Researchers present results in the form of technical reports, abstracts, posters, oral or platform presentations, manuscripts, and books. Writing and presenting results clearly is an art form in and of itself. The research is not complete unless important patterns are summarized, the specific aims of a study are addressed, methods are described clearly, and the “story” of the data is told. For more on writing about the results of statistical analyses, see The Chicago Guide to Writing about Numbers and The Chicago Guide to Writing About Multivariate Analysis, both by Jane Miller (29,30).
TABLE 7.3 Variable Types | ||
![]() |
TABLE 7.4 Standard Statistical Techniques, Tests, and Tools | |
![]() |
Types of Research Studies
The statistical methods used to analyze research data depend on how the study that gave rise to that data was conducted. There are four basic types of study designs used in clinical research:
- Cross-sectional
- Case control
- Cohort
- Experimental (clinical trials)
For the following discussion about these study designs, we need to clarify some terms that are commonly used to describe medical research and epidemiologic findings. These may have different meanings depending on the context they are used in. These terms include sample, outcome, factor, exposure, treatment, and control.
- The term sample refers to the subjects being studied in the research. This reminds us that we are looking at a subset of a population of interest and that the purpose of the research is to apply what we find out about the sample to the population.
- The outcome of any research is handled in statistical analysis as the independent variable. The outcome is what we are primarily interested in understanding, treating, or preventing. In medical research, the outcome often relates to some disease or condition, with the simplest results being present or absent. In the following critical care–related examples, VAP (present or absent) will serve as the outcome. Defining and determining outcome (disease) status in clinical research and epidemiology is a large subject in itself. We will stipulate that there is an unambiguous and agreed upon way to measure the outcome status in the following discussion and examples.
- A factor is measured along with the outcome to determine if there is a correlation between them. In statistical parlance, factors are referred to as independent variables while the outcome is the dependent variable. The structure of relationships between factors and outcome is often quite complex, which, under the philosophy of scientific realism, reflects some underlying causal structure. In our example of VAP, factors that have positive association with VAP are considered to be risk factors. On the other hand, a protective factor has a negative association with the outcome (VAP). Note that we avoid directly asserting that an association between factor and outcome implies that the factor causes or prevents the outcome in deference to the old—and still true today—saying that correlation does not prove causation.
- With respect to a factor, exposure simply refers to the status (or level) of the factor in a particular subject. In the case of VAP (outcome), we would say that a patient in a coma is exposed to the risk factor of obtundation. Perhaps the best known example to both professional and lay public is lung cancer (outcome) with a risk factor of tobacco use to which a person is exposed if he or she is a smoker.
- In any clinical research, factors and outcomes are things that we seek to observe, measure, and record, but not influence. In contrast, a treatment (or intervention) is something that is actively controlled by the investigator. Conveniently, statistical terminology uses the term treatment in the same spirit as implied in clinical research. That is to say, treatment is an experimentally manipulated factor whose influence on the outcome we are interested in knowing.
In experimental studies, the term control group refers to subjects that do not receive any treatment. In case control studies (described further below), control subjects are those who do not have the outcome in question.
Table 7.5 summarizes the features of the four types of clinical research designs in their simplest forms, and they are each described below in more detail. We also include an example from current core literature in critical care medicine to illustrate the principles. It would be useful for readers to obtain copies of these papers and review them along with our explanations. Note that these studies often used more complex and involved methods of statistical analysis including secondary outcome measures; we will focus only on primary outcomes, factors, or treatments, and simple relationships between them. The order in which we present the design types reflects increasing cost, time, and potential risk or inconvenience for patients. Thus, observational and retrospective studies (cross-sectional and case control) are discussed first, followed by prospective cohort studies and clinical trials.
TABLE 7.5 Comparison of Four Study Design Types |
![]() |
Cross-Sectional
A cross-sectional study does not involve the passage of time. A single sample is selected without regard to outcome or risk factor exposure status. Information on outcome and exposure status is determined with data collected at a single time point. The status of the outcome can be compared between exposed and unexposed groups. It is important to understand that even though we may define one attribute as the “outcome” and others as “factors,” there is no logical way to determine anything other than the current relationship between them. Without additional information, there is no valid way to infer even the temporal order of factor levels and outcome status, let alone any causal connection.
Our example, “Prevention of ventilator-associated pneumonia: current practice in Canadian intensive care units”, comes from the Journal of Critical Care (31). In this study, Heyland et al. wanted to know the current status of VAP prevention strategies in Canadian ICUs prior to disseminating a new set of clinical guidelines. There is not really a defined “outcome” in this study; rather, a number of factors of interest were simultaneously measured. Such a study design is sometimes called a “snapshot.” In this case, dietitians recruited by the investigators directly observed VAP prevention strategies in ICUs throughout Canada on a single date (April 18, 2001). Since the 66 observers recorded data for every patient currently in their assigned ICU, the unit of analysis was the single patient (N = 702). The investigators followed up the initial observations by manually abstracting the medical chart entries from April 18, 2001, for each of the 702 patients. Finally, surveys were filled out by 66 ICU directors that asked questions about the regular practices relating to VAP prevention on their unit; thus, the unit of observation for this part of the study was an individual ICU (N = 66). These three methods are good examples of the various ways that cross-sectional data can be gathered and aggregated.
From the many results presented in this paper, we present a few examples of the sorts of statistics that are typically reported in cross-sectional studies. From the survey of ICU directors, we get results like university affiliation (29/66 = 44%) and number of beds (mean = 13.9). From the observations and chart abstractions, authors report patient gender (women = 299/702 for 43% and men = 403/702 for 57%), intubated and ventilated (403/702 for 57%), and patient age (mean = 63.5 years). As for VAP prevention strategies from direct observation, we have elevation of head of bed (mean = 30 degrees) and kinetic bed therapy (22/702 for 3%). From the survey of unit directors, 61 of 66 (92%) responded that they never used special endotracheal tubes that allowed subglottic secretion drainage, and none stated that they used prophylactic antibiotics. This example is typical of cross-sectional studies in that only descriptive statistics (as just described) are necessary and usually suffice. Additional methods that might be used in analyzing a cross-sectional study such as this one would be simple statistics to quantify relationships (correlation) between measured factors (none was reported in the paper). For example, at the ICU level, authors could have used survey results to look for a relationship between university affiliation and VAP strategies such as subglottic secretion drainage. This would be done by cross-tabulating the two variables into a 2 × 2 table in this case. The appropriate statistical method to test for a significant relationship between two categoric variables is a chi-square test (or the Fisher exact test in the case of sparse cell sizes).
Case Control
In a case control study, the investigator compares individuals who have a positive outcome status (the cases) and individuals who do not have the outcome (the controls). In the simplest implementation of the case control method, one or more control patients (outcome negative) are chosen for each case (outcome positive). When the outcome being studied is relatively rare, there are often more available candidates for controls than for cases in the sample available for study. A subsample of available controls is usually selected to match the cases on characteristics (factors) that might be related to the outcome but are not of primary interest to the research question. For example, it is common to match on gender and age by finding a single control subject with same gender and similar age for each case. This is called 1:1 (control:case) matching. When there are many more outcome-negative (control) candidates, investigators may use 2:1, 3:1, or greater (control:case) matching ratios while still maintaining similarity between each case and its controls (e.g., gender and age).
Investigators look backward in time (i.e., retrospectively) to collect information about risk or protective factors for both cases and controls by examining past records, interviewing the subject, or in some other way. Unlike with a cross-sectional study, we can get an indication of whether the factor status predated the development of the outcome by asking the questions carefully. However, case control studies are subject to well-known bias in assessing presence and timing of risk/protective factors. It is very important to understand that in a case control study, the outcome frequency is fixed in the design, which means that we cannot directly estimate risk of the outcome. We can estimate relative risk of the outcome between different status levels of the factors by calculating the odds ratio between cases and controls for each factor. This estimate of relative risk will be biased, depending on the actual prevalence of the outcome in the population of interest and the relative numbers of cases and controls. For example, in a 1:1 case control study, the outcome frequency is, by definition, 50%. If the actual frequency of the outcome in the population is less than 10%, the odds ratios will severely overestimate the relative risk increase or reduction. The raw odds ratios may be corrected for this bias to form a better estimate of relative risk, though this is rarely done in practice.
An example of a case control study looking at factors associated with VAP, “Epidemiology and outcomes of ventilator-associated pneumonia in a large US database”, can be found in Chest (32). The investigators used information from a large (750,000/year) database of inpatient hospital (N = 100) admission abstracts (MediQaul-Profile Database) for 18 months beginning in January of 1998. They first identified 9,080 patients having at least 1 day of mechanical ventilation in the ICU during their hospitalization without an admission diagnosis of pneumonia. Of these, 842 (9.3%) developed pneumonia after initiation of ventilation, thus meeting criteria for VAP. From one to three controls (VAP negative, N = 2,243) were selected to match each case (VAP positive, N = 842) on duration of ventilation, severity of illness on admission, and age. It is important to note that after this step, none of the matched factors can be meaningfully evaluated because their distributions were forced to be in direct proportion to each other during the process of matching.
Investigators calculated odds ratios between cases and controls for several factors that might alter risk of developing VAP in practice; these included gender, race, obtundation, and type of ICU admission (trauma, medical, surgical) among others. As an example of how such results are evaluated, the numbers of males and females in VAP-positive cases were 540 (64%) and 302 (36%) and for VAP-negative controls were 4,262 (52%) and 3,976 (48%), respectively. The odds ratio male:female is calculated by (540)(3,976)/(302)(4,262) = 1.67. This can be interpreted by stating that the odds of VAP in males are 67% greater than for females, which can be used as an estimate of the relative risk of VAP in males compared with females. Note that in the original sample of patients, the frequency of VAP was 9.3%, whereas in the case:control sample used to estimate relative risk in males, it was 27%. Thus, the estimate of relative risk should be revised downward by methods that are beyond the scope of this chapter.
Cohort
A cohort is a group of individuals. The term comes from Roman military tradition where legions of the army were divided into 10 cohorts, and each in turn divided into centuries. These cohorts “march forward together” in time. In a typical prospective cohort study, investigators follow subjects after study inception to collect information about development of the outcome (disease). In a retrospective study, outcome status is determined from records produced prior to beginning the study. The cohorts are articulated based on information about risk factors predating the outcome determination. In both types, initially outcome-negative (disease-free) subjects are divided into groups (cohorts) based on exposure status with respect to a risk factor. Cumulative incidence (the proportion that develops the outcome in a specified length of time) can be computed and compared for the exposed and unexposed cohorts. The main difference between prospective and retrospective cohort studies is whether the time period in question is before (retrospective) or after (prospective) the study is begun. In terms of bias and error in measuring outcome and risk factors, the retrospective cohort design is more problematic because we must rely entirely on historical records to know that subjects were initially outcome negative, what their risk factor status was, and the subsequent outcome status over time.
Despite logistical difficulty and expense, prospective cohort studies are very attractive to investigators because they allow direct estimation of absolute risk for the outcome of interest as well as differences in outcome based on various risk (or protective) factors (relative risk). In general, outcomes that develop quickly are easier to evaluate prospectively since the study will be finished sooner, with less chance for subjects to drop out or be lost to follow-up. Critical care medicine lends itself quite well to prospective cohort studies for this reason.
An example of a multinational and quite complex cohort study, “Incidence, risk factors, and outcome of ventilator-associated pneumonia,” comes from the Journal of Critical Care (25) and reports results of a study encompassing 361 ICUs in 20 countries. The cohort in question included 2,897 consecutive ICU patients who were mechanically ventilated for more than 2 days, with reason for admission not being pneumonia. These patients were a subset from a larger (N = 5,183) and already completed prospective study. Even though the authors analyzed existing data, they properly labeled their study as prospective because the patients were entered into the original study at time of admission to ICU, and all information was sequentially recorded in a database as the research progressed.
The outcome of VAP was strictly defined using Centers for Disease Control and Prevention (CDC) criteria prior to study inception and measured for each patient on a daily basis as yes/no. Though it may seem a trivial point, it is important to note that all patients had a VAP status of “no” on the first day of their ICU stay. Multiple baseline and clinical factors were measured. In the results section, the authors first considered the entire sample as a single cohort and reported the incidence of VAP as 439/2,897 (15%). There is not a meaningful hypothesis for the simple question of VAP incidence in the whole cohort, and we are instead making an estimate of VAP incidence in the population. Because of the large sample size, the authors were able to place 95% confidence intervals on this estimate of 14% to 16%.
In reading the rest of the results, it is helpful to think of each separate factor as dividing the entire study group (N = 2,897) into “subcohorts.” For example, gender would give two cohorts (male = 1,809 and female = 1,088) for which VAP incidence was 293 (16%) for the males and 142 (13%) for the females. When considering the factor of problem type (medical = 1,911 and surgical = 986), the VAP incidence was 322 (17%) for medical and 117 (12%) for surgical. The null hypothesis in each of these “subcohort” studies is that VAP incidence is equal between the factor levels (male/female and medical/surgical). In these two examples, the null hypothesis was rejected for each of the factors (gender p = 0.02 and problem p < 0.001). Below, we give an example of a randomized trial with a sample size in each of three groups of about 130. The percentages of VAP are similar in magnitude as are the intergroup differences (roughly 18%, 10%, and 13%). However, because of the smaller sample size, the results do not reach statistical significance (at the 0.05 level).
There are two problems with doing multiple separate tests for simultaneously measured factors in a big study such as this one. First, the measured factors quite probably interact with each other in a complex way in their combined effect on VAP development. Thus, in any single (univariate) analysis such as with gender and VAP, the calculated relationship may be confounded with one or more other factors, and therefore biased away from the actual effect. Second, it is problematic to look at the same set of data repeatedly using different factor combinations because by sheer chance, 1 in 20 such tests will be “significant” at the 0.05 level. The optimal solution to both these problems is to perform multivariable regression analysis where the joint effect of all variables is tested simultaneously. The authors of this paper did such analyses, though the details are beyond the scope of this chapter. Suffice it to say, gender showed no significant effect on VAP after accounting for all other factors while problem still did. Finally, it is important to note that multivariable analysis cannot rescue a study from being confounded by unmeasured factors. The only certain way to avoid confounding is through the random assignment of factor levels prior to measuring the outcome (i.e., an experimental study).
Experimental (Clinical Trial)
In an experimental study (called a clinical trial in medical research), the investigator selects a sample of subjects with the same outcome status and randomly assigns each to a treatment (or intervention) condition. Subjects are followed in time, and the status of the outcome is measured and compared between the treatment groups; experiments and clinical trials are, by definition, prospective. As described above, the term control group is used for subjects that do not receive any treatment or intervention. Sometimes patients who are given “standard” treatment are said to be in the control group. A randomized experiment is the only way to definitively establish causality by empirical means. Similarly, for testing medical treatments and interventions, randomized trials are the only sure way to determine clinical efficacy. Both necessary and sufficient conditions to establish causality between a factor or treatment and outcome status are met in a randomized trial. These include unambiguous temporal association of cause before effect and elimination of any potential confounding factors (measured or unmeasured) that might affect the outcome. This helps to explain what may seem to be near-worship of the “prospective randomized clinical trial” in many discussions about medical research.
A good example of a randomized clinical trial comes from the American Journal of Respiratory and Critical Care Medicine (33), entitled, “Oral decontamination with chlorhexidine reduces the incidence of ventilator-associated pneumonia,” which nicely describes the research question and the result. Koeman et al. wanted to determine if two oral decontamination regimens would reduce incidence of VAP in intubated patients. Performing a classic double-blinded, placebo-controlled clinical trial in which 385 eligible patients were randomized to three treatment arms: chlorhexidine 2% (CHX), chlorhexidine 2% and colistin 2% (CHX/COL), and water (PLAC). During the ICU stay, all patients got mouth swabbing at identical intervals with the type of solution unknown to those caring for the patients. The primary outcome was carefully defined and evaluated using chart abstraction by a team of physicians who did not know the treatment assignment. These two design elements satisfy the definition of a double-blinded study because neither patients and providers nor those determining the outcome knew what the treatment assignments were. The first table in the results (their Table 1) shows baseline characteristics grouped by treatment assignment. Such a table is always included in any complete report of a randomized trial. The baseline characteristics are measured and presented because they might also influence the outcome (VAP). One is reassured to see relative equality of the baseline characteristics because it shows us that “randomization works.” Note that the true power of randomized treatment assignment lies in the fact that we know that any other factors or characteristics that were not anticipated and/or measured will, by definition, also be equally distributed between the treatment arms. Some might rightfully observe that such baseline characteristic tables are superfluous to the core logic of a randomized trial. We carry on presenting them because they are reassuring and otherwise informative.
The outcome can be most simply expressed as an incidence of VAP during the ICU stay with individuals having a yes/no answer. When tabulated, the results (VAP/total) were PLAC = 23/130 (17.7%), CHX = 13/127 (10.2%), and CHX/COL = 16/128 (12.5%). The trialists performed sophisticated statistical techniques using days to onset of VAP and survival analysis, as well as interim analyses to allow early termination of the trial. These are beyond the scope of this brief chapter. The null hypothesis in this study is that the incidence (hazard in survival analysis) of VAP was identical among all three treatment arms. The alternate hypothesis is that one or more of the treatment groups had significantly different incidence (hazard) of VAP. A chi-square test on the simple incidence of VAP between the treatment groups gives a p-value at 0.20, which indicates that the null hypothesis cannot be rejected. However, using survival analysis, authors found that both CHX (p = 0.012) and CHX/COL (p = 0.030) reduced the hazard of VAP compared with PLAC.
This is a good example of how the type of outcome variable analyzed affects the power of a study to detect differences. For simple counting of VAP incidence, the outcome variable is dichotomous (yes/no), while for survival analysis, the outcome variable is quantitative (days to development of VAP). In general, outcomes defined and measured numerically have “more information,” and thus greater power to detect small differences, than categoric or dichotomous ones. In the paper cited above, the authors did not report the results of the simple chi-square test, which failed to reject the null hypothesis. We can speculate that if the chi-square statistic on simple VAP incidence had shown significant differences, the authors would have reported it. Finally, we recall the results of our cohort study example with similar VAP percentage differences but much larger sample size. In that study, the achieved p-values were much lower, reflecting the greater power of large samples to demonstrate small effects.
CRITICAL READING OF THE MEDICAL LITERATURE
Articles in the medical literature generally follow a prescribed structure consisting of the following components: (a) title, (b) author list, (c) keywords, (d) funding source, (e) abstract, (f) objective and hypothesis, (g) background, (h) methods (includes study design, measures, and data analysis), (i) description of sample, (j) presentation of findings and results, (k) discussion and conclusions, and (l) references.
Statistical aspects permeate a large number of articles in the medical literature. Miller (30) describes the similarity of writing about statistical analysis to the presentation of a legal argument. She writes:
In the opening statement, a lawyer raises the major questions to be addressed during the trial and gives a general introduction to the characters and events in question. To build a compelling case, he then presents specific facts collected and analyzed using standard methods of inquiry. If innovative or unusual methods were used, he introduces experts to describe and justify those techniques. He presents individual facts, then ties them to other evidence to demonstrate patterns or themes. He may submit exhibits such as diagrams or physical evidence to supplement or clarify the facts. He cites previous cases that have established precedents and standards in the field and discusses how they do or do not apply to the current case. Finally, in the closing argument he summarizes conclusions based on the complete body of evidence, restating the critical points but with far less detail than in the evidence portion of the trial.

Full access? Get Clinical Tree
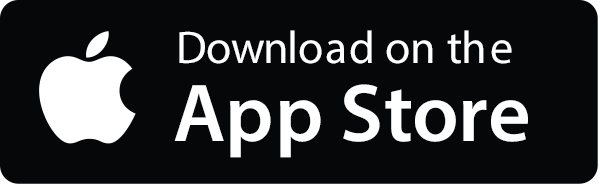
