Genomic Basis of Perioperative Medicine
Kevin Friede
Joseph P. Mathew
Mihai V. Podgoreanu
Key Points








Related Matter
Host Response to Injury
Scientific Rationale for Perioperative Genomic Medicine
Human biologic diversity involves interindividual variability in morphology, behavior, physiology, development, susceptibility to disease, and response to stressful stimuli and drug therapy (i.e., phenotypes). This phenotypic variation is determined, at least in part, by differences in the specific genetic makeup (i.e., genotype) of an individual. In 2003, the 50th anniversary of Watson and Crick’s description of the DNA double-helix structure also marked the completion of the Human Genome Project.1 This major accomplishment provides the discipline of genomics with basic resources to study the functions and interactions of all genes in a systematic fashion, including their interaction with environmental factors, and translate the findings into clinical and societal benefits. Functional genomics employs large-scale experimental methodologies and statistical analyses to investigate the regulation of gene expression in response to physiologic, pharmacologic, and pathologic changes. It also uses genetic information from clinical studies to examine the impact of genetic variability on disease characterization and outcome.2 One of the major challenges and ongoing research efforts facing the post-genomic period is to connect the nearly 26,000 protein-coding genes of mammalian organisms to the genetic basis of complex polygenic diseases and the integrated function of complex biologic systems. The rapidly evolving field of genomic medicine proposes to use genomic information to assist medical decision-making and tailor health care to the individual patient.
A breathtaking acceleration on the path from the Human Genome Project to genomic medicine has been paved by landmark “big biology” efforts concentrated on the main themes of uncovering the function of human genes, identifying all human genomic variation, and elucidating the genetic basis for human diseases. Furthermore, dramatic improvements in sequencing technologies and cost reductions have fueled even more ambitious initiatives such as the ongoing Personal Genome Project,3 which aims to publish the complete genomes and medical records of a number of volunteers in order to enable personalized medicine research. Nevertheless, it is fair to say a decade after its completion, although the Human Genome Project has revolutionized the pace and scope of biomedical science, it has failed so far to fulfill the promise and grand vision of “personalized medicine,” leaving the scientific community somewhat sobered and divided.


With increasing evidence suggesting that genetic variation can significantly modulate risk of adverse perioperative events,5,6,7,8 the emerging field of perioperative genomics aims to apply functional genomic approaches to discover underlying biologic mechanisms that explain why similar patients have such dramatically different outcomes after surgery and is justified by a unique combination of environmental insults and postoperative phenotypes that characterize surgical and critically ill patient populations. To integrate this new generation of genetic results into clinical practice, perioperative physicians need to understand the patterns of human genome variation, the methods of population-based genetic investigation, and the principles of gene and protein expression analysis. This chapter reviews general genetic/genomic concepts and highlights current and future applications of genomic technologies for perioperative risk stratification, outcome prediction, mechanistic understanding of surgical stress responses, as well as identification and validation of novel targets for perioperative organ protection.
Table 6-1. Categories of Perioperative Phenotypes | ||||
---|---|---|---|---|
|
Overview of Human Genomic Variation
Although the human DNA sequence is 99.9% identical between individuals, the variations may greatly affect a person’s disease susceptibility. In elucidating the genetic basis of disease, much of what has been investigated in the pre-Human Genome Project era focused on identifying rare genetic variants (mutations) responsible for >1,500 monogenic disorders such as hypertrophic cardiomyopathy, long QT syndrome, sickle cell anemia, cystic fibrosis, or familial hypercholesterolemia, which are highly penetrant (carriers of the mutant gene will likely have the disease) and inherited in Mendelian fashion (hence termed Mendelian diseases). However, most of the genetic diversity in the population is attributable to more widespread DNA sequence variations (polymorphisms), typically single nucleotide base substitutions [single nucleotide polymorphisms (SNPs)], or to a broader category of previously overlooked structural genetic variants which include short sequence repeats (microsatellites), insertion/deletion (I/D) of one or more nucleotides (indels), inversions,
and the recently discovered copy number variants (CNVs, large segments of DNA that vary in number of copies)9 all of which may or may not be associated with a specific phenotype (Fig. 6-2). To be classified as a polymorphism, the DNA sequence alternatives (i.e., alleles) must exist with a frequency of at least 1% in the population. About 15 million SNPs are estimated to exist in the human genome, approximately once every 300 base pairs, located in genes as well as in the surrounding regions of the genome. Polymorphisms may directly alter the amino acid sequence and therefore potentially alter protein function or alter regulatory DNA sequences that modulate protein expression. Sets of nearby SNPs on a chromosome are inherited in blocks, referred to as haplotypes. As it will be shown later, haplotype analysis is a useful way of applying genotype information in disease gene discovery. On the other hand, CNVs involve approximately 12% of the human genome, often encompass genes (especially regulating inflammation and brain development), and may influence disease susceptibility through dosage imbalances. The year 2007 was marked by the realization that DNA differs from person to person much more than previously suspected; equipped with faster and cheaper DNA sequencing technologies, researchers have catalogued more than 3 million SNPs as part of the HapMap Project,10 published the first diploid genome sequence of an individual human,11 launched the 1000 Genomes Project (sequencing the genomes of 1,000 people from around the world), and begun charting CNVs and other structural variants, thus making understanding of human genetic variation the 2007 Science magazine “Breakthrough of the Year.” Below, we review the common strategies used to incorporate genetic analysis into clinical studies.
and the recently discovered copy number variants (CNVs, large segments of DNA that vary in number of copies)9 all of which may or may not be associated with a specific phenotype (Fig. 6-2). To be classified as a polymorphism, the DNA sequence alternatives (i.e., alleles) must exist with a frequency of at least 1% in the population. About 15 million SNPs are estimated to exist in the human genome, approximately once every 300 base pairs, located in genes as well as in the surrounding regions of the genome. Polymorphisms may directly alter the amino acid sequence and therefore potentially alter protein function or alter regulatory DNA sequences that modulate protein expression. Sets of nearby SNPs on a chromosome are inherited in blocks, referred to as haplotypes. As it will be shown later, haplotype analysis is a useful way of applying genotype information in disease gene discovery. On the other hand, CNVs involve approximately 12% of the human genome, often encompass genes (especially regulating inflammation and brain development), and may influence disease susceptibility through dosage imbalances. The year 2007 was marked by the realization that DNA differs from person to person much more than previously suspected; equipped with faster and cheaper DNA sequencing technologies, researchers have catalogued more than 3 million SNPs as part of the HapMap Project,10 published the first diploid genome sequence of an individual human,11 launched the 1000 Genomes Project (sequencing the genomes of 1,000 people from around the world), and begun charting CNVs and other structural variants, thus making understanding of human genetic variation the 2007 Science magazine “Breakthrough of the Year.” Below, we review the common strategies used to incorporate genetic analysis into clinical studies.
Methodologic Approaches to Study the Genetic Architecture of Common Complex Diseases
Most ongoing research on complex disorders focuses on identifying genetic polymorphisms that enhance susceptibility to given conditions. Often the design of such studies is complicated by the presence of multiple risk factors, gene–environment interactions, and a lack of even rough estimates of the number of genes underlying such complex traits. Two broad strategies are being employed to identify complex trait loci. The candidate gene approach is motivated by what is known about the trait biologically and can be characterized as a hypothesis-testing approach, but is intrinsically biased. The second strategy is the genome-wide scan, in which thousands of markers uniformly distributed throughout the genome are used to locate regions that may harbor genes influencing the phenotypic variability. This is a hypothesis-free and unbiased approach, in the sense that no prior assumptions are being made about the biologic processes involved and no weight is given to known genes, thus allowing the detection of previously unknown trait loci. Both the candidate gene and the genome scan approaches can be implemented using one of two fundamental methods of identifying polymorphisms affecting common diseases: Linkage analysis or association studies in human populations.
Linkage Analysis. Used to identify the chromosomal location of gene variants related to a given disease by studying the distribution of disease alleles in affected individuals throughout a pedigree, linkage analysis has successfully mapped hundreds of genes for rare, monogenic disorders. However, common complex diseases are characterized by a multitude of genes with rare and/or common alleles, which create an apparently chaotic pattern of heterogeneity within and between families. The overall effect of this heterogeneity, together with the potentially weak influence of many loci, places a heavy burden on the statistical power needed to detect individual contributing genes and may be the reason why very few genome linkage scans so far have yielded disease loci that meet genome-wide significance criteria.12 Furthermore, the nature of most complex diseases (especially for perioperative adverse events) precludes the study of extended multigenerational family pedigrees. Nevertheless, a few positive findings have emerged using this approach: A stroke susceptibility locus was mapped on chromosome 5q12,13 risk of myocardial infarction was mapped to a single region on chromosome 14,14 and a recent meta-analysis of several genome-wide scans for pulse pressure variation, an emerging risk factor for perioperative complications, has identified several linkage bins on chromosomes 22 and 10.15
Genetic Association Studies. Association studies examine the frequency of specific genetic polymorphisms in a population-based sample of unrelated diseased individuals and appropriately matched unaffected controls. The increased statistical power to uncover small clinical effects of multiple genes16 and the fact that they do not require family-based sample collections are the main advantages of this approach over linkage analysis. Until very recently, most significant results in dissecting common complex diseases were gathered from candidate gene association studies, with genes selected because of a priori hypotheses about their potential etiologic role in disease based on current understanding of the disease pathophysiology.17 For example, genetic variants
within the renin–angiotensin system,18 nitric oxide synthase (NOS),19 and β2-adrenergic receptors (AR),20 known to modulate vascular tone, were tested and found to be associated with hypertension. Similarly, the possible effects of polymorphisms on genetic predisposition for CAD21 or restenosis after angioplasty22 have been extensively investigated; more recently, two large-scale association studies have identified gene variants that might affect susceptibility to myocardial infarction.23 As it will be presented in more detail later, accumulating evidence from candidate gene association studies also suggests that specific genotypes are associated with a variety of organ-specific perioperative adverse outcomes, including myocardial infarction,24,25 neurocognitive dysfunction,26,27,28 renal compromise,29,30,31 vein graft restenosis,32,33 postoperative thrombosis,34 vascular reactivity,35 severe sepsis,36,37 transplant rejection,38 and death [for reviews, see (5,8)].
within the renin–angiotensin system,18 nitric oxide synthase (NOS),19 and β2-adrenergic receptors (AR),20 known to modulate vascular tone, were tested and found to be associated with hypertension. Similarly, the possible effects of polymorphisms on genetic predisposition for CAD21 or restenosis after angioplasty22 have been extensively investigated; more recently, two large-scale association studies have identified gene variants that might affect susceptibility to myocardial infarction.23 As it will be presented in more detail later, accumulating evidence from candidate gene association studies also suggests that specific genotypes are associated with a variety of organ-specific perioperative adverse outcomes, including myocardial infarction,24,25 neurocognitive dysfunction,26,27,28 renal compromise,29,30,31 vein graft restenosis,32,33 postoperative thrombosis,34 vascular reactivity,35 severe sepsis,36,37 transplant rejection,38 and death [for reviews, see (5,8)].
One of the main weaknesses of the candidate gene association approach is that, unless the marker of interest “travels” (i.e., is in linkage disequilibrium) with a functional variant, or the marker allele is the actual functional variant, the power to detect and map complex trait loci will be reduced. Other known limitations of genetic association studies include potential false-positive findings resulting from population stratification (i.e., admixture of different ethnic or genetic backgrounds in the case and control groups) and multiple comparison issues when large numbers of candidate genes are being assessed.39 Replication of findings across different populations or related phenotypes remains the most reliable method of validating a true relationship between genetic polymorphisms and disease,17 but poor reproducibility in subsequent studies has been one of the main criticisms of the candidate gene association approach.40 However, a recent meta-analysis suggested that lack of statistical power may be the main contributor to this inconsistent replication and proposed more stringent statistical criteria to exclude false-positive results and the design of large collaborative association studies.41
At last, after several decades of frustrating limitations in the ability to find genetic variations responsible for common disease risk, with the completion of the second phase of the International HapMap Project (a high-resolution maps of human genetic variation and haplotypes)10 and advances in high-throughput genotyping technologies, the year 2007 marked an explosion of adequately powered and successfully replicated genome-wide association studies (GWAS) that identified very significant genetic contributors to risk for common polygenic diseases like CAD,42,43,44 myocardial infarction,45 diabetes (types I and II),46,47 atrial fibrillation,48 obesity,49 asthma, common cancers, rheumatoid arthritis, Crohn’s disease, and others. GWAS make use of the known linkage disequilibrium pattern between SNPs from the human HapMap and the new high-density SNP chip technology to comprehensively interrogate between 65% and 80% of common variation across the genome, with even higher coverage being possible using statistical imputation techniques. The largest, most comprehensive GWAS to date was conducted by the Wellcome Trust Case-Control Consortium, investigating the association between 500,000 SNPs and 7 common diseases in 2,000 cases and 3,000 shared controls, which identified 25 independent association signals at stringent levels of significance (P < 5 × 10−7).42 Interestingly, variants in or near CDKN2A/B (cyclin-dependent kinase inhibitor 2 A/B) conferred increased risk for both type II diabetes (odds ratio (OR) 1.2, P = 7.8 × 10−15) and myocardial infarction (OR 1.64, P = 1.2 × 10−20), which may lead to a mechanistic explanation for the link between the two disorders. This finding also highlights the power of GWAS to identify variants outside described genes: While one of the signals occurs in the CDKN2A/B region, the other much stronger association signal occurs >200 kb from these genes, in a gene desert, and thus would not have been picked up by a candidate gene approach. Identifying the mechanism by which this variant may affect CDKN2A/B expression will provide new insights into the regulation of these important genes.
Large-Scale Gene and Protein Expression Profiling: Static Versus Dynamic Genomic Markers of Perioperative Outcomes
Genomic approaches are anchored in the “central dogma” of molecular biology, the concept of transcription of messenger RNA (mRNA) from a DNA template, followed by translation of RNA into protein (Fig. 6-3). Since transcription is a key regulatory step that may eventually signal many other cascades of events, the study of RNA levels in a cell or organ (i.e., quantifying gene expression) can improve the understanding of a wide variety of biologic systems. Furthermore, while the human genome contains only about 25,000 genes, functional variability at the protein level is far more diverse, resulting from extensive posttranscriptional, translational, and posttranslational modifications. It is believed that there are approximately 200,000 distinct proteins in humans, which are further modified posttranslationally by phosphorylation, glycosylation, SUMOylation, oxidation, and disulfide structures. The “static” view of constitutive DNA sequence variants potentially involved in the pathophysiology of perioperative complications can be complemented by a “dynamic” view that integrates their functionality. There is increasing evidence that variability in gene expression levels underlies complex disease and is determined by regulatory DNA polymorphisms affecting transcription, splicing,
and translation efficiency in a tissue- and stimulus-specific manner.50 Thus, analysis of large-scale variability in the pattern of RNA, microRNA (miRNA), and protein expression both at baseline and in response to the multidimensional perioperative stimuli (dynamic genomics) using microarray and proteomic approaches provides a much needed understanding of the overall regulatory networks involved in the pathophysiology of adverse postoperative outcomes. This information is complementary to the assessment of genetic variability at the DNA sequence level using various genotyping techniques as described in previous sections (static genomics). Such dynamic genomic markers can be incorporated in genomic classifiers and used clinically to improve perioperative risk stratification or monitor postoperative recovery.51 This emergent concept of molecular classification involves the description of informational features in a training dataset using changes in relative RNA, miRNA, protein, or metabolite abundance in the context of genetic predisposition and applying to a test dataset to recognize a defined “fingerprint” characteristic of a particular perioperative phenotype (Table 6-2). For example, Feezor et al. used a combined genomic and proteomic approach to identify expression patterns of 138 genes from peripheral blood leukocytes and the concentrations of 7 circulating plasma proteins that discriminated patients who developed multiple organ dysfunction syndrome (MODS) after thoracoabdominal aortic aneurysm repair from those who did not. More importantly, these patterns of genome-wide gene expression and plasma protein concentration were observed before surgical trauma and visceral ischemia-reperfusion injury, suggesting that patients who developed MODS differed in either their genetic predisposition or their preexisting inflammatory state.52
and translation efficiency in a tissue- and stimulus-specific manner.50 Thus, analysis of large-scale variability in the pattern of RNA, microRNA (miRNA), and protein expression both at baseline and in response to the multidimensional perioperative stimuli (dynamic genomics) using microarray and proteomic approaches provides a much needed understanding of the overall regulatory networks involved in the pathophysiology of adverse postoperative outcomes. This information is complementary to the assessment of genetic variability at the DNA sequence level using various genotyping techniques as described in previous sections (static genomics). Such dynamic genomic markers can be incorporated in genomic classifiers and used clinically to improve perioperative risk stratification or monitor postoperative recovery.51 This emergent concept of molecular classification involves the description of informational features in a training dataset using changes in relative RNA, miRNA, protein, or metabolite abundance in the context of genetic predisposition and applying to a test dataset to recognize a defined “fingerprint” characteristic of a particular perioperative phenotype (Table 6-2). For example, Feezor et al. used a combined genomic and proteomic approach to identify expression patterns of 138 genes from peripheral blood leukocytes and the concentrations of 7 circulating plasma proteins that discriminated patients who developed multiple organ dysfunction syndrome (MODS) after thoracoabdominal aortic aneurysm repair from those who did not. More importantly, these patterns of genome-wide gene expression and plasma protein concentration were observed before surgical trauma and visceral ischemia-reperfusion injury, suggesting that patients who developed MODS differed in either their genetic predisposition or their preexisting inflammatory state.52
Table 6-2. Summary of Gene Expression Studies with Implications for Perioperative Cardiovascular Outcomes | ||||||||||||||||||||||||||||||||||||||||||||||||||||||||||||||||||||||||
---|---|---|---|---|---|---|---|---|---|---|---|---|---|---|---|---|---|---|---|---|---|---|---|---|---|---|---|---|---|---|---|---|---|---|---|---|---|---|---|---|---|---|---|---|---|---|---|---|---|---|---|---|---|---|---|---|---|---|---|---|---|---|---|---|---|---|---|---|---|---|---|---|
|
Alternatively, dynamic genomic markers can be used to improve mechanistic understanding of perioperative stress, and to evaluate and catalogue organ-specific responses to surgical stress and severe systemic stimuli such as cardiopulmonary bypass (CPB)
and endotoxemia, which can be subsequently used to identify and validate novel targets for organ-protective strategies.69 Similarly, integrated transcriptomic and proteomic analyses have been used to characterize the peripheral blood molecular response signatures to cardiac surgery with and without CPB, a robust trigger of systemic inflammation.70 The study demonstrated that, rather than being the primary source of serum cytokines, peripheral blood leukocytes only assume a “primed” phenotype upon contact with the extracorporeal circuit which facilitates their trapping and subsequent tissue-associated inflammatory response. Interestingly, many inflammatory mediators achieved similar systemic levels following off-pump surgery but with delayed kinetics, offering novel insights into the concepts of contact activation and compartmentalization of inflammatory responses to major surgery.
and endotoxemia, which can be subsequently used to identify and validate novel targets for organ-protective strategies.69 Similarly, integrated transcriptomic and proteomic analyses have been used to characterize the peripheral blood molecular response signatures to cardiac surgery with and without CPB, a robust trigger of systemic inflammation.70 The study demonstrated that, rather than being the primary source of serum cytokines, peripheral blood leukocytes only assume a “primed” phenotype upon contact with the extracorporeal circuit which facilitates their trapping and subsequent tissue-associated inflammatory response. Interestingly, many inflammatory mediators achieved similar systemic levels following off-pump surgery but with delayed kinetics, offering novel insights into the concepts of contact activation and compartmentalization of inflammatory responses to major surgery.
Several studies have profiled myocardial gene expression in the ischemic heart, demonstrating alterations in the expression of immediate-early genes (c-fos, junB), as well as genes coding for calcium-handling proteins (calsequestrin, phospholamban), extracellular matrix, and cytoskeletal proteins.53 Upregulation of transcripts mechanistically involved in cytoprotection (heat shock proteins), resistance to apoptosis, and cell growth has been found in stunned myocardium.71 Moreover, cardiac gene expression profiling after CPB and cardioplegic arrest has identified the upregulation of inflammatory and transcription activators, apoptotic genes, and stress genes,54 which appear to be age-related.55 Microarray technology has also been utilized in the quest for novel cardioprotective genes, with the ultimate goal of designing strategies to activate these genes and prevent myocardial injury. Preconditioning is one of such well-studied models of cardioprotection, which can be induced by various triggers including intermittent ischemia, osmotic or redox stress, heat shock, toxins, and inhaled anesthetics. The main functional categories of genes identified as potentially involved in cardioprotective pathways include a host of transcription factors, heat shock proteins, antioxidant genes (heme-oxygenase, glutathione peroxidase), and growth factors, but different gene programs appear to be activated in ischemic versus anesthetic preconditioning, resulting in two distinct cardioprotective phenotypes.56 More recently, a transcriptional response pattern consistent with late preconditioning has been reported in peripheral blood leukocytes following sevoflurane administration in healthy volunteers, characterized by reduced expression of L-selectin as well as downregulation of genes involved in fatty acid oxidation and the PCG1α (peroxisome-activated receptor γ coactivator 1α) pathway,59 which mirrors changes observed in the myocardium from patients undergoing off-pump coronary artery bypass grafting (CABG) (OPCAB; Table 6-2).58 Deregulation of these novel survival pathways thus appears to generalize across tissues, making them important targets for cardioprotection, but further studies are needed to correlate perioperative gene expression response patterns in end organs such as the myocardium to those in readily available potential surrogate tissues such as peripheral blood leukocytes.
The transcriptome (the complete collection of transcribed elements of the genome) is not fully representative of the proteome (the complete complement of proteins encoded by the genome), since many transcripts are not targeted for translation, as evidenced recently with the concept of gene silencing by RNA interference. Alternative splicing, a wide variety of posttranslational modifications, and protein–protein interactions responsible for biologic function would remain therefore undetected by gene expression profiling (Fig. 6-3). This has led to the emergence of a new field, proteomics, studying the sequence, modification, and function of many proteins in a biologic system at a given time. Rather than focusing on “static” DNA, proteomic studies examine dynamic protein products, with the goal of identifying proteins that undergo changes in abundance, modification, or localization in response to a particular disease state, trauma, stress, or therapeutic intervention [for a review, see (72)]. Thus, proteomics offers a more global and integrated view of biology, complementing other functional genomic approaches. Currently available methods for proteomic analysis include protein extraction, separation by two-dimensional gel electrophoresis or chromatography, followed by identification using mass spectrometry. Although rapidly improving, these methods are currently limited by sensitivity, specificity, and throughput. Several preclinical proteomic studies relevant to perioperative medicine have characterized the temporal changes in brain protein expression in response to various inhaled anesthetics73,74 or following cardiac surgery with hypothermic circulatory arrest.75 This may focus further studies aimed to identify new anesthetic binding sites and the development of neuroprotective strategies. Furthermore, detailed knowledge of the plasma proteome has profound implications in perioperative transfusion medicine,76 in particular related to peptide and protein changes that occur during storage of blood products. The development of protein arrays and real-time proteomic analysis technologies has the potential to allow the use of these versatile and rigorous high-throughput methods for clinical applications and is the object of intense investigation.
Genomics and Perioperative Risk Profiling
More than 40 million patients undergo surgery annually in the United States at a cost of $450 billion. Each year approximately 1 million patients sustain medical complications after surgery, resulting in costs of $25 billion annually. The proportion of the US population older than 65 is estimated to double in the next two decades, leading to a 25% increase in the number of surgeries, a 50% increase in surgery-related costs, and a 100% increase in complications from surgery. Recognizing the significant increase in surgical burden due to accelerated aging of the population and increased reliance on surgery for treatment of disease, the National Heart, Blood and Lung Institute has recently convened a working group on perioperative medicine. The group concluded that perioperative complications are significant, costly, variably reported, and often imprecisely detected, and identified a critical need for accurate comprehensive perioperative outcome databases. Furthermore, presurgical risk profiling is inconsistent and deserves further attention, especially for noncardiac, nonvascular surgery and older patients.77

atherosclerotic cardiovascular disease and neurologic injury may facilitate preoperative patient optimization and resource utilization. Furthermore, understanding the role of allotypic variation in pro-inflammatory and pro-thrombotic pathways, the main pathophysiologic mechanisms responsible for perioperative complications, may contribute to the development of target-specific therapies, thereby limiting the incidence of adverse events in high-risk patients. Recently, rigorous methodologic frameworks for the design and analysis of studies aiming to evaluate the clinical validity and utility of genetic risk prediction have been developed,78 together with detailed recommendations for reporting their results.79 To increase clinical relevance for the practicing perioperative and critical care physician, we summarize below existing evidence by specific outcome while highlighting candidate genes in relevant mechanistic pathways (Tables 6-3–6-5).
Predictive Biomarkers for Perioperative Adverse Cardiac Events
As part of the preoperative evaluation, anesthesiologists are involved in assessing the risks of perioperative complications. It is commonly accepted that patients who have underlying cardiovascular disease are at risk for adverse cardiac events after surgery, and several multifactorial risk indices have been developed and validated for patients undergoing noncardiac surgical procedures (such as the Goldman or the Lee Cardiac risk index), as well as cardiac surgery (such as the Hannan or Sergeant scores). However, identifying patients at the highest risk of perioperative infarction remains difficult, and risk scores, while potentially valuable for population studies, are not an ideal tool for directing care in an individual patient.130 Genomic approaches have been used in the search for a better assessment of the individual coronary risk profile. Numerous reports from animal models, linkage analysis, family, twin, and population association studies have definitely proven the role of genetic influences in the incidence and progression of CAD, with a heritability of death from CAD as high as 58%; furthermore, hazardous patterns of angiographic CAD (left main and proximal disease), known major risk factors for perioperative cardiac complications, are also highly heritable. Similarly, genetic susceptibility to myocardial infarction (MI) has been established through multiple lines of evidence14,23 including a recent well-powered and replicated GWAS.45 While these studies do not directly address the heritability of adverse perioperative myocardial events, they do suggest a strong genetic contribution to the risk of adverse cardiovascular outcomes in general.
Table 6-3. Representative Genetic Polymorphisms Associated with Altered Susceptibility to Adverse Perioperative Cardiovascular Events | |||||||||||||||||||||||||||||||||||||||||||||||||||||||||||||||||||||||||||||||||||||||||||||||||||||||||||||||||||||||||||||||||||||||||||||||||||||||||||||||||||||||||||||||||||||||||||||||||||||||||||||||||||||||||||||||||||||||||||||||||||||||||||||||||||||||||||||||||||||||||||||
---|---|---|---|---|---|---|---|---|---|---|---|---|---|---|---|---|---|---|---|---|---|---|---|---|---|---|---|---|---|---|---|---|---|---|---|---|---|---|---|---|---|---|---|---|---|---|---|---|---|---|---|---|---|---|---|---|---|---|---|---|---|---|---|---|---|---|---|---|---|---|---|---|---|---|---|---|---|---|---|---|---|---|---|---|---|---|---|---|---|---|---|---|---|---|---|---|---|---|---|---|---|---|---|---|---|---|---|---|---|---|---|---|---|---|---|---|---|---|---|---|---|---|---|---|---|---|---|---|---|---|---|---|---|---|---|---|---|---|---|---|---|---|---|---|---|---|---|---|---|---|---|---|---|---|---|---|---|---|---|---|---|---|---|---|---|---|---|---|---|---|---|---|---|---|---|---|---|---|---|---|---|---|---|---|---|---|---|---|---|---|---|---|---|---|---|---|---|---|---|---|---|---|---|---|---|---|---|---|---|---|---|---|---|---|---|---|---|---|---|---|---|---|---|---|---|---|---|---|---|---|---|---|---|---|---|---|---|---|---|---|---|---|---|---|---|---|---|---|---|---|---|---|---|---|---|---|---|---|---|---|---|---|---|---|---|---|---|---|---|---|---|---|---|---|---|---|---|---|---|---|---|---|---|---|---|
|
Table 6-4. Representative Genetic Polymorphisms Associated with Altered Susceptibility to Adverse Perioperative Neurologic Events | |||||||||||||||||||||||||||||||||||||||||||||||||||||||||||||||||
---|---|---|---|---|---|---|---|---|---|---|---|---|---|---|---|---|---|---|---|---|---|---|---|---|---|---|---|---|---|---|---|---|---|---|---|---|---|---|---|---|---|---|---|---|---|---|---|---|---|---|---|---|---|---|---|---|---|---|---|---|---|---|---|---|---|
|
Table 6-5. Representative Genetic Polymorphisms Associated with other Adverse Perioperative Outcomes | |||||||||||||||||||||||||||||||||||||||||||||||||||||||||||||||||||||||||||||||||||||||||||||||||||||||||||||||||||||||||||||
---|---|---|---|---|---|---|---|---|---|---|---|---|---|---|---|---|---|---|---|---|---|---|---|---|---|---|---|---|---|---|---|---|---|---|---|---|---|---|---|---|---|---|---|---|---|---|---|---|---|---|---|---|---|---|---|---|---|---|---|---|---|---|---|---|---|---|---|---|---|---|---|---|---|---|---|---|---|---|---|---|---|---|---|---|---|---|---|---|---|---|---|---|---|---|---|---|---|---|---|---|---|---|---|---|---|---|---|---|---|---|---|---|---|---|---|---|---|---|---|---|---|---|---|---|---|
|
Despite advances in surgical, cardioprotective, and anesthetic techniques, the incidence of perioperative myocardial infarction (PMI) following cardiac and vascular surgery in several large randomized clinical trials has been reported at 7% to 19%131,132 and is consistently associated with reduced short- and long-term survival. In the setting of cardiac surgery, PMI involves three major converging pathophysiologic processes, including systemic and local inflammation, “vulnerable” blood, and neuroendocrine stress.5 In noncardiac surgery, pathophysiology of PMI is not so clearly understood, but a combination of two mechanisms appears predominant: (1) Plaque rupture and coronary thrombosis triggered by perioperative endothelial injury from catecholamine surges and pro-inflammatory and pro-thrombotic states; and (2) prolonged stress-induced ischemia and tachycardia in the setting of compromised perfusion. Extensive genetic variability exists in each of these mechanistic pathways, which may combine to modulate the magnitude of myocardial injury. However, only a paucity of studies exist relating genetic risk factors to adverse perioperative myocardial outcomes, mainly following CABG surgery (Table 6-3).32,85,89
Inflammation Biomarkers and Perioperative Adverse Cardiac Events
Although the role of inflammation in cardiovascular disease biology has long been established, we are just beginning to understand the relationship between genetically controlled variability in inflammatory responses to surgery and PMI pathogenesis. Consistent with the “inflammatory hypothesis” in the pathogenesis of perioperative organ injury, our group has recently identified three inflammatory gene polymorphisms that are independently predictive of PMI following cardiac surgery with CPB. These include the pro-inflammatory cytokine interleukin 6 (IL6)–572G > C (OR 2.47) and two adhesion molecules—intercellular adhesion molecule 1 (ICAM1 Lys469Glu, OR 1.88) and E-selectin (SELE 98G > T, OR 0.16).24 Importantly, inclusion of genotypic information from these SNPs improves prediction models for postcardiac surgery MI based on traditional risk factors alone. Using a similar definition of PMI, Collard et al. have reported that a combined haplotype in the mannose-binding lectin gene (MBL2 LYQA secretor haplotype), an important recognition molecule in the lectin complement pathway, is independently associated with PMI in a cohort of Caucasian patients undergoing primary CABG with CPB.25 Furthermore, genetic variants in IL6 and TNFA are associated with increased incidence of postoperative cardiovascular complications (a composite outcome that included PMI) following lung resection for cancer.92 Other genetic variants modulating the magnitude of postoperative inflammatory response have been identified. Polymorphisms in the promoter of the IL6 gene (−572G > C and −174G > C) significantly increase the inflammatory response after heart surgery with CPB133 and have been associated with length of hospitalization after CABG.134 Furthermore, apolipoprotein E (APOE) genotype (the ε4 allele),135 several variants in the tumor necrosis factor (TNF) genes [tumor necrosis factor α (TNFA)–308G > A, lymphotoxin α (LTA) + 250G > A],136 and a functional SNP in the macrophage migration inhibitory factor (MIF)137 have been associated with pro-inflammatory effects in patients undergoing CPB and in some instances with postoperative ventricular dysfunction.93 Conversely, a genetic variant modulating the release of the anti-inflammatory cytokine interleukin 10 (IL10) in response to CPB has been reported (IL10–1082G > A), with high levels of IL10 being associated with postoperative cardiovascular dysfunction.94 In patients undergoing elective surgical revascularization for peripheral vascular disease, several SNPs in IL6 and IL10 were associated with endothelial dysfunction and increased risk of a composite endpoint of acute postoperative cardiovascular events.91 Overall, while genetic factors may not be better predictors of outcomes than intermediate phenotypes (e.g., plasma cytokine levels), their greater ease of assessment, stability, and availability pre-procedure are significant advantages influencing potential future clinical utility.

Full access? Get Clinical Tree
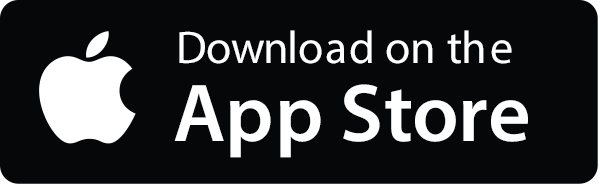

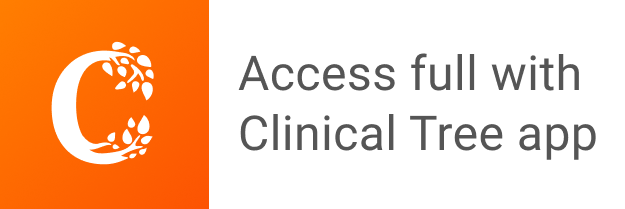