All the statistical procedures developed so far were designed to help decide whether or not a set of observations is compatible with some hypothesis. These procedures yielded P values to estimate the chance of reporting that a treatment has an effect when it really does not and the power to estimate the chance that the test would detect a treatment effect of some specified size. This decision-making paradigm does not characterize the size of the difference or illuminate results that may not be statistically significant (i.e., not associated with a value of P below .05) but does nevertheless suggest an effect. In addition, since P depends not only on the magnitude of the treatment effect but also the sample size, it is not unusual for experiments with large sample sizes to yield very small values of P (what investigators often call “highly significant” results) when the magnitude of the treatment effect is so small that it is clinically or scientifically unimportant. As Chapter 6 noted, it can be more informative to think not only in terms of the accept—reject approach of statistical hypothesis testing but also to estimate the size of the treatment effect together with some measure of the uncertainty in that estimate.
This approach is not new; we used it in Chapter 2 when we defined the standard error of the mean to quantify the certainty with which we could estimate the population mean from a sample. We observed that since the population of all sample means at least approximately follows a normal distribution, the true (and unobserved) population mean will lie within about 2 standard errors of the mean of the sample mean 95% of the time. We now develop the tools to make this statement more precise and generalize it to apply to other estimation problems, such as the size of the effect a treatment produces. The resulting estimates, called confidence intervals, can also be used to test hypotheses.* This approach yields exactly the same conclusions as the procedures we discussed earlier because it simply represents a different perspective on how to use concepts like the standard error, t, and normal distributions. Confidence intervals are also used to estimate the range of values that include a specified proportion of all members of a population, such as the “normal range” of values for a laboratory test.
then computed its value for the data observed in an experiment. Next, we compared the result with the value tα that defined the most extreme 100α percent of the possible values to t that would occur (in both tails) if the two samples were drawn from a single population. If the observed value of t exceeded tα (given in Table 4-1), we reported a “statistically significant” difference, with P < α As Figure 4-4 showed, the distribution of possible values of t has a mean of zero and is symmetric about zero.
On the other hand, if the two samples are drawn from populations with different means, the distribution of values of t associated with all possible experiments involving two samples of a given size is not centered on zero; it does not follow the t distribution. As Figures 6-3 and 6-5 showed, the actual distribution of possible values of t has a nonzero mean that depends on the size of the treatment effect. It is possible to revise the definition of t so that it will be distributed according to the t distribution in Figure 4-4 regardless of whether or not the treatment actually has an effect. This modified definition of t is
Notice that if the hypothesis of no treatment effect is correct, the difference in population means is zero and this definition of t reduces to the one we used before. The equivalent mathematical statement is
In Chapter 4 we computed t from the observations, then compared it with the critical value for a “big” value of t with ν = n1 + n2 − 2 degrees of freedom to obtain a P value. Now, however, we cannot follow this approach since we do not know all the terms on the right side of the equation. Specifically, we do not know the true difference in mean values of the two populations from which the samples were drawn, μ1 − μ2. We can, however, use this equation to estimate the size of the treatment effect, μ1 − μ2.
Instead of using the equation to determine t, we will select an appropriate value of t and use the equation to estimate μ1 − μ2. The only problem is that of selecting an appropriate value for t.
By definition, 100α percent of all possible values of t are more negative than −tα or more positive than +tα. For example, only 5% of all possible t values will fall outside the interval between −t.05 and +t.05, where t.05 is the critical value of t that defines the most extreme 5% of the t distribution (tabulated in Table 4-1). Therefore, 100(1 − α) percent of all possible values of t fall between −tα and +tα. For example, 95% of all possible values of t will fall between −t.05 and +t.05.
Every different pair of random samples we draw in our experiment will be associated with different values of, and
and 100(1 − α) percent of all possible experiments involving samples of a given size will yield values of t that fall between −tα and +tα. Therefore, for 100(1 − α) percent of all possible experiments
In other words, the actual difference of the means of the two populations from which the samples were drawn will fall within ta standard errors of the difference of the sample means of the observed difference in the sample means. (ta has ν = n1 + n2 − 2 degrees of freedom, just as when we used the t distribution in hypothesis testing). This range is called the 100(1 − α) percent confidence interval for the difference of the means. For example, the 95% confidence interval for the true difference of the population means is
This equation defines the range that will include the true difference in the means for 95% of all possible experiments that involve drawing samples from the two populations under study.
Since this procedure to compute the confidence interval for the difference of two means uses the t distribution, it is subject to the same limitations as the t test. In particular, the samples must be drawn from populations that follow a normal distribution at least approximately.*
* It is also possible to define confidence intervals for differences in means when there are multiple comparisons, by using a Bonferroni or Holm-Sidak correction to determine the appropriate value of t. For a detailed discussion of these computations, see Zar JH. Biostatistical Analysis, 4th ed. Upper Saddle River, NJ: Prentice Hall; 1999.
Figure 6-1 showed the distributions of daily urine production for a population of 200 individuals when they are taking a placebo or a drug that is an effective diuretic. The mean urine production of the entire population when all members are taking the placebo is μpla = 1200 mL/day. The mean urine production for the population when all members are taking the drug is μdr = 1400 mL/day. Therefore, the drug increases urine production by an average of μdr − μpla = 1400 − 1200 = 200 mL/day. An investigator, however, cannot observe every member of the population and must estimate the size of this effect from samples of people observed when they are taking the placebo or the drug. Figure 6-1 shows one pair of such samples, each of 10 individuals. The people who received the placebo had a mean urine output of 1180 mL/day, and the people receiving the drug had a mean urine output of 1400 mL/day. Thus, these two samples suggest that the drug increased urine production by = 1400 − 1180 = 220 mL/day. The random variation associated with the sampling procedure led to a different estimate of the size of the treatment effect from that really present. Simply presenting this single estimate of 220 mL/day increase in urine output ignores the fact that there is some uncertainty in the estimates of the true mean urine output in the two populations, so there will be some uncertainty in the estimate of the true difference in urine output. We now use the confidence interval to present an alternative description of how large a change in urine output accompanies the drug. This interval describes the average change seen in the people included in the experiment and also reflects the uncertainty introduced by the random sampling process.
To estimate the standard error of the difference of the means we first compute a pooled estimate of the population variance. The standard deviations of observed urine production were 245 and 144 mL/day for people taking the drug and the placebo, respectively. Both samples included 10 people; therefore,
Now we are ready to compute the 95% confidence interval for the mean change in urine production that accompanies use of the drug
Thus, on the basis of this particular experiment, we can be 95% confident that the drug increases average urine production somewhere between 31 and 409 mL/day. The range of values from 31 to 409 is the 95% confidence interval corresponding to this experiment. As Figure 7-1A shows, this interval includes the actual change in mean urine production, μdr − μpla, 200 mL/day.
Figure 7-1.

(A) The 95% confidence interval for the change in urine production produced by the drug using the random samples shown in Figure 6-1. The interval contains the true change in urine production, 200 mL/day (indicated by the dashed line). Since the interval does not include zero (indicated by the solid line), we can conclude that the drug increases urine output (P < .05). (B) The 95% confidence interval for change in urine production computed for the random samples shown in Figure 6-2. The interval includes the actual change in urine production (200 mL/day), but it also includes zero, so that it is not possible to reject the hypothesis of no drug effect (at the 5% level). (C) The 95% confidence intervals for 48 more sets of random samples, for example, experiments, drawn from the two populations in Figure 6-1A. All but 3 of the 50 intervals shown in this figure include the actual change in urine production; 5% of all possible 95% confidence intervals will not include the 200 mL/day. Of the 50 confidence intervals, 22 include zero, meaning that the data do not permit rejecting the hypothesis of no difference at the 5% level. In these cases, we would make a Type II error. Since 44% of all possible 95% confidence intervals include zero, the probability of detecting a change in urine production is 1 − β = .56.
Of course, there is nothing special about the two samples of 10 people each selected in the study we just analyzed. Just as the values of the sample mean and standard deviation vary with the specific random sample of people we happen to draw, so will the confidence interval we compute from the resulting observations. (This should not be surprising, since the confidence interval is computed from the sample means and standard deviations.) The confidence interval we just computed corresponds to the specific random sample of individuals shown in Figure 6-1. Had we selected a different random sample of people, say those in Figure 6-2, we would have obtained a different 95% confidence interval for the size of the treatment effect.
The individuals selected at random for the experiment in Figure 6-2 show a mean urine production of 1216 mL/day for the people taking the placebo and 1368 mL/day for the people taking the drug. The standard deviations of the two samples are 97 and 263 mL/day, respectively. In these two samples the drug increased average urine production by = 1368 − 1216 = 152 mL/day. The pooled estimate of the population variance is
in which case,
So the 95% confidence interval for the mean change in urine production associated with the sample shown in Figure 6-2 is
This interval, while different from the first one we computed, also includes the actual mean increase in urine production, 200 mL/day (Fig. 7-1B). Had we drawn this sample rather than the one in Figure 6-1, we would have been 95% confident that the drug increased average urine production somewhere between −34 and 338 mL/day. (Note that this interval includes negative values, indicating that the data do not permit us to exclude the possibility that the drug decreased as well as increased average urine production. This observation is the basis for using confidence intervals to test hypotheses later in this chapter.) In sum, the specific 95% confidence interval we obtain depends on the specific random sample we happen to select for observation.
So far, we have seen two such intervals that could arise from random sampling of the populations in Figure 6-1; there are more than 1027 possible samples of 10 people each, so there are more than 1027 possible 95% confidence intervals. Figure 7-1C shows 48 more of them, computed by selecting two samples of 10 people each from the populations of placebo and drug takers. Of the 50 intervals shown in Figure 7-1, all but 3 (about 5%) include the value of 200 mL/day, the actual change in average urine production associated with the drug.
We are now ready to attach a precise meaning to the term 95% confident. The specific 95% confidence interval associated with a given set of data will or will not actually include the true size of the treatment effect, but in the long run 95% of all possible 95% confidence intervals will include the true difference of mean values associated with the treatment. As such, it describes not only the size of the effect but quantifies the certainty with which one can estimate the size of the treatment effect.
The size of the interval depends on the level of confidence you want to have that it will actually include the true treatment effect. Since tα increases as α decreases, requiring a greater and greater fraction of all possible confidence intervals to cover the true effect will make the intervals larger. To see this, let us compute the 90%, 95%, and 99% confidence intervals associated with the data in Figure 6-1, where the observed mean difference in urine production was 220 mL/day. To do so, we need only substitute the values of t.10 and t.01 corresponding to ν = 18 from Table 4-1 for tα in the formula derived above. (We have already solved the problem for t.05.)
For the 90% confidence interval, t.10 = 1.734, so the interval associated with the samples in Figure 6-1 is
which, as Figure 7-2 shows, is narrower than the 95% interval. Does this mean the data now magically yield a more precise estimate of the treatment effect? No. If you are willing to accept the risk that 10% of all possible confidence intervals will not include the true change in mean values, you can get by with a narrower interval.
Figure 7-2.

Increasing the level of confidence you wish to have that a confidence interval includes the true treatment effect makes the interval wider. All the confidence intervals in this figure were computed from the two random samples shown in Figure 6-1. The 90% confidence interval is narrower than the 95% confidence interval, and the 99% confidence interval is wider. The actual change in urine production, 200 mL/day, is indicated with the dashed line.
On the other hand, if you want to specify an interval selected from a population of confidence intervals, 99% of which include the true change in population means, you compute the confidence interval with t.01 = 2.878. The 99% confidence interval associated with the samples in Figure 6-1 is
In sum, the confidence interval gives a range that is computed in the hope that it will include the parameter of interest (in this case the difference of two population means). The confidence level associated with the interval (say 95%, 90%, or 99%) gives the percentage of all such possible intervals that will actually include the true value of the parameter. A particular interval will or will not include the true value of the parameter. Unfortunately, you can never know whether or not that interval does. All you can say is that the chances of selecting an interval that does not include the true value is small (say 5%, 10%, or 1%). The more confidence you wish to have that the interval will cover the true value, the wider the interval.
As already noted, confidence intervals can provide another way to test statistical hypotheses. This fact should not be surprising because we use all the same ingredients, the difference of the sample means, the standard error of the difference of sample means, and the value of t that corresponds to the biggest α fraction of the possible values defined by the t distribution with ν degrees of freedom.
Given a confidence interval one cannot say where within the interval the true difference in population means lies. If the confidence interval contains zero the evidence represented by the experimental observations is not sufficient to rule out the possibility that μ1 − μ2 = 0, that is, that μ1 = μ2, the hypothesis that the t test tests. Hence, we have the following rule:
If the 100 (1 − α) percent confidence interval associated with a set of data includes zero, there is not sufficient evidence to reject the hypothesis of no effect with P < α. f the confidence interval does not include zero, there is sufficient evidence to reject the hypothesis of no effect with P < α.
Apply this rule to the two examples just discussed. The 95% confidence interval in Figure 7-1A does not include zero, so we can report that the drug produced a statistically significant change in urine production (P <.05), just as we did using the t test. The 95% confidence interval in Figure 7-1B includes zero, so the random sample (shown in Fig. 6-2) used to compute it does not provide sufficient evidence to reject the hypothesis that the drug has no effect. This, too, is the same conclusion we reached before.

Full access? Get Clinical Tree
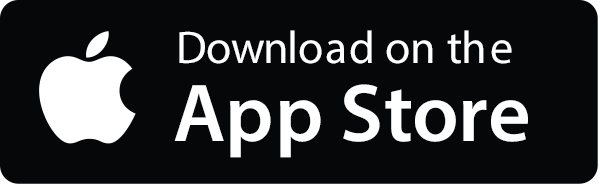
