Abstract
Shared decision-making involves using the best scientific evidence available to make a choice together with the patient or their surrogate, while weighing the options against the patient’s values, preferences, and goals. A lion’s share of the process, therefore, must be devoted to conveying the clinical information and the science surrounding the available options to the patient, or their surrogate, as is often the case in critical care. There are multiple pitfalls to be aware of when conveying numerical risk to patients and families that can impede their decision-making ability. First, numeracy — an aspect of literacy that deals specifically with understanding numbers — can be very poor, even among highly educated people, creating a barrier to comprehending clinical information. Second, the manner in which numerical data are presented can easily manipulate the cognitive biases of patients and their surrogates, limiting their autonomy. Third, clinicians may often present their interpretation of numerical risk rather than the actual data, corrupting the step in shared decision-making where we elicit values and preferences from the patient, imprinting their own values instead. In this Chapter, we explore these issues and discuss ways to optimize the presentation of numerical information.
Shared decision-making involves using the best scientific evidence available to make a choice together with the patient or their surrogate, while weighing the options against the patient’s values, preferences, and goals. A lion’s share of the process, therefore, must be devoted to conveying the clinical information and the science surrounding the available options to the patient, or their surrogate, as is often the case in critical care. There are multiple pitfalls to be aware of when conveying numerical risk to patients and families that can impede their decision-making ability. First, numeracy — an aspect of literacy that deals specifically with understanding numbers — can be very poor, even among highly educated people, creating a barrier to comprehending clinical information. Second, the manner in which numerical data are presented can easily manipulate the cognitive biases of patients and their surrogates, limiting their autonomy. Third, clinicians may often present their interpretation of numerical risk rather than the actual data, corrupting the step in shared decision-making where we elicit values and preferences from the patient, imprinting their own values instead. In this Chapter, we explore these issues and discuss ways to optimize the presentation of numerical information.
3.1 Understanding the Difficulty of Numeracy
At the heart of many critical care conversations is information sharing and prognostication – patients and families want to know what to expect as a serious illness takes its course. Numerical risk ends up being a large part of these discussions (i.e., “What are the exact chances my loved one is ever going to walk again?”). However, if patients and families do not understand the information being presented, it would be suboptimal to have it used as the basis of their supposedly “informed” decisions.
Leiter et al.1 uncovered in their study of numeracy in the intensive care setting that almost one-half of participants had low numeracy and had difficulty with basic questions with numbers (e.g. “Which confers the greatest risk, 1%, 5%, or 10%?”). Many of these participants were highly educated, with 70% of those considered low numeracy surrogates having completed at least some college. Numerous cases given were exactly the type of statements that clinicians present to surrogates and patients every day; for example, “A 50-year-old woman presents with an intracerebral hemorrhage score of 2, which predicts a 74% chance of survival. What do you think the chances are that this patient will survive?” with participants being asked to complete a fill-in-the-blank of the percent likelihood, from 0% to 99%. Almost 50% of participants answered incorrectly. Numerical risk is, therefore, extremely difficult to communicate effectively, recognizing that a large portion of people do not fully understand its true implications.
Adding to this challenge, people do not necessarily have insight regarding their own numeracy skills, although these skills factor heavily into how we make decisions. Even though many people have low numeracy, they nevertheless often prefer to receive numerical rather than qualitative information, often placing more trust in physicians who present numbers rather than verbal descriptions. Higher levels of trust do correlate with decision-makers with higher levels of numeracy.2
Although patients with greater numeracy are in general more likely to actually use numbers in making their decisions, those with lesser numeracy are also more likely to use other factors, such as emotions and trust in the healthcare system.3 Patients and surrogates may have a lack of insight into this tendency, a fact that may also contribute to communication issues in the clinical setting.
Finally, providers themselves can have a difficult time with interpreting and using numbers in their clinical practice and their conversations with patients and families.4 There is a great deal of uncertainty in medicine, and extrapolating choices for a single patient based on statistics — even assuming the best quality evidence, i.e., multicenter double-blind randomized control trial, is a challenging task. When the evidence provides clear guidelines, clinicians still have to use their own judgment to make treatment decisions, understanding that statistics cannot necessarily be applied to individual events or patients.
3.2 Cognitive Biases
Aside from issues of actually understanding the numbers, human cognition is governed in large part by unconscious, rapid, gut reaction judgments, including our cognitive biases (Table 3.1).5 Clinicians can exploit these cognitive biases to guide patients’ and families’ decisions toward outcomes they have deemed to be in the best interest of their patient(s).
Table 3.1. Examples of cognitive biases
Framing is a common way in which biases are used; i.e., the same information is described differently and influences people to make one choice or another. Patients and families may choose different treatment options if the prognosis is described as having a 90% survival rate rather than as having a 10% mortality rate, even though these statements are equivalent. Peters et al.3 found that people with lower numeracy skills were actually more susceptible to framing effects. Indeed, stating a risk as a percentage versus a frequency can have implications on the perceived risk. Moreover, using qualitative statements such as a “high probability of death” can lead to a wider range of interpretations.6
Cognitive biases are an important driver of human behavior, and it is vitally important for clinicians to be aware of them when discussing these important life-and-death matters with their patients and families.
When presenting numbers and data to patients and families, one must keep in mind that what they may consider a “good” outcome may be very different than what the clinician, or even the medical community, views as an acceptable outcome. The modified Rankin Scale (mRS), for example, is a widely used functional outcome measure that describes the level of neurologic disability that patients experience (see Chapter 11, Decompressive Craniectomy for Stroke Patients). However, often when talking about “good” outcomes, the scores 0–3, ranging from no disability to moderate disability (i.e., requiring some help but able to walk without assistance) are used; and when talking about “poor” outcomes, scores of 4–6, ranging from moderately severe disability (i.e., unable to walk without assistance and unable to attend to bodily needs without assistance, to death) are used. Although death, an mRS of 6, is obviously a poor outcome, it is much less obvious that an mRS of 4 might also be, given that many patients who recover from devastating illnesses go on to require a cane or walker, and may need help with some of their activities of daily living. Many people may consider themselves very fortunate to survive a devastating stroke or traumatic brain injury with an mRS of 4, and to present convincing data that frames this outcome as poor may not be fair to decision makers. As such, it is important to note that much of the evidence-based data available is defined using similar scales with relatively arbitrary values delineating “good” from “bad.”
Even in cases where a priori statements indicate that patients would not want to live with any type of disability, many post-illness disabled survivors consider themselves to have an excellent quality of life – a situation known as the “disability paradox.” Although there are many reasons one might question this paradox’s validity — including simply that the most severely disabled patients are unlikely to be able to report their quality of life, which may in fact be very poor — there are an equal number of real-world examples demonstrating its truth when we look.7, 8

Full access? Get Clinical Tree
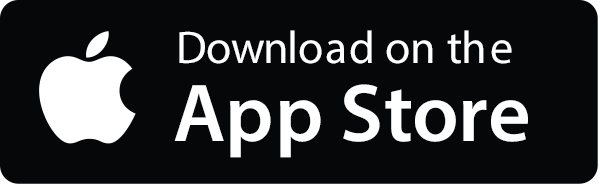
