Despite significant advancements in surgical and perioperative technology, cardiac surgery remains associated with significant risk of morbidity and mortality. Over the last 30 years, at least 20 risk models have been developed to account for variations in patient comorbidities, operations subtypes, and statistical techniques. The utility of risk models is intimately related to the characteristics of the population used to generate them. Therefore, currently accepted and prominent risk calculators based on data from the 1990s and early 2000s require further attention to ensure accurately informed patient and provider decision-making. Furthermore, risk assessment in cardiac surgery has evolved from a primary focus on mortality to other measures of perioperative morbidity, approaching the currently unattainable standard of assessment quality-of-life measures and patient satisfaction with high-risk surgical interventions.
Purpose of Risk Assessment Before Cardiac Surgery
In 1986, publication of poorly adjusted institutional mortality data for coronary bypass surgery by the federal government spurred the creation of the Society of Thoracic Surgeons (STS) database to create a fair and unbiased registry for public reporting. Risk modeling has become an integral instrument in the current health-care environment driven by value-based care. Furthermore, risk adjustment allows cardiac surgery programs, hospitals, and regions to be benchmarked in mortality and morbidity performance reflecting variations in the patient population better than unadjusted comparisons. Several studies have shown that dissemination of risk-adjusted outcomes has resulted in improved mortality and morbidity within a health-care system. Appropriate risk adjustment has become increasingly emphasized, given pay-for-performance reimbursement programs. Finally, appropriate patient counseling is reliant on the integrity of preoperative risk modeling. Nonetheless, risk calculation mandates the recognition of strengths and limitations of a designated model in order to prevent misinterpretation of derived endpoints.
Outcome Measurement in Cardiac Surgery
With major improvements in myocardial protection and rapid dissemination of cardiac surgery in the late 1970s, institutional, regional, and national databases facilitated outcome reporting and risk prediction. Though some efforts were motivated in response to government reports thought to inaccurately depict operative outcomes, the majority of efforts were scholarly and voluntary. Although many efforts used administrative data, prospectively collected clinical data gathered by objective clinicians has become the mainstay of national databases and quality outcome initiatives.
Development of Perioperative Risk Assessment for Cardiac Surgery
Regardless of collection method, accuracy of data elements is critical to the validity of any prediction model. Moreover, responsible stewardship of statistical methodology and choice of sound statistical techniques enhance the ultimate utility of risk models. Among the various perioperative risk calculators, patient characteristics, operation types (all cardiac surgery, coronary artery bypass grafting [CABG], valve, CABG + valve), institutional structure and location, and statistical methods impact the predictive capability. Inclusion of emergent and urgent operations will further bias the results of risk assessment tools. Models are often forced to lump heterogeneous operations (such as valve repair/replacement) in order to achieve improved discriminatory power of relatively rare events such as death. Regardless of these considerations, risk scores based on retrospective observational data are inherently biased because of the inevitable impact of the surgeon’s selection bias. Furthermore, model development requires balanced inclusion of patient and hospital characteristics.
Features of a risk assessment scale include discrimination and ability for calibration. Discrimination can be defined as a model’s ability to distinguish between patients suffering from a specific adverse event such as mortality and/or major morbidity and those who do not. Most models measure discriminatory power using the C-statistic obtained from the area under the receiver operating characteristic curve (AUC). Calibration of models has also been described as a crucial part of accurate model development. Without calibration, a risk calculator cannot be expected to provide accurate predictions of patient risk. Historic techniques for assessing model calibration have included the Hosmer-Lemeshow goodness of fit. More recently, many have proposed replacement of Hosmer-Lemeshow model calibration with risk-adjusted mortality using observed/predicted ratios. Bhatti and colleagues have also suggested performing chi-square tests to compare the observed to expected mortality as a means to better fit the model to actual data. While availability of a validation cohort to perform model discrimination and calibration is critical, several currently available risk assessment scores, including the European System for Cardiac Operative Risk Evaluation (EuroSCORE), were calibrated using only the derivation dataset.
Currently, most risk algorithms are based on logistic regression analysis with a priori assumptions of linear relationships. Current risk prediction can be improved by using complex techniques such as an artificial neural network, which has the advantage of the capacity to model complex, nonlinear relationships and is relatively robust and tolerant of missing data.
Established Risk Assessment Models in Cardiac Surgery
Over the last 30 years, over 20 cardiac surgery risk stratification models have been devised ( Table 6.1 ). The characteristics included vary for each unique patient population; the most commonly used models are compared in Table 6.2 . Discussed in more detail in this section are the Parsonnet; EuroSCORE; age, creatinine, and ejection fraction (ACEF); and STS mortality and morbidity scores.
Model | Region | Years of data collection | Year of publication | Number of patients (centers) | Risk variables |
---|---|---|---|---|---|
Amphiascore | The Netherlands | 1997–2001 | 2003 | 7282 (1) | 8 |
Cabdeal | Finland | 1990–1991 | 1996 | 386 (1) | 7 |
Cleveland Clinic | USA | 1986–1988 | 1992 | 5051 (1) | 13 |
EuroSCORE (additive) | Europe | 1995 | 1999 | 13,302 (128) | 17 |
EuroSCORE (logistic) | Europe | 1995 | 1999 | 13,302 (128) | 17 |
French score | France | 1993 | 1995 | 7181 (42) | 13 |
Magovern | USA | 1991–1992 | 1996 | 1567 (1) | 18 |
NYS | USA | 1998 | 2001 | 18,814 (33) | 14 |
NNE | USA | 1996–1998 | 1999 | 7290 (N/A) | 8 |
Ontario | Canada | 1991–1993 | 1995 | 6213 (9) | 6 |
Parsonnet | USA | 1982–1987 | 1989 | 3500 (1) | 16 |
Parsonnet (modified) | France | 1992–1993 | 1997 | 6649 (42) | 41 |
Pons | Spain | 1994 | 1997 | 1309 (7) | 11 |
STS risk calculator a
| USA | 2002–2006 | 2007 | 774,881 (819) 109,759 101,661 | 49 50 50 |
Toronto | Canada | 1993–1996 | 1999 | 7491 (2) | 9 |
Toronto (modified) | Canada | 1996–1997 | 2000 | 1904 (1) | 9 |
Tremblay | Canada | 1989–1990 | 1993 | 2029 (1) | 8 |
Tuman | USA | N/A | 1992 | 3156 (1) | 10 |
UK national score | UK | 1995–1996 | 1998 | 1774 (2) | 19 |
Veterans Affairs | USA | 1987–1990 | 1993 | 12,715 (43) | 10 |
a The STS risk calculator of seven risk prediction models in three main categories, namely isolated CABG, valve procedures, and combined CABG and valve procedures. Data represented for the STS risk calculator reflect the number of patients and risk variable captured in the database used for the latest models developed (version 2.61).
Preoperative risk factor | EuroSCORE | STS | Initial parsonnet | Cleveland clinic | NNE (CABG only) | Complex bayes (CABG only) |
---|---|---|---|---|---|---|
Age | X | X | X | X | X | X |
Sex | X | X | X | X | ||
Race | X | |||||
Weight/BSA | X | X | X | X | X | |
IABP/inotropes | X | X | X | |||
LV function | X | X | X | X | X | X |
Renal disease | X | X | X | X | X | X |
Lung disease | X | X | X | X | X | |
PVD | X | X | X | X | ||
Diabetes | X | X | X | X | X | |
Neurologic dysfunction | X | X | X | X | ||
Active endocarditis | X | |||||
Unstable angina or recent MI | X | X | X | |||
Previous cardiac surgery | X | X | X | X | X | X |
Combined surgery | X | X | X | NA | NA | |
Aortic involvement | X | X | NA | NA | ||
Valve surgery | X | X | X | X | NA | NA |
Emergency surgery | X | X | X | X | X | X |
Parsonnet
In 1989, Victor Parsonnet popularized the first risk score in cardiac surgery mortality prognostication, based on 14 variables derived from a single institution. While the model has been criticized because of advancement of surgical techniques compared with the original era in which the score was devised, the scoring methodology holds reasonable discriminatory power nearly 30 years later. Some of the criticism is associated with inclusion of too few variables and certain variables that are thought to be arbitrary and could significantly influence the calculated risk (e.g., catastrophic states and other rare circumstances). The Parsonnet score divides patients into five risk groups based on the score accumulated from 16 different preoperative variables. Later iterations of this scale, namely the 2000 Bernstein-Parsonnet logistic regression–based additive risk model, have been shown to be comparable to the EuroSCORE in prediction of mortality. Despite this modification, the Parsonnet score is still not favored, given that it is based on data that are very old and predate many advances in state-of-the-art cardiac surgery.
E uro SCORE
The EuroSCORE was developed in the late 1990s in response to the need for an objective scoring system representative of a diverse population of cardiac surgery patients. In contrast to earlier scoring systems such as the Parsonnet score and the Cleveland Clinic Risk Score, which were developed on the basis of individual institutional experience, the EuroSCORE was derived from and validated on the basis of 20,014 consecutive patients from 132 hospitals in eight European countries. The score was developed using 97 risk factors to generate the EuroSCORE calculation, which can be calculated by the additive method or more complex, logistic model. The original cohort had a mean age of 62.5 years, with 10% of patients being older than 75 years of age and only 28% being female. The derivation cohort featured 0.5% dialysis dependence; 13.7% had chronic cardiac insufficiency, while only 1% of cases were reported as emergent. The additive method has been found to overestimate the chance of mortality for patients at the lower end of the risk spectrum while underestimating this parameter in the very high-risk patient groups. Thus mortality estimates transition from over to under at the 12% margin pivot point. Further limitations of the EuroSCORE include lower and upper limits of risk (0 and 22%) as well as reported maximal sensitivity of 64% and specificity of 87%. In a study by Nashef and colleagues, the EuroSCORE was validated in the North American population using STS data from 1995 to 1999. A comparison of the two scales found that the EuroSCORE had satisfactory discriminatory power in various subsets of patients across several years of data despite differences in baseline demographics, risk levels, and surgical characteristics between the European and American cohorts.
ACEF
Creation of the ACEF score was motivated by the fact that internationally used risk calculators were based on large populations for low event rates, including emergent and nonemergent cases. If these published risk calculators were applied to centers with low annual operative volume and special patient populations, they may no longer be valid, given limited power for most of the covariates. Thus Ranucci and colleagues attempted to evaluate the discriminatory power of a parsimonious mortality calculator with only three factors. The ACEF score was based on 8648 patients undergoing elective operations and calculated by dividing patient age by left ventricular ejection fraction and adding 1 if serum preoperative creatinine was >2.0 mg/dL. Patients’ predicted mortality was calculated on the basis of the nomogram shown in Fig. 6.1 . Compared with the EuroSCORE and several regional risk scores, ACEF performed best in predicting mortality after an isolated CABG and only second to the Cleveland Clinic Score overall.
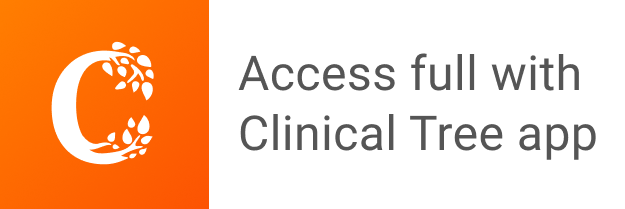