Chapter 99 Genomic and Proteomic Medicine in Critical Care
Genomics
From the Discovery of the Double Helix to the Human Genome Project
In 1953, in a manuscript that scarcely exceeded one page,1 the double helical structure of deoxyribonucleic acid (DNA) was described. This brief report opened the door for a new understanding of heredity and gene function. As a direct result of this discovery, the field of molecular biology emerged and the task of deciphering the genetic code began. Initially, progress was slow, depending mostly on methodical detective work and a certain measure of luck. It was not until 1983, with the localization of the mutation for Huntington’s disease to chromosome 4, that a gene was unequivocally linked to a physical location within the human genome (Figure 99-1). Another 10 years would elapse before the sequence of this gene was known and the molecular abnormality causing Huntington disease was identified. The cystic fibrosis gene was among the earliest disease-causing genes to be sequenced in 1989. Among the insights gained was that the disease was genetically heterogenous. Only 70% of patients with this illness had the most common mutation (ΔF508); the remaining patients could have any of hundreds of mutations in the chloride-channel protein encoded by this gene. Knowledge of variation in the gene’s sequence helped to explain the tremendous diversity in the clinical manifestations of this disease.
Gene Expression and Microarrays
High-throughput automated DNA sequencing was developed in the late 1980s, allowing rapid determination of DNA sequences. This development has led to new challenges, because managing the large volumes of sequence data demanded new technologies. Fortunately, the rapid increase in computing power in mainframe and desktop computers and the availability of the Internet to link investigators to public databases provided a solution to this problem. A fusion of these technologies greatly accelerated the pace of gene sequencing.
In the late 1980s and early 1990s, leaders from the National Institutes of Health and the Department of Energy began to create the infrastructure necessary for large-scale sequencing of the human genome. Although the Human Genome Project began in the early 1990s, the international effort to sequence the entire genome did not begin until 1998.2 Remarkably, this massive project was completed just 5 years later.3 Scientists throughout the world who participated in the human genome project contributed DNA sequence data to public databases so that the entire sequence of the human genome is freely available to the scientific community.
In the early 1990s, as the DNA sequence of an increasing number of genes became available, investigators began to experiment with gene expression microarrays. These investigators made microarrays from slides with a series of spots, in which each spot contained the DNA from a single gene. As the technology improved, the investigators were able to examine the expression of an increasingly larger number of genes in a single experiment. In the earliest published experiments, the investigators examined expression patterns of 45 yeast genes.4 Less than a decade later, commercially produced gene expression microarrays were available with more than 30,000 human genes on each array.5 The field of functional genomics emerged when gene expression microarrays made it feasible to study the expression of thousands of genes at once. Gene expression microarray use has increased rapidly since 1995, when the first gene expression microarray publications appeared in the literature. These powerful tools are readily available to laboratory investigators and are beginning to find their way into clinical practice. Within the next few years, gene expression microarrays and other high-throughput technologies will find increasing numbers of applications in clinical medicine.
Quantifying Gene Expression
Microarray experiments usually are designed so that gene expression from two tissue samples may be compared either directly or indirectly.6 This allows investigators to learn how different conditions can alter gene expression. For example, much has been learned about the biology of cancer by comparing gene expression from cancer cells to gene expression from normal cells in the same tissue.
When a gene is expressed in a cell, the DNA from that gene is transcribed into messenger ribonucleic acid (mRNA). This is transported out of the nucleus and then used as a template to guide the synthesis of proteins in the cell. At any given time, the mRNA content of a cell represents a snapshot of the genes being expressed and the proteins being made in the cell. If a gene is turned on (upregulated), more mRNA will be produced from that gene. Conversely, if a gene is turned off (downregulated), less mRNA will be produced from that gene. To quantify gene expression, mRNA is extracted from the cell and reverse transcribed to make cDNA that is labeled with a fluorescent dye. The labeled cDNA is then incubated on a microarray slide to permit the hybridization with complementary DNA oligonucleotide probes that are bound to the surface of the slide (Figure 99-2). The amount of labeled cDNA hybridized to each oligonucleotide spot will be proportional to the quantity of mRNA that was expressed from the target gene.
Genes and Human Variation
When contemplating the great diversity among humans, it is somewhat surprising to realize that the DNA of two unrelated humans is more than 99.9% identical.7 Although the vast majority of nuclear DNA is identical from one person to the next, a small fraction of DNA sequence (~0.1%) varies between individuals and is responsible for the genetically determined variation in our physical characteristics and physiology. Genetic variability also appears to be involved with susceptibility to some diseases, as well as therapeutic responses to treatment.
The sequencing of the human genome (actually now several individuals’ genomes) and the advent of high-throughput sequencing and genotyping technologies has revolutionized the understanding of gene structure and genetic variation. Many genes are polymorphic; that is, there are small differences in DNA sequence between individuals. Polymorphisms are sites in DNA in which variation at a specific nucleotide or DNA region is found in greater than 1% of the general population, and in some instances in as much as 50% of the population. (Mutations are considered to be sites at which variation occurs in 1% or less of the population.) Polymorphisms may alter protein level or function in several ways. For example, altering a single base can alter an amino acid in a protein, which may lead to a change in protein function. Polymorphisms can also have significant effects without altering proteins. A polymorphism occurring in a promoter region, that controls gene expression through controlling mRNA synthesis, may lead to increased or decreased synthesis of that protein, which may have significant effects. Although polymorphisms are still being mapped and the function of most polymorphisms is still being defined, it is clear that these genetic variations account for the vast majority of inherited human phenotypes, from differences in hair color to differences in response to medications.
Single-Nucleotide Polymorphisms
Although such substitutions may occur spontaneously and represent a new mutation, the vast majority of the observed substitutions are stable variations in the human gene pool. SNPs are the most common type of polymorphism, and are thought to account for approximately 90% of human variation.8 One SNP is believed to occur in every 100 to 300 bases. Although most of the SNPs in the human genome remain to be identified, if this figure holds true for the entire genome, then more than 20 million SNPs exist in our genome, and constitute an enormous source of variation.
Copy-Number Variations
In addition, polymorphisms within genes may be due to insertions or deletions of fragments of DNA, or to the presence of a variable number of tandem repeats (VNTRs) of short, repetitive DNA sequences. Some of these insertions or deletions, although submicroscopic, can be relatively large, resulting in gene copy-number variations (CNVs). CNVs are generally defined as stretches of DNA of greater than 1 kb that show differences in the expected number of copies of the DNA (that generally would be two due to the presence of one copy on each chromosome) in greater than 1% of the human population.9,10 Very recently it has become clear that CNVs are common in human genomes and contribute significantly to human genetic variation.11,12 In addition, CNVs have been demonstrated to be associated with a number of diseases including Williams-Beuren syndrome, DiGeorge syndrome, mental retardation, and autism.9,10 It is thought that alterations in phenotypes due to CNVs are due to differences in gene dosage or to gene disruption that may be caused during the duplication or deletion. This is a new and rapidly expanding field and the next 10 years will likely determine how large a role CNVs play in human variation and disease.
Genotyping and Microarrays
DNA microarrays have been designed for both SNP and CNV genotyping. SNP microarrays are similar to those used for gene expression studies, but the oligonucleotides on SNP arrays have each been designed to selectively hybridize with one form of an SNP. Some of these microarrays have probes for almost a million different SNPs from throughout the genome and can quickly reveal the genotypes of an individual at these sites. Many of these same arrays also have the ability to genotype the individual for almost a million CNVs. Such arrays have been used for genome-wide association studies to identify genes associated with complex diseases such as asthma13–16 and diabetes.17–19 The ability to define the genetic components of variation with speed and precision is more than a valuable research tool. In the near future, this information will be used to identify disease and to help select therapies for illness, taking into consideration the individual variations that can be predicted on the basis of a patient’s SNP and CNV genotype. As more polymorphisms are identified and their function understood, this technology will probably become an integral part of clinical practice. In the future, this new technology likely will permit physicians to plan highly individualized therapy for each patient, taking into account issues such as individual disease susceptibility and variations in drug metabolism.
Proteomics
Genes are the main sites of biologic information, but proteins are the main centers of biologic activity, which gives proteins a unique importance. The discipline of proteomics encompasses the study of all the proteins encoded by the genome present in specific tissues, cells, or fluids. Proteomics encompasses not only the study of differences in protein levels but also the study of the modifications that occur after protein synthesis. Study of the proteome is particularly important because levels of mRNA often do not correspond to levels of the protein product.20 In addition, it is estimated that there are over a million proteins encoded by only about 30,000 genes, suggesting that there is substantial protein processing and modification involved in generating the proteome.21 In clinical medicine, proteomic studies often examine differences in proteins between normal and diseased—or between untreated and treated—cells, tissues, or body fluids. Often the goal is to identify biomarkers associated with disease, or to identify novel targets for drug development.
Because the protein complement within a cell can vary widely over time in response to intracellular and extracellular influences, any picture of the proteome must consider these influences. Knowing which genes are expressed or suppressed by a given disease state is important but is only part of the picture. After proteins are synthesized, they can be modified in a number of ways that can dramatically alter their function. Such alterations are generally due to activation of a signaling cascade or enzyme pathway. Because activation of these cascades and pathways can occur without the activation of gene expression, gaining a full picture of the functioning of a cell, particularly during differentiation, development of disease, or response to extracellular signals or drugs, will require determination of protein levels, protein modifications, and protein interactions.
Unfortunately, proteins are much more complex than DNA and RNA in a variety of ways. Proteins are composed of 20 amino acids rather than the four nucleotides that constitute DNA and RNA. The three-dimensional structure of proteins, which is critical to their function, usually is much more complicated than the three-dimensional structure of DNA. Finally, after proteins are synthesized, they undergo a variety of modifications (e.g., cleavage, phosphorylation, and glycosylation) termed posttranslational modifications. In contrast, DNA undergoes relatively little modification after synthesis. Proteomic methods are being developed to detect and measure posttranslational modifications.22,23 The complexity of proteins has slowed the development of high-throughput methods for examining large numbers of proteins simultaneously. Nevertheless, great progress has been made in this field and a number of new techniques are being developed to enhance the use of proteomics for studying disease.21 Some of the techniques bear a resemblance to DNA-based microarrays, except that proteins or ligands rather than oligonucleotides are spotted on a slide.24,25 Other approaches, including mass spectrometry-based analysis of proteins, are also showing considerable promise.21,25,26
Metabolomics
Because metabolites are a heterogeneous group of small molecules, many of which are structurally unrelated, this field presents great challenges. Substantial progress is being made in measuring the metabolic state of a cell using the technologies of gas and liquid chromatography coupled to mass spectrometry.27–30 Recently, relatively high-throughput metabolomics approaches have been utilized to identify metabolomic signatures for a number of disease processes including cancer, motor neuron disease, and type 2 diabetes, and have demonstrated that metabolomic signatures are also useful in identifying drug-response phenotypes.27,29 Metabolomics studies in cancer patients have resulted in the use of this technology for tests for diagnosis of breast and prostate cancer that are paid for by insurance providers.29 Future metabolomic studies will likely help elucidate disease processes, identify biomarkers, and identify new drug targets.
Systems Biology
Together, the fields of genomics, proteomics, and metabolomics characterize biologic processes at a level of detail that was almost unimaginable 20 years ago. However, amalgamating these details into a meaningful narrative is one of the greatest challenges facing scientists (the genomic data alone can have more than 30,000 data points per experiment). The ambitious goal of systems biologists is to integrate data from all the “omic” disciplines, identify important components, and assemble the knowledge into a meaningful whole that can be validated (Figure 99-3).31 Integrating information about system structures, system dynamics, the control method, and the design method can lead to a systems-level understanding of an organism.32 Because of the obvious technical challenges in such an undertaking, this field is still relatively new. Nevertheless, a systems biology approach has already produced novel biologic insights regarding the regulation of innate immunity33,34 and protein kinases.33 As expertise in this field continues to grow, there is optimism that it will lead to new insights in such diverse areas as drug discovery,35,36 synthetic transgene control networks,37 and neurologic diseases,38 to name a few.
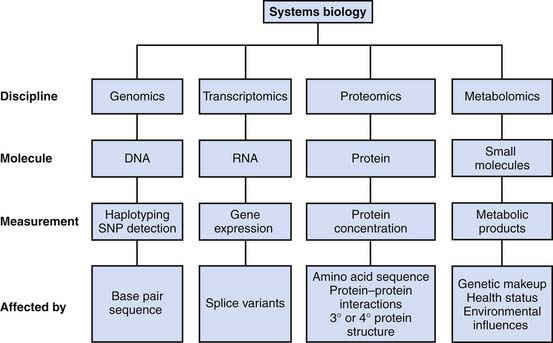
(Modified from Minie ME: Module 7, expression resources, NCBI Advanced Workshop for Bioinformatics Information Specialists. Available at http://www.ncbi.nlm.nih.gov/Class/NAWBIS/.)
Metabolic control analysis is another discipline dedicated to the development of an integrated overview of genetic, enzymatic, and substrate control mechanisms in biologic systems.38,39 When a metabolic control analysis is fully developed, a control coefficient is assigned to each step in an enzymatic pathway.40 These coefficients reflect the magnitude of change that is induced in a pathway compared with the change in the state or level of an enzyme. Enzymes with high coefficients are logical targets for therapeutic intervention (drug design). Identification of important regulatory points also can help with the understanding of carcinogenesis and can provide new insights into genetic disorders.
Clinical Applications
Cancer
Oncologists have made extensive use of gene expression profiling to revise and more accurately classify the prognostic categories of malignancies.41–44 One of the earliest uses of microarray technology for prognostic purposes was to study lymphomas using a specialized microarray, the “Lymphochip.” Using this microarray, investigators were able to identify different histologic classes of lymphoma by their gene expression patterns, indicating that histologically distinguishable tumors differ in their gene expression, so these tumors can be distinguished at a molecular level. More importantly, the investigators found patients with gene expression patterns that permitted them to separate B-cell lymphomas into two groups: one group that resembled germinal center B cells and another that resembled activated B lymphocytes. Although these subgroups had identical histology, patient survival was significantly altered by the gene expression patterns. The lymphomas that exhibited gene expression patterns similar to germinal center B cells had a 5-year survival of 76%, whereas lymphomas that demonstrated gene expression patterns similar to activated B cells had a 5-year survival of 16%.
The promise of expression arrays to help define the prognosis of tumor types has also been used effectively in classifying breast cancers according to risk of metastasis. Use of microarray analysis of gene expression patterns permitted grouping of patients into high- and low-risk groups with greater accuracy than currently used clinical parameters.45,46 Although these investigations were performed with high-density microarrays containing thousands of genes, the investigators found that expression levels of just 70 genes were sufficient to distinguish risk groups.45 The authors believed that these results indicate the propensity to metastasize was an inherent genetic property of certain tumors and that this was not necessarily something that developed late in tumorigenesis. In the near future, these tools may be used to select patients who will require adjuvant therapy and to spare patients in the low-risk group who will not benefit from therapy.
Proteomic and metabolomic studies have also been utilized to characterize cancers. As described earlier (in the Metabolomic section), a specific metabolomic signature has been identified that is now used diagnostically to identify patients with breast and prostate cancer. Proteomic approaches have also been utilized in cancer research. Such studies have generally focused either on better understanding the cancer process with the goal of identifying novel therapeutic targets or on identifying biomarkers that can be used clinically. Proteomics studies have provided significant insight into understanding the mechanism of cancer development and currently much effort is targeting the use of proteomics to identify biomarkers that can be utilized in clinical oncology.47,48

Full access? Get Clinical Tree
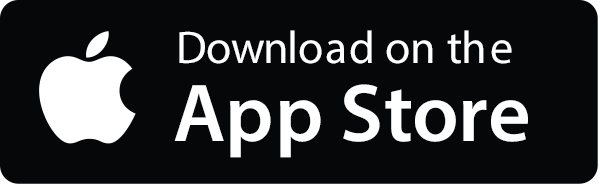
