The statistical procedures developed in Chapters 2 to 4 are appropriate for analyzing the results of experiments in which the variable of interest is measured on an interval scale, such as blood pressure, urine production, or length of hospital stay. Much of the information physicians, nurses, other health professionals, and medical scientists use cannot be measured on interval scales. For example, an individual may be male or female, dead or alive, or Caucasian, African American, Hispanic, or Asian. These variables are measured on nominal scales, in which there is no arithmetic relationship between the different classifications. We now develop the statistical tools necessary to describe and analyze such information.
It is easy to describe things measured on a nominal scale: simply count the number of patients or experimental subjects with each condition and (perhaps) compute the corresponding percentages.
For example, John Song and colleagues* wanted to study whether or not providing homeless people with personal counseling on end-of-life care and advanced directives would lead more of them to complete such directives. (This question had been studied among insured general adult populations, but not among the homeless, who have more health problems and less access to stable health care relationships.) To investigate this question, they recruited people at emergency night shelters, 24-hour shelters, a day program and treatment programs. They conducted an experiment in which volunteers were randomly assigned to either receive written material on advance directives or invited to attend a 1-hour in-person counseling session on advance directives. The outcome of the study was whether the people returned a completed advance directive within 3 months. Among the 262 people who participated in the study 37.9% of the people who received the in-person counseling returned the advanced directives within 3 months, compared with 12.8% of the people who were just given written instructions. Is this difference likely to be a real effect of the counseling or simply a reflection of random sampling variation?
To answer this and other questions about nominal data, we must first invent a way to estimate the precision with which percentages based on limited samples approximate the true rates that would be observed if we could examine the entire population, in this case, all homeless people. We will use these estimates to construct statistical procedures to test hypotheses.
Before we can quantify the certainty of our descriptions of a population on the basis of a limited sample, we need to know how to describe the population itself. Since we have already visited Mars and met all 200 Martians (in Chapter 2), we will continue to use them to develop ways to describe populations. In addition to measuring the Martians’ heights, we noted that 50 of them were left-footed and the remaining 150 were right-footed. Figure 5-1 shows the entire population of Mars divided according to footedness. The first way in which we can describe this population is by giving the proportion p of Martians who are in each class. In this case, pleft = 50/200 = 0.25 and pright = 150/250 = 0.75. Since there are only two possible classes, notice that pright = 1 – pleft. Thus, whenever there are only two possible classes and they are mutually exclusive, we can completely describe the division in the population with the single parameter p, the proportion of members with one of the attributes. The proportion of the population with the other attribute is always 1 – p.
Note that p also is the probability of drawing a left-footed Martian if one selects one member of the population at random.
Thus p plays a role exactly analogous to that played by the population mean μ in Chapter 2. To see why, suppose we associate the value X = 1 with each left-footed Martian and a value of X = 0 with each right-footed Martian. The mean value of X for the population is
This idea can be generalized quite easily using a few equations. Suppose M members of a population of N individuals have some attribute and the remaining N – M members of the population do not. Associate a value of X = 1 with the population members having the attribute and a value of X = 0 with the others. The mean of the resulting collection of numbers is
Since we can compute a mean in this manner, why not compute a standard deviation in order to describe variability in the population? Even though there are only two possibilities, X = 1, and X = 0, the amount of variability will differ, depending on the value of p. Figure 5-2 shows three more populations of 200 individuals each. In Figure 5-2A only 10 of the individuals are left-footed; it exhibits less variability than the population shown in Figure 5-1. Figure 5-2B shows the extreme case in which half the members of the population fall into each of the two classes; the variability is greatest. Figure 5-2C shows the other extreme; all the members fall into one of the two classes, and there is no variability at all.
Figure 5-2.

This figure illustrates three different populations, each containing 200 members but with different proportions of left-footed members. The standard deviation, quantifies the variability in the population. (A) When most of the members fall in one class, σ is a small value, 0.2, indicating relatively little variability. (B) In contrast, if half the members fall into each class, σ reaches its maximum value of .5, indicating the maximum possible variability. (C) At the other extreme, if all members fall into the same class, there is no variability at all and σ = 0.
To quantify this subjective impression, we compute the standard deviation of the 1s and 0s associated with each member of the population when we computed the mean. By definition, the population standard deviation is
This equation for the population standard deviation produces quantitative results that agree with the qualitative impressions we developed from Figures 5-1 and 5-2. As Figure 5-3 shows, σ = 0 when p = 0 or p = 1, that is, when all members of the population either do or do not have the attribute, and s is maximized when p = .5, that is, when any given member of the population is as likely to have the attribute as not.
Figure 5-3.

The relationship between the standard deviation of a population divided into two categories varies with p, the proportion of members in one of the categories. There is no variation if all members are in one category or the other (so σ = 0 when p = 0 or 1) and maximum variability when a given member is equally likely to fall in one class or the other (σ = 0.5 when p = 0.5).
Since σ depends only on p, it really does not contain any additional information (in contrast to the mean and standard deviation of a normally distributed variable, where μ and σ provide two independent pieces of information). It will be most useful in computing a standard error associated with estimates of p based on samples drawn at random from populations such as those shown in Figures 5-1 or 5-2.
Of course, if we could observe all members of a population, there would not be any statistical question. In fact, all we ever see is a limited, hopefully representative, sample drawn from that population. How accurately does the proportion of members of a sample with an attribute reflect the proportion of individuals in the population with that attribute? To answer this question, we do a sampling experiment, just as we did in Chapter 2 when we asked how well the sample mean estimated the population mean.
Suppose we select 10 Martians at random from the entire population of 200 Martians. Figure 5-4A shows which Martians were drawn; Figure 5-4B shows all the information the investigators who drew the sample would have. Half the Martians in the sample are left-footed and half are right-footed. Given only this information, one would probably report that the proportion of left-footed Martians is 0.5%, or 50%.
Figure 5-4.

Panel A shows one random sample of 10 Martians selected from the population in Figure 5-1; panel B shows what the investigator would see. Since this sample included five left-footed Martians and five right-footed Martians, the investigator would estimate the proportion of left-footed Martians to be left = 5/10 = .5, where the circumflex denotes an estimate.
Of course, there is nothing special about this sample, and one of the four other random samples shown in Figure 5-5 could just as well have been drawn, in which case the investigator would have reported that the proportion of left-footed Martians was 30%, 30%, 10%, or 20%, depending on which random sample happened to be drawn. In each case, we have computed an estimate of the population proportion p based on a sample. Denote this estimate . Like the sample mean, the possible values of
depend on both the nature of the underlying population and the specific sample that is drawn. Figure 5-6 shows the five values of
computed from the specific samples in Figures 5-4 and 5-5 together with the results of drawing another 20 random samples of 10 Martians each. Now we change our focus from the population of Martians to the population of all values of
computed from random samples of 10 Martians each. There are more than 1016 such samples with their corresponding estimates
of the value of p for the population of Martians.
Figure 5-6.

There will be a distribution of estimates of the proportion of left-footed Martians left depending on which random sample the investigator happens to draw. This figure shows the five specific random samples drawn in Figures 5-4 and 5-5 together with 20 more random samples of 10 Martians each. The mean of the 25 estimates of p and the standard deviation of these estimates are also shown. The standard deviation of this distribution is the standard error of the estimate of the proportion
; it quantifies the precision with which
estimates p.
The mean estimate of for the 25 samples of 10 Martians each shown in Figure 5-6 is 30%, which is remarkably close to the true proportion of left-footed Martians in the population (25% or 0.25). There is some variation in the estimates. To quantify the variability in the possible values of
, we compute the standard deviation of values of
computed from random samples of 10 Martians each. In this case, it is about 14% or 0.14. This number describes the variability in the population of all possible values of the proportion of left-footed Martians computed from random samples of 10 Martians each.
Does this sound familiar? It should. It is just like the standard error of the mean. Therefore, we define the standard error of the proportion to be the standard deviation of the population of all possible values of the proportion computed from samples of a given size. Just as with the standard error of the mean
in which is the standard error of the proportion, σ is the standard deviation of the population from which the sample was drawn, and n is the sample size. Since
We estimate the standard error from a sample by replacing the true value of p in this equation with our estimate obtained from the random sample. Thus,
The standard error is a very useful way to describe the uncertainty in the estimate of the proportion of a population with a given attribute because the central-limit theorem (Chapter 2) also leads to the conclusion that the distribution of is approximately normal, with mean p and standard deviation
for large enough sample sizes. On the other hand, this approximation fails for values of p near 0 or 1 or when the sample size n is small. When can you use the normal distribution? Statisticians have shown that it is adequate when n
and n(1 −
) both exceed about 5.* Recall that about 95% of all members of a normally distributed population fall within 2 standard deviations of the mean. When the distribution of
approximates the normal distribution, we can assert, with about 95% confidence, that the true proportion of population members with the attribute of interest p lies within 2
of
These results provide a framework within which to consider the question we posed earlier in this chapter regarding whether in-person counseling led to higher levels of completing end-of-life advance directives among homeless people. Of the 145 people who received in-person counseling 37.9% completed the advance directives and 12.8% of the 117 people who just received written instructions did so. The standard errors of these proportions are
for written instructions. Given that there was a 25.1% difference in the rate that people returned the advance directive, it seems likely that the counseling had an effect beyond just random sampling variation.
Before moving on, we should pause to list explicitly the assumptions that underlie this approach. We have been analyzing what statisticians call independent Bernoulli trials, in which
- Each individual trial has two mutually exclusive outcomes.
- The probability p of a given outcome remains constant.
- All the trials are independent.
- Each member of the population belongs to one of two classes.
- Each member of the sample is selected independently of all other members.
*When the sample size is too small to use the normal approximation, you need to solve the problem exactly using the binomial distribution (or use a table of exact values). For a discussion of the binomial distribution, see Zar JH. Dichotomous variables. Biostatistical Analysis, 5th ed. Upper Saddle River, NJ: Prentice Hall; 2010:chap 24.
In Chapter 4, the sample mean and standard error of the mean provided the basis for constructing the t test to quantify how compatible observations were with the null hypothesis. We defined the t statistic as
The role of is analogous to that of the sample mean in Chapters 2 and 4, and we have also derived an expression for the standard error of
. We now use the observed proportion of individuals with a given attribute and its standard error to construct a test statistic analogous to t to test the hypothesis that the two samples were drawn from populations containing the same proportion of individuals with a given attribute.
Let 1 and
2 be the observed proportions of individuals with the attribute of interest in the two samples. The standard error is the standard deviation of the population of all possible values of
associated with samples of a given size, and since variances of differences add, the standard error of the difference in proportions is
z replaces t because this ratio is approximately normally distributed for large enough sample sizes,* and it is customary to denote a normally distributed variable with the letter z.
Just as it was possible to improve the sensitivity of the t test by pooling the observations in the two sample groups to estimate the population variance, it is possible to increase the sensitivity of the z test for proportions by pooling the information from the two samples to obtain a single estimate of the population standard deviation s. Specifically, if the null hypothesis that the two samples were drawn from the same population is true, 1 = m1n1 and
2 = m2n2, in which m1 and m2 are the number of individuals in each sample with the attribute of interest, are both estimates of the same population proportion p. In this case, we could consider all the individuals drawn as a single sample of size n1 + n2 containing a total of m1 + m2 individuals with the attribute and use this single pooled sample to estimate
:
Therefore, our test statistic, based on a pooled estimate of the uncertainty in the population proportion, is
Like the t statistic, z will have a range of possible values depending on which random samples happen to be drawn to compute 1 and
2, even if both samples were drawn from the same population. If z is sufficiently “big” we will conclude that the data are inconsistent with this null hypothesis and assert that there is a difference in the proportions. This argument is exactly analogous to that used to define the critical values of the t for rejecting the hypothesis of no difference. The only change is that in this case we use the standard normal distribution (Fig. 2-5) to define the cutoff values. In fact, the standard normal distribution and the t distribution with an infinite number of degrees of freedom are identical, so we can get the critical values for 5 or 1% confidence levels from the last line in Table 4-1. This table shows that there is less than a 5% chance of z being beyond −1.96 or +1.96 and less than a 1% chance of z being beyond −2.58 or +2.58 when, in fact, the two samples were drawn from the same population.
*The criterion for a large sample is the same as in the last section, namely that n and (1 −
) both exceed about 5 for both samples. When this is not the case, one should use the Fisher exact test discussed later in this chapter.
The standard normal distribution only approximates the actual distribution of the z test statistic in a way that yields P values that are always smaller than they should be. Thus, the results are biased toward concluding that the treatment had an effect when the evidence does not support such a conclusion. The mathematical reason for this problem has to do with the fact that the z test statistic can only take on discrete values, whereas the theoretical standard normal distribution is continuous. To obtain values of the z test statistic which are more compatible with the theoretical standard normal distribution statisticians have introduced the Yates correction (or continuity correction), in which the expression for z is modified to become
This adjustment slightly reduces the value of z associated with the data and compensates for the mathematical problem just described.
We can now formally test the null hypothesis that counseling and just giving homeless people written instructions on end-of-life care leads to the same rate of completing advance directives. (Note that we can say “leads” or “causes” rather than just “is associated with” because this is a randomized experiment, not an observational study.) Since 55 (37.9% of 145) people who received in-person counseling completed the advance directives and 15 (12.8% of 117) people who just received written instructions did so,
Since n for the two samples, .267 · 145 = 38.7 and .267 · 117 = 31.2 both exceed 5, we can use the test described in the last section.* Our z test statistic is therefore
Including the Yates correction, it is
Note that the Yates correction reduced the value of the z test statistic. (Since the sample sizes are reasonably large, the effect was small.) The value of the z test static, 4.443, exceeds 3.2905, the value that defines the most extreme 1% of the normal distribution (from Table 4-1), so we reject the null hypothesis of no difference and conclude that the in-person counseling significantly increased the rate at which homeless people returned the advance directives.
* n(1 − ) also exceeds 5 for both samples because
< .5, so n
< n(1 −
).
The methods we just developed based on the z statistic are perfectly adequate for testing hypotheses when there are only two possible attributes or outcomes of interest. The z statistic plays a role analogous to the t test for data measured on an interval scale. There are many situations, however, where there are more than two samples to be compared or more than two possible outcomes. To do this, we need to develop a testing procedure, analogous to analysis of variance, which is more flexible than the z test just described. While the following approach may seem quite different from the one we just used to design the z test for proportions, it is essentially the same.
To keep things simple, we begin with the problem we just solved, assessing the effectiveness of in-person counseling of homeless people to prepare advance directives. In the last section we based the analysis on the proportion of people in each of the two treatment groups (in-person counseling or written materials). Now we change our emphasis slightly and base the analysis on the number of people in each group who did and did not file advance directives. Since the procedure we will develop does not require assuming anything about the nature of the parameters of the population from which the samples were drawn, it is called a nonparametric method.
Table 5-1 presents the data from this experiment in terms of the number of people in each treatment group who did and did not file advance directives. This table is called a 2 × 2 contingency table. Most of the people in the study fall along the diagonal in this table, suggesting an association between the experimental intervention and whether or not the person filed and advance directive. Table 5-2 shows what the experimental results might have looked like if the experimental intervention had no effect on the results, if the null hypothesis of no effect was true. It also shows the total number of people who received each intervention as well as the total who did and did not file advance directives. (The sums of the rows and columns are the same as in Table 5-1.) In Table 5-2, fewer people in both intervention groups filed advance directives than did not; the differences in the absolute numbers occur because more people were randomized into the counseling group than the written instructions group. In contrast to Table 5-1, there does not seem to be a relationship between the intervention and whether people filed advance directives.
Number of People | |||
---|---|---|---|
Intervention | Filed Advance Directive | Did Not File Advance Directive | Total in Intervention Group |
In-person counseling | 55 | 90 | 145 |
Written instructions | 15 | 102 | 117 |
Total | 70 | 192 | 262 |
Number of People | |||
---|---|---|---|
Intervention | Filed Advance Directive | Did Not File Advance Directive | Total in Intervention Group |
In-person counseling | 38.74 | 106.26 | 145 |
Written instructions | 31.26 | 85.74 | 117 |
Total | 70.00 | 192.00 | 262 |
To understand why most people have this subjective impression, let us examine where the numbers in Table 5-2 came from. Of all the 262 people in the study, 70, or 70/262 = 26.7%, filed advance directives and 192, or 192/262 = 73.3%, did not. Now, let us assume that the null hypothesis is true and that the intervention had no effect on the likelihood that a person would file an advance directive. In this case, we would expect 26.7% of the 145 people who received in-person counseling to file advance directives (38.74 people) and 26.7% of the 117 people who just received written materials (31.26) to file advance directives. We would expect the remaining 73.3% of people in each group to not have filed advance directives.* (We compute the expected frequencies to two decimal places to ensure accurate results in the computation of the χ2 test statistic below.) Thus, Table 5-2 shows how we would expect the data to look if 145 people received in-person counseling and 117 received written materials and 70 of them were destined to file advance directives regardless of which intervention they received. Compare Tables 5-1 and 5-2. Do they seem similar? Not really; the actual pattern of observations seems quite different from what we expected if the intervention had no effect.
The next step in designing a statistical procedure to test the hypothesis that the pattern of observations is due to random sampling rather than the intervention is to reduce this subjective impression to a single number, a test statistic, such as F, t, or z, so that we can reject the null hypothesis of no effect when this statistic is “big.”
Before constructing this test statistic, however, let us consider another example. Hypothermia is a problem for extremely low birth weight infants. To investigate whether wrapping these infants in polyethylene bags in the delivery room and while they are being transferred to the neonatal intensive care unit affected survival, Patrick Carroll and colleaguesch5.fn6 reviewed medical records and located 70 infants who were kept warm with polyethylene bags and 70 infants who were kept warm with traditional methods. In an effort to avoid problems created by confounding variables in this observational study, they matched the infants according to birth weight, gestational age, and gender. They found that the infants wrapped in the polyethylene bags had statistically significantly higher skin temperatures, by an average of 1° C (see Prob. 4-2). The more important question was whether or not there was a mortality benefit.
Table 5-3 shows the results of this study, presented in the same format as Table 5-1. Table 5-4 shows the expected pattern of observations if the null hypothesis that the warming treatment had no effect on mortality was true. Out of the 140 infants, 124, or 124/140 = 88.6%, lived. If the warming treatment had no effect on survival, we would expect 88.6% of the 70 infants in each treatment group, 62 to live and the remaining 8 in each group to die. Comparing the observed mortality pattern in Table 5-3 with the expected pattern if the null hypothesis of no effect was true shows little difference, suggesting that there is no association between the kind of warming treatment and mortality.
*We could also have computed the estimated numbers by multiplying the number of people who did or did not file advance directives times the fraction of all the 262 people in the study, 145, or 145/262 = 55.3%, received in-person counseling and 117, or 117/262 = 44.7%, received written materials. The result would be the same.
†Carroll PD, Nanketvis CA, Giannone PJ, Cordero L. Use of polyethylene bags in extremely low birth weight infant resuscitation for the prevention of hyperthermia. J Reprod Med. 2010;55:9–13.
Now we are ready to design our test statistic. It should describe, with a single number, how much the observed frequencies in each cell in the table differ from the frequencies we would expect if there is no relationship between the treatments and the outcomes that define the rows and columns of the table. In addition, it should allow for the fact that if we expect a large number of people to fall in a given cell, a difference of one person between the expected and observed frequencies is less important than in cases where we expect only a few people to fall in the cell.
We define the test statistic χ2 (the square of the Greek letter chi) as
The sum is calculated by adding the results for all cells in the contingency table. The equivalent mathematical statement is
in which O is the observed number of individuals (frequency) in a given cell, E is the expected number of individuals (frequency) in that cell, and the sum is over all the cells in the contingency table. Note that if the observed frequencies are similar to the expected frequencies, χ2 will be a small number and if the observed and expected frequencies differ, χ2 will be a big number.
We can now use the information in Tables 5-1 and 5-2 to compute the χ2 statistic associated with the data on counseling and filing advanced directives. Table 5-1 gives the observed frequencies, and Table 5-2 gives the expected frequencies. Thus,
To begin getting a feeling of whether or not 20.854 is “big,” let us compute χ2 for the data on warming technique for extreme low birth weight infants and mortality using the observed and expected counts in Tables 5-3 and 5-4:
which is pretty small, in agreement with our intuitive impression that the observed and expected frequencies are quite similar. (Of course, it is also in agreement with our earlier analysis of the same data using the z statistic in the last section.) In fact, it is possible to show that χ2 = when there are only two samples and two possible outcomes.
Like all test statistics, χ2 can take on a range of values even when there is no relationship between the treatments and outcomes because of the effects of random sampling. Figure 5-7 shows the distribution of possible values for χ2 computed from data in 2 × 2 contingency tables such as those in Tables 5-1 or 5-3. It shows that when the hypothesis of no relationship between the rows and columns of the table is true, χ2 would be expected to exceed 3.841 only 5% of the time.
Figure 5-7.

The χ2 distribution with 1 degree of freedom. The shaded area denotes the biggest 5% of possible values of the χ2 test statistic when there is no relationship between the treatments and observations.

Full access? Get Clinical Tree
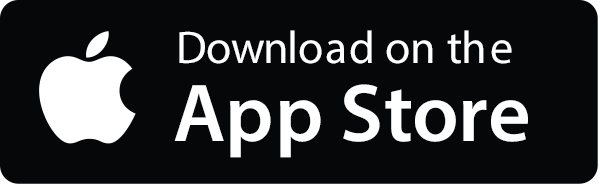

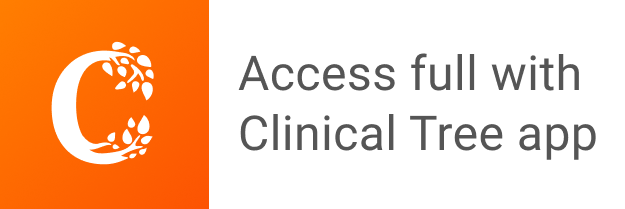